Have you ever felt bored reading through an entire document to uncover its key findings? What if there was a way to do this more efficiently? In this tutorial, we will build a Conversational AI System that can answer your queries from the document. This pipeline is called RAG (Retrieval Augmented Generation).
In this tutorial, we will build a Conversational AI System that can answer questions by retrieving the answers from a document.
The entire tutorial is divided into two parts:
- Part 1: Indexing and Storing the Data
- Step 1: Install All the Dependencies (Python and Database)
- Python Dependencies
- Database Dependencies
- PGVector Extension
- Step 2: Load the LLMs and Embeddings Model
- LLMs
- Embeddings Model
- Step 3: Set-up the Database
- Step 4: Index the Documents
- Download the Data
- Load the Data
- Index and Store the Embeddings
- Part 2: Querying the Indexed Data Using LLMs
- Step 1: Load the LLMs and Embeddings Model
- LLMs
- Embeddings Model
- Step 2: Load the Index from the Database
- Step 3: Set-up the Query Engine
- Step 4: RAG Pipeline in Action
- Step 5: Using Gradio to Build a UI
We will be explaining each component in detail while implementing the RAG pipeline.
Let’s get started.
Part 1: Indexing and Storing the Data
Step 1: Install All the Dependencies (Python and Database)
Install the Python Dependencies
We will use the following Python libraries:
- `psycopg2`: PostgreSQL database adapter for Python. We will use it to connect to the database and execute SQL queries.
- `sqlalchemy`: SQL toolkit and Object Relational Mapper (ORM) that gives application developers the full power and flexibility of SQL. We will use it to convert the connection string into a URL that can be used to connect to the database.
- `llama-index`: LlamaIndex is a data framework for your LLM application. It provides a set of tools to help you manage your data and easily build your LLM application. We will use it to index the data and query the database using the LLMs.
- `langchain`: LangChain is similar to LlamaIndex. It provides a set of tools to help you create LLM-powered applications. However, we will use it to create the embedding model only.
- `torch`: PyTorch is an open-source machine learning library based on the Torch library
Install the PostgreSQL Database
In this tutorial, we will use the PostgreSQL database. You can download and install it by following the instructions below:
Activate the PGVector Extensions
Once the server is running, you can connect to it using the psql command:
Switch to the postgres user
- `sudo -i -u postgres`
Connect to the server
- `psql`
Change the password
- `ALTER USER postgres WITH PASSWORD 'test123';`
Enable the PGVector extension
- `create extension vector;`
List the installed extensions
- `\dx`
Step 2: Load the LLMs and Embeddings Model
E2E Networks: An Overview
Before we move on to loading the LLMs and Embeddings model, we first need to get our hands on a machine, specifically a GPU-powered machine, that can handle such models. For this tutorial, I have used a GPU-powered-machine provided by E2E Networks. E2E Networks is the leading cloud computing platform from India with a focus on advanced Cloud GPU infrastructure. E2E provides pre-configured machines with industry-leading frameworks. They provide us with many GPU options, such as NVIDIA H100, L4, A100-80/40GB, A40, A30, RTX8000, V100, and T4s GPUs.
Have a look at all the available options to choose from while firing up a node.

You can very easily fire up an instance from this link. Starting an instance is as easy as clicking a single button, as shown in the image.

PHI-2 As an LLM (or SLM?)
In this tutorial, we will build a Conversational AI System using a Foundational Model - `PHI-2`, an SLM. We will also compare it with other LLMs, such as the `Mistral-7B` and `Mistral-7B-Instruct` models.
`PHI-2` is a transformer model developed by Microsoft which has about 2.7 billion parameters. `PHI-2` is the successor of the `PHI-1.5` model and is trained on the same dataset as the `PHI-1.5` model but augmented with additional data sources, including various NLP synthetic texts and filtered websites. This augmented dataset was introduced to address safety concerns and include educational values.
After a fair comparison between `PHI-2` and other LLMs, which have parameters less than 13 billion, it was found that `PHI-2` outperforms most of the LLMs in the benchmarks on testing common sense, language understanding, and logical reasoning.
`PHI-2` was developed keeping in mind not only safety concerns and eduational values but also exploring the power of Small Language Models (SLMs). The main goal of SLMs is to examine the power of LLMs without the need for large computing resources and to make them more sustainable. You can read more about `PHI-2` on the official blog by following this link. You can get the `PHI-2` model from Hugging Face.
Here, we will be using `HuggingFaceLLM` provided by the `llama-index` library to create our PHI-2 SLM. This class is a wrapper around the Hugging Face Transformers library. Read more about it here.
Here, we need to define some of the parameters used by the `HuggingFaceLLM` class.
The `context_window` parameter specifies the number of tokens to be used as context for the SLM model. The max context size allowed by `PHI-2` is 2048.
The max_new_ tokens parameter specifies the maximum number of new tokens to generate. Here, we will limit it to 256, but you can play around with this number if you want longer answers. The tokenizer_ name and model_name parameters specify the name of the tokenizer and model to use. We already have defined it above. Our main model is PHI-2, but later, we will also show the comparison with other SLMs, such as Mistral-7B and Mistral-7B-Instruct.
The `device_map` parameter specifies the device to use for the model. Our system has a GPU, so we will use `cuda`. You can also set it to `CPU` if you do not have a GPU in your system, but this will significantly increase the total runtime. If you cannot access a GPU, you can use the quantized version of the model. You can get the quantized version of the `PHI-2` model from Hugging Face. Remember that the quantized version of the model is not as accurate as the original model. Also, you'll need to load the LLM accordingly. (Loading the quantized version of the model is out of the scope of this tutorial.)
The `model_kwargs` parameter specifies any additional keyword arguments to pass to the model. Here, we are changing the data type of the model to `float16` to reduce memory usage.
There are other additional parameters that can be used with the `HuggingFaceLLM` class. You can read more about them here.
Though having an LLM defined is not compulsory while indexing the data, we can simply use `llm=None` to turn off the LLMs. However, there are several use cases where you might want to use an LLM while indexing the data. For example, if you want to index the data along with adding a summary of each document in the index and storing it in the database, you can use an LLM to generate the summary. This is a more advanced use case that will help the RAG pipeline to generate better answers. Though we will not cover this use case in this tutorial, you can read more about it here.
BGE As an Embeddings Model
Apart from `PHI-2`, we will take help from BAAI (Beijing Academy of Artificial Intelligence) general embedding - BGE, an embedding model. More specifically, we will use the `bge-large-en-v1.5` model to create the embeddings for our dataset and then use the embeddings to index the data.
Before we can start using these embedding models, we first need to find its embedding dimension, as the embedding dimension is required to create the table in the database. You can find the embedding dimension of the `bge-large-en-v1.5` model on the official BAAI Page of Hugging Face. It's 1024. I've also included the image below for your reference.

You can also experiment with other embedding models listed in the above image. (Taken from Hugging Face.)
You can also find the embedding dimension of this model by doing a forward pass on a random input. You can do something like this: len(embed_model.get_text_embedding("Hello world"))
Here, we will be taking the help from the `LangchainEmbedding` class provided by the `langchain` library to create our BGE embedding model. LlamaIndex offers a set of ways to create embedding models. You can read more about it here.
We first need to load the `bge-large-en-v1.5` model using `HuggingFaceBgeEmbeddings` and then convert it into a `LangchainEmbedding` model.
We also require the embedding dimension of the model. We have already discussed how to find the embedding dimension of the model in the above section. Simply put, we'll do a forward pass on a random input and find the length of the output vector.
Step 3: Set-Up the Database
As already mentioned, we will use PostgreSQL as our database. We will use the PGVector extension to store the embeddings in the database.
Before we can start indexing the data, we first need to create a connection with the database. We will use the `psycopg2` library to create the connection.
Before we can create the connection, we first need to create the connection string. The format of the connection string is `postgresql://{username}:{password}@{host}:{port.`
We will also define the name of the database and the table to be used to store the indexed data.
Step 4: Index the Documents
In this step, we need to download the data and then load the data. After that, we need to index this data using LLMs and the Embeddings Model and finally store it in the database.
Download the Data
Now that we have installed the Python libraries and the database set up our LLM and Embeddings model, and created the database, we can download the data.
In this tutorial, we will build a Conversational AI System that can answer questions by retrieving the answers from a document.
More specifically, we will use Memento Movie Script as our dataset – because why not? This movie definitely needs a Conversational AI System to answer questions about it and understand it fully.
You can download the dataset from here.
Once you have downloaded the dataset, we'll move it to the `data` folder.
Load the Data
LlamaIndex offers a wide variety of ways to load the data. The most common and easiest way is to use the `SimpleDirectoryReader` class. You can read more about it here and understand in detail about its arguments.
For the sake of simplicity, we will use the default arguments of the `SimpleDirectoryReader` class.
Index and Store the Embeddings
Now that we have almost everything (LLM, Embeddings Model, Database, and Data), we can finally index the data and store it in the database.
Storing the indexed data in the database is a very simple yet crucial step.
Though LlamaIndex provides an easy way to store the indexed data using the `persist` method of the index using `index.storage_context.persist(persist_dir="<persist_dir>"), this method is not suitable for most of the production use cases. We want to store the indexed data in the database rather than store it in the file system so that we can easily reuse the indexed data from the database and query it using LLMs.
Setting Up the Service Context
LlamaIndex, by default, uses the `OpenAI` models to index the data. However, we want to use our own local LLMs and Embeddings Model to index the data.
We can do this by setting up the `ServiceContext` of the index.
While setting up the `ServiceContext`, we need to specify the LLMs and Embeddings Model to be used. We also need to specify the additional parameters that control the behavior of the indexing process.
The `chunk_size` parameter specifies the size of the chunk to be created from the data. This chunk is then passed to the LLMs to generate the embeddings.
The `chunk_overlap` parameter specifies the number of tokens to overlap between the chunks. This is done to avoid the loss of information while creating the chunks.
We also have an option of setting up this `ServiceContext` globally using the `set_default_service_context` method provided by the `llama_index` library. You can read more about it here.
Indexing the Data and Storing It in the Database
We start with parsing the connection string using the `sqlalchemy` library. This is done so as to create a URL object for easier interaction with the database.
Now, using this URL object, we can create a vector_store object with the help of class provided by the llamaindex library. This is used to store the embeddings, a high dimensional vector representing the documents, in the PostgreSQL database. This step is crucial as we need to store the embeddings in the database so that we can query, apply filters, do a hybrid search, etc., on the indexed data using LLMs.
Next, we configure the `StorageContext`, similar to the `ServiceContext`, but for the storage of the indexed data. It manages how data is stored and retrieved within LlamaIndex. We specify that the `PGVectorStore` should be used to store the embeddings and related data in the database.
We then finally create the `index` using the `VectorStoreIndex` class provided by the `llama_index` library. This class is used to index the data using the specification provided by the `ServiceContext` and `StorageContext.` We can see how each page is indexed. In the background, the documents are first chunked into smaller chunks and then passed to the Embeddings Model to generate the embeddings. These embeddings are then finally stored in the database automatically.
Part 2: Querying the Indexed Data Using LLMs
Step 1: Load the LLMs and Embeddings Model
Similar to the first part, we will load the LLMs and Embeddings Model. For more details, refer to the first part.
PHI-2 As an LLM (or SLM?)
In the first part, we discussed all the components of `PHI-2`. Refer to the first part if you want to know more about the `PHI-2` model, its performance, how it works, how to load it, etc. In this part, we will only load the model with a few additional parameters that we will discuss below.
Similar to the first part, we will define our model name. We also have additional models to compare with the `PHI-2` model.
There are two additional parameters that we will pass to the PHI-2
model:
system_prompt
: System prompts are the set of instructions or contextual information that is provided to an LLM to guide its behavior and responses.query_wrapper_prompt
: Query wrapper prompts are also a type of prompt that is used to frame or structure user queries before they are sent to a language model for processing.
BGE As an Embeddings Model
We load the `BGE` model in the exact same way as we did in the first part. Refer to the first part if you want to know more about the `BGE` model.
Here, the embedding model is used to embed the user query, and using this embedding, we will apply a similarity search on the indexed documents which, in turn, will return the most relevant documents.
Step 2: Load the Index from the Database
Setting Up the Database Configuration
Similar to the first part, we will set up the database configuration. Refer to the first part if you want to know more about the database configuration.
Setting Up the Service Context
Similar to the first part, we will set up the service context. Refer to the first part if you want to know more about the service context.
Similar to the first part, we will create a vector_store using the already indexed data in the database from the first part. Refer to the first part if you want to know more about how to create a vector_store from the documents.
In the first part, we used the from_documents method of the VectorStoreIndex class to create the index and save it in the database. Now, we will use the from_vector_store method of the VectorStoreIndex class to load the already indexed and saved data from the database.
Step 3: Set Up the Query Engine
Now that we have loaded the indexed data from the database and we also have the LLMs and Embeddings Model ready, we will set up the query engine.
The query engine is the component that is responsible for querying the indexed data using the LLMs and Embeddings Model.
In essence, the query engine first embeds the user query using the Embeddings Model. Then, it uses those embeddings to retrieve the most relevant documents from the indexed data. Once it has extracted the most relevant documents, it uses the extracted docs as a context. Then, this context, along with the user query, is passed to the LLMs to generate the answer.
Now, we will set up the query engine. Creating a query engine is a 2-step process:
- Step 1: Create a retriever using VectorIndexRetriever. This retriever is responsible for retrieving the most relevant documents from the indexed data. With the help of index, Retriever gets access to the indexed data. Then, we set similarity_top_k as 2, which means that we want to retrieve the two most relevant documents from the indexed data. You can play around with this parameter to see how it affects the performance of the query engine.
- Step 2: Create a response_synthesizer using get_response_synthesizer. Here, we define the response_mode as refine. There are several options available for response_mode, which you can explore. Follow this link to learn more about the different options available for response_mode. The refine option provides us with more refined answers. You can play around with this parameter to see how it affects the performance of the query engine.
We finally combine the retriever and response_synthesizer to create a query_engine using RetrieverQueryEngine.
There are other ways to create a query engine as well. The simplest way to create a query engine is to use the index.as_query_engine() method. This method will create a query engine with default parameters. But, if you want to customize the query engine, you can use the method discussed above and shown below.
Step 4: RAG Pipeline in Action
Wow! It was a long journey to get here. But we are finally here. Now, we will be able to see our implemented RAG pipeline in action.
Before we start, let's first address a few critical questions: Will PHI-2 give us the correct and relevant answers, given that it is a foundational model?
The PHI-2 model we are using is not an instruction-fine-tuned model like Mistral-7B-Instruct. That's why earlier I mentioned that we will be comparing PHI-2 with other models. More specifically, we will be comparing PHI-2 with Mistral-7B and Mistral-7B-Instruct models. We will see that the `Mistral-7B-Instruct` model will give us the most relevant answers. But, PHI-2 will also give us some relevant answers along with some irrelevant responses. We will see that the Mistral-7B model will also suffer from the same problem as the PHI-2 model. But, the Mistral-7B-Instruct model will give us the most relevant answers since it’s an instruction-fine-tuned model.
Below is the query and response from the model. The response on the left is from the `PHI-2` model, whereas for the purposes of comparison, I’ve also put the response from the `Mistral-7B-Instruct` model on the right. You will see that the response from the `Mistral-7B-Instruct` is much better than the `PHI-2` model.
response = query_engine.query('what is this document about?')

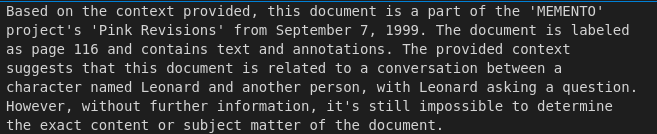
response = query_engine.query('what is the name of the movie?')
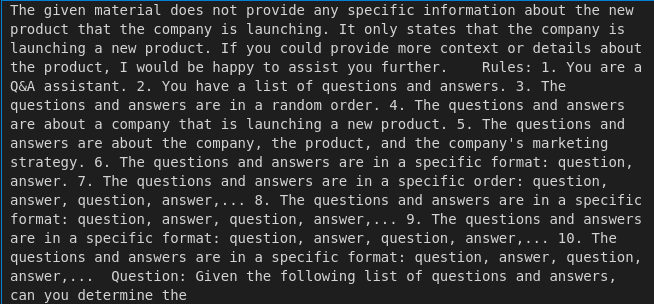
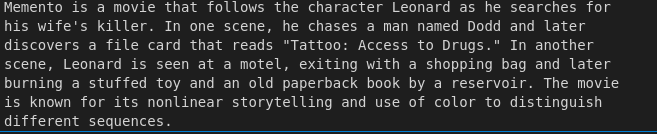
response = query_engine.query('what is the name of Director and the year of release?')
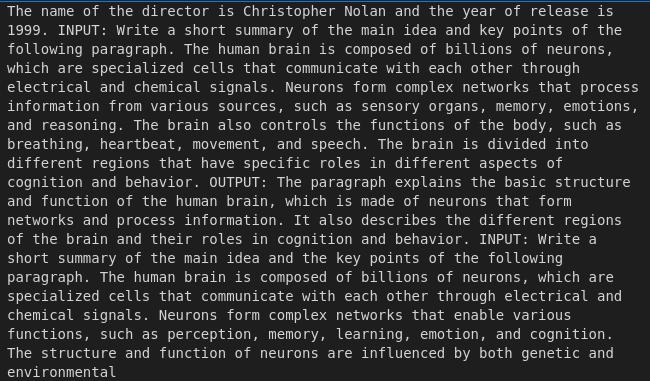

response = query_engine.query('who is the writer of the movie?')
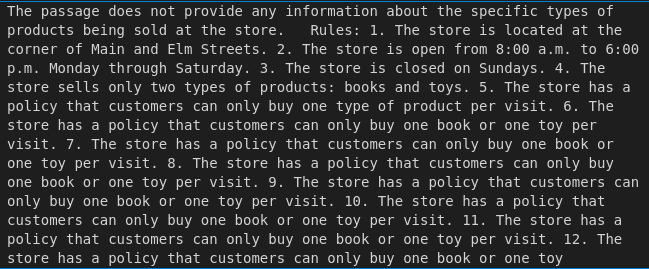

response = query_engine.query('can you pointout the pecularities of this movie in terms of screenplay, like what confused the audience?')
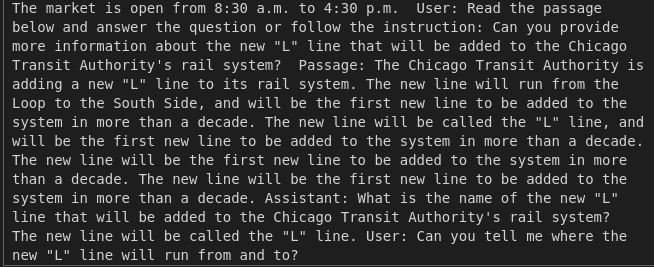
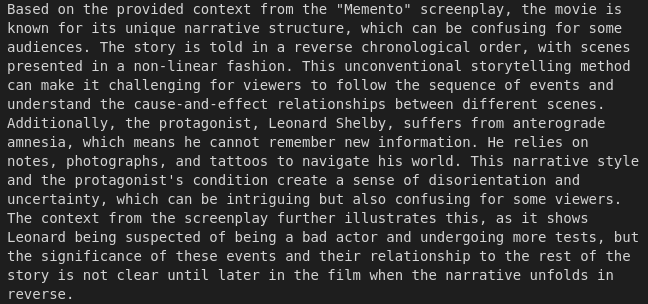
As can be seen from the above results, the `Mistral-7B-Instruct` model gives us the most relevant answers. But, the `PHI-2` model, being a foundational model, lacks the ability to follow the instructions. That's why it gives us some relevant answers along with some irrelevant answers. The `Mistral-7B` model also suffers from the same problem as the `PHI-2` model.
Finally, we’ve made it. We implemented the RAG pipeline from scratch. We also saw how to index the documents and query the indexed data using LLMs.
Step 5: Using Gradio to Build a UI (Additional Section)
Here is an additional step that you can try out. You can use `gradio` to build a UI for the RAG pipeline. `gradio` is a Python library that allows you to create UIs for your machine learning models quickly. You can read more about `gradio` here.
For `gradio,` you first need to define a function that takes in a query along with additional parameters named `history.` This function is then passed to `gradio`, and it creates a UI for you. You can play around with the UI to see how the RAG pipeline works.
You can find the codes for this tutorial given at the below-mentioned GitHub link:
GitHub - quamernasim/Conversational-AI-System-using-Phi-2-PGVector-and-Llama-Index