Introduction
In today’s digital age, the way we consume news has undergone a significant transformation. The persistent wave of information and the abundant clickbait culture pose a considerable challenge for those seeking quick and reliable news. Consumers are constantly bombarded with headlines that vie for attention, often at the expense of factual accuracy. This information overload makes it difficult to filter through the noise and find content that is both trustworthy and relevant. Even few reputed media houses use clickbaits and fake news to attract readers to their site.
NewsGPT (Neotice) is a new open source application created by Tim K.C. Ho, designed to cut through the clutter and deliver news that matters, and was one of the applications that won the Streamlit Hackathon.
Summary of The Technologies Used
NewsGPT uses LlamaIndex, Apache Spark, Firebase and Qdrant Vector DB to not only summarize news articles but also ensure the information is pulled from credible sources. The NewsGPT github repo explains how to deploy and build on it.
Apache Spark
Apache Spark™ is a highly regarded open-source, distributed processing system designed for large-scale data workloads. Spark is recognized for its ability to handle batch and real-time analytics and data processing tasks. It unifies these capabilities within a single framework, making it a go-to solution for a wide range of data engineering, data science, and machine learning applications on both single-node machines and clusters.
Apache Spark includes several components that enhance its utility across various domains:
Spark Core: This is the foundation of the platform, handling memory management, fault recovery, job scheduling, and other fundamental functions.
Spark SQL: A distributed query engine that provides low-latency, interactive queries. It's used for processing structured data with SQL or Hive Query Language, making it suitable for a range of applications from business analytics to data reporting.
Spark Streaming: This component is designed for real-time data processing. It allows the processing of live data streams, making it a robust solution for real-time analytics.
MLlib (Machine Learning Library): Spark includes a library of machine learning algorithms, enabling scalable and efficient data science applications. It supports various tasks like classification, regression, and clustering.
GraphX: A library for graph processing, enabling users to interactively build and transform graph data structures at scale
LlamaIndex
LlamaIndex is a comprehensive data framework specifically designed for enhancing Large Language Model (LLM) applications. It acts as a bridge between custom data sources and LLMs, enabling users to integrate, manage, and query a wide range of data types using natural language.
Key Features of LlamaIndex
Data Ingestion: LlamaIndex supports a variety of data sources, including APIs, PDFs, documents, SQL, and more, allowing users to connect their existing data formats with LLM applications.
Data Indexing: It provides capabilities to store and index data for various use cases. This involves converting data into a format that can be easily queried and understood by LLMs.
Query Interface: One of the standout features is its query interface, which allows for knowledge-augmented responses to input prompts over the indexed data. This enables the generation of responses that are contextually relevant and informed by the underlying data.
How LlamaIndex Works
Ingestion: LlamaIndex can connect custom data sources to LLMs using hundreds of data loaders. It supports a wide range of pre-built solutions like Airtable and Salesforce, as well as generic plugins for loading data from various formats.
Indexing: After ingestion, data is indexed to be mathematically represented, allowing for easy querying by LLMs. Different types of indexing models, such as vector, list, tree, and keyword indexes, cater to specific application needs.
Querying: The querying process in LlamaIndex is intuitive, using natural language through prompt engineering. This simplifies the interaction with data, making it more accessible and user-friendly.
Firebase
Firebase is a comprehensive backend platform, offering a suite of cloud-based tools and services for application development.
Key Features and Services of Firebase
Authentication: Firebase provides a robust authentication system supporting various methods, including email, phone numbers, and social media platforms like Google, Facebook, and Twitter. It also offers FirebaseUI for building familiar sign-in user interfaces.
Real-Time Database and Cloud Firestore: These are NoSQL databases that offer real-time data syncing across client devices. The Real-time Database stores and syncs data in a JSON-like format, whereas Firestore organizes data into collections and documents for more complex, hierarchical data structures.
Hosting: Firebase offers easy-to-configure hosting for websites, supporting static frontends and dynamic content via technologies like HTML, CSS, JavaScript, and Express.js. It provides automatic HTTPS configuration and fast delivery through a global Content Delivery Network.
Cloud Functions: This is a serverless framework allowing developers to write functions in Node.js, which respond to various events triggered within Firebase or via HTTP requests. It's designed for high scalability and reliability.
Cloud Storage: Suitable for storing user-generated content like images and videos, Firebase Storage ensures robust, resilient uploads and downloads.
Cloud Messaging: This service enables the sending of notifications to users to enhance engagement and user retention
Qdrant Vector DB
Qdrant is an open-source vector database and vector search engine, predominantly written in Rust. It is designed to offer fast and scalable vector similarity search services with a user-friendly API. Qdrant facilitates the efficient handling of high-dimensional vectors, making it particularly suitable for applications that involve embeddings or neural network encoders. These capabilities enable Qdrant to support a wide range of use cases, including matching, searching, recommending, and much more in data-intensive applications.
One of Qdrant's strengths lies in its ability to manage embeddings produced by neural network encoders, which are essential for semantic-based matching and various other applications. It supports a range of features such as faceted search, making it versatile for different kinds of data matching and retrieval tasks. The platform is also designed to be easy to set up and use, offering different methods for deployment including Docker images and a Python client, as well as a cloud-based solution for production environments.
Deploying NewsGPT on E2E Cloud
NewsGPT is open source, so you can easily customize and deploy it for your own individual use case. To do so, head to E2E Cloud, sign up, and launch a GPU node.
E2E Cloud has A100 Cloud GPU Server, and has recently also launched HGX 8xH100, the most powerful AI supercomputer currently available, for instant access.
Once you have launched your GPU node, clone the repository, and set up your Python virtual environment, and install the requirements.
The current repo has been setup with the following in the config, which you can modify:
This mentions the news domains, and the number of articles to fetch for the feed.
How It Works
The technological framework of NewsGPT is built upon real-time news aggregation, and then a RAG pipeline that creates embeddings from each of the articles, and allows the LLMs to query and access the information within the articles.
Data Pipeline
The data pipeline of NewsGPT starts with Google News, which acts as a primary source for the latest news articles across various domains. This is followed by asynchronous web scraping, a process designed to collect data efficiently without being bottlenecked by the sequential loading of articles.
The scraped content is then channeled through Kafka, a distributed event streaming platform that handles the flow of data with high throughput and scalability.
From Kafka, topics are organized and funneled into Spark Streaming, a technology adept at processing real-time data streams. It performs two critical functions here: it recognizes named entities within the content, and it generates embeddings. Named Entity Recognition (NER) isolates and categorizes key components from the text, such as individuals, organizations, and locations.
The generation of embeddings translates articles into vectorized forms that capture the semantic essence of the content. These vectors are then stored in the Qdrant Vector DB, which serves as a repository for the processed data, ready for retrieval and analysis.

Personalization and Recommendation
Personalization is at the heart of NewsGPT, where user preferences and activities dictate the content that is recommended. When a user interacts with the platform, their choices and behaviors are captured—what articles they read, what topics they linger on, and what they search for.
This data is funneled into the Qdrant Vector Database, where it informs the recommendation algorithms. The database acts as a matchmaker, aligning the user's interests with articles from a vast pool of content. The result is a tailored news feed that feels personal because it is shaped by the individual's own reading patterns and preferences.
Chat with Articles
The Chat with Articles feature is where the LlamaIndex Retriever-Augmented Generation (RAG) system shines. This system engages with the user's query by searching through the indexed vectors in the Qdrant Vector Database for related articles.
It then synthesizes information from these articles to generate a comprehensive and concise response. This is where the app becomes interactive, as users can engage in a dialogue with the system, probing deeper into topics of interest or asking for clarifications, much like they would with a human expert.
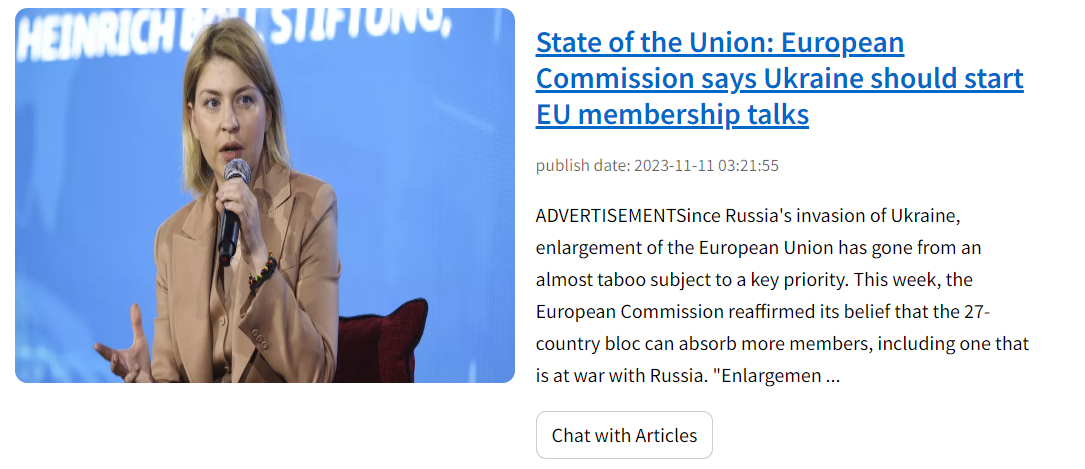

Each query triggers a cascade of events within the LlamaIndex RAG, which pulls together various sources, summarizes, compares, and contrasts information to provide a response that is informative and nuanced. The responses are then presented through the Chat Bot Display, offering users a seamless and engaging experience.
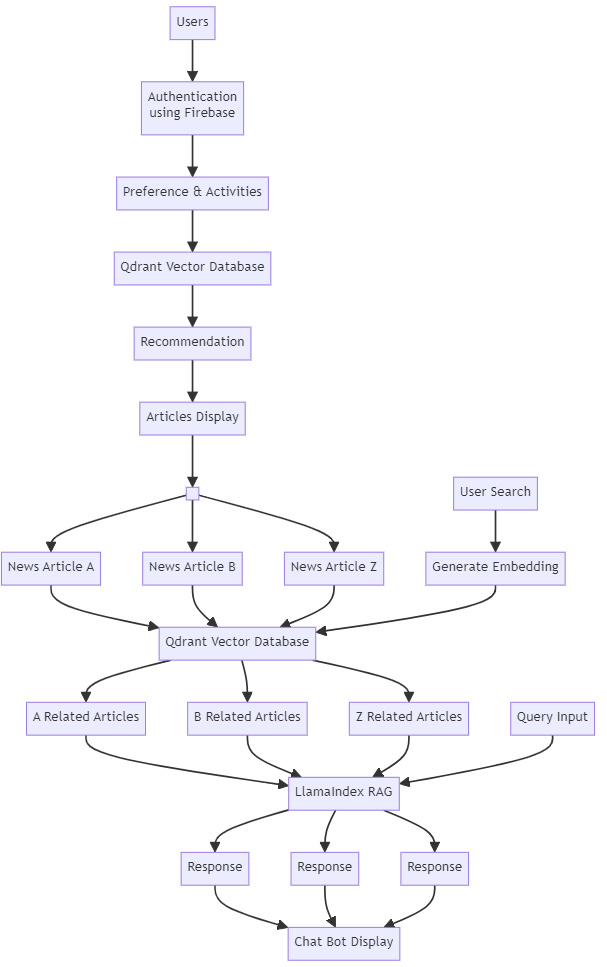
The flowchart provided helps visualize the web of processes that make up the NewsGPT platform. Each component is interconnected from data sourcing to user interaction, creating a harmonious and intelligent system for news consumption.
Core Ideas behind NewsGPT
- Reliable News Sources: NewsGPT's dynamic data pipeline is the backbone of its operation, beginning with a sophisticated algorithm that sources news from credible outlets. This system ensures that the information being summarized is accurate and up-to-date. The application employs advanced Named-Entity Recognition (NER) to identify and categorize key information from articles, while text embedding processes, facilitated by state-of-the-art machine learning techniques, capture the essence of the content. This is all meticulously organized within a Qdrant Vector Database, ensuring that the news is not only reliable but also relevant and ready for rapid retrieval.
- Tailored News Recommendations: Understanding that each user's interests are unique, NewsGPT personalizes the news-reading experience through a learning algorithm that adapts to individual reading habits. By analyzing engagement with different topics and stories, the app refines its news recommendations, creating a bespoke news feed that becomes more aligned with the user's preferences over time. This personalized approach is reflected in the flowchart's depiction of user interaction with the vector database, where preferences and activities inform the recommendation engine.
- Efficient Information Retrieval: NewsGPT simplifies the process of obtaining comprehensive news summaries through a one-click solution. By aggregating and summarizing news from multiple reliable sources, the app provides a multifaceted view of current events. This feature is powered by a Streamlit chatbot, which presents a conversational interface for users to interact with, allowing for an engaging and efficient retrieval of news summaries. The flowchart illustrates how, upon a user's query, the chatbot interfaces with the LlamaIndex RAG to pull responses from a vector store of indexed articles, delivering concise summaries directly to the user.
- Time-Saving Aspects: NewsGPT provides quick summaries. It features a sidebar that displays the amount of time users would have spent reading full articles versus the time taken to read the summaries, offering a tangible sense of efficiency. Moreover, the app provides insights into news category distribution, allowing users to visualize the spread of their news consumption across different topics, as depicted in the flowchart through the various streams of article recommendation and retrieval.
Each of these features comes together to create an app that not only respects the intelligence and time of its users but also enhances their ability to stay informed in an era of information saturation.
Why Build an AI Platform for News?
The consumption of news has seen a profound evolution over the years, driven by technological advancements and changing consumer preferences. From the days when reading the newspaper was a morning ritual, we have journeyed through an era where radio and television brought the world's events into our living rooms, creating a shared experience for families nationwide.
The advent of the 24-hour news cycle and cable networks catered to an ever-growing demand for up-to-the-minute news, while the rise of the internet brought about a seismic shift, as news websites and online editions of newspapers provided real-time updates and a platform for interactive engagement.
This evolution continued with the emergence of social media and blogs, which used news distribution, allowing anyone with internet access to both consume and share information. The mobile revolution and app-based news consumption personalized news delivery, with alerts and feeds tailored to individual preferences.
Now, news aggregators and summarization services are the latest type of news, which condenses information from various sources. Despite the changing landscape, the public's need for reliable, factual news has remained constant, with the latest technologies striving to balance the demand for brief, accessible content with the essential need for accuracy and integrity.
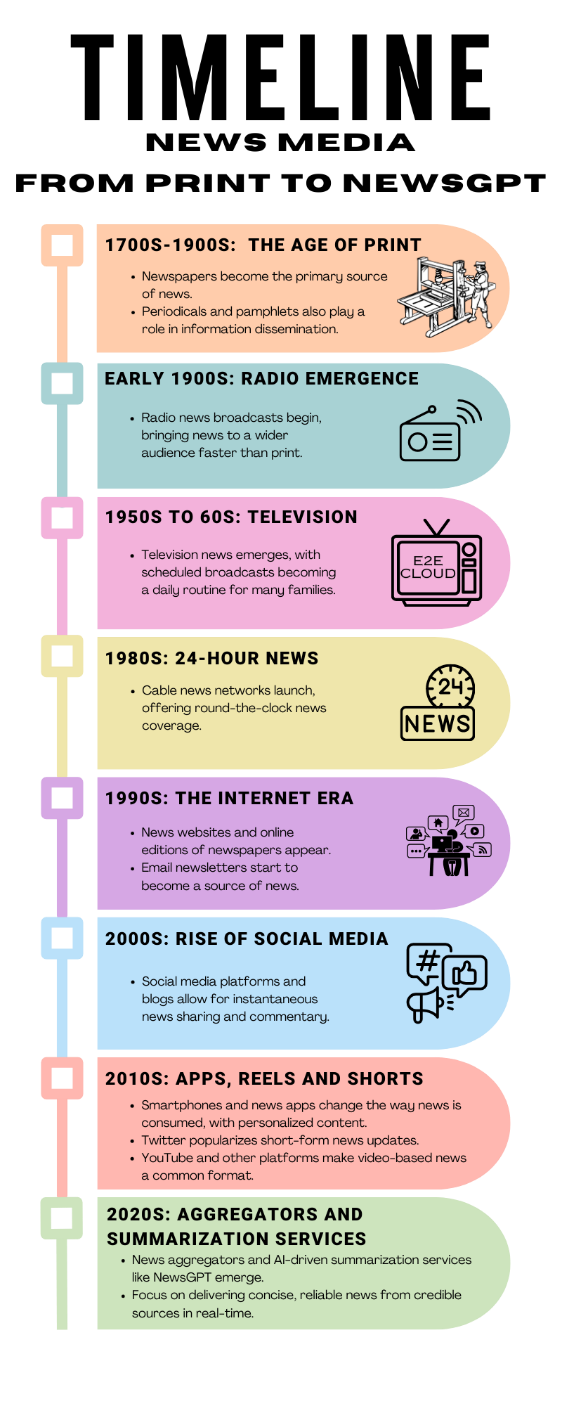
Credible news sources actually do more than report facts; they provide context, depth, and analysis, helping readers understand the complex world around them. They are the antidote to the misinformation spread by clickbait, offering a more nuanced and comprehensive view of events.
In a time where there is a lot of information, but insight is scarce, tools that can differentiate through the noise and present news from reliable outlets are not just convenient; they are necessary for an informed public.
This is where RAG-pipeline driven AI comes in, an approach where contextual data is fetched from credible sources, and thereby generating summaries that are more informed and accurate. NewsGPT uses Apache Spark for Named Entity Recognition, and capabilities of LlamaIndex to filter news articles into concise, digestible summaries from trusted sources.
In the coming future, AI would become part of the workflow in news and media industries.
The Future of News and Conclusion
The future of news is currently at the exciting intersection of technology and information. NewsGPT points to a possible new future direction that technology would possibly take in the news industry, offering a powerful mixture of speed, accuracy, and personalization. Its impact is not just in how news is consumed, but in how it is experienced, turning the passive act of reading into an active dialogue.
The efficiency and responsiveness of AI-driven platforms are heavily reliant on robust computational power. This is where cloud GPU services that provide instant access to advanced GPUs come into play, offering scalable resources that can process large datasets, manage machine learning tasks, and handle user queries in real-time. In India, the company that pioneered advanced cloud GPUs is E2E Networks, offering the most advanced AI Supercomputer HGX 8xH100 to developers and researchers. The way to get started is by heading to MyAccount and signing up.
On E2E Cloud, developers can choose from a range of GPUs, including the powerful A100, H100 and HGX 8xH100 at a nominal price. If you're ready to start building or expanding your AI-driven solutions, you can get started today by signing up. Moreover, E2E Cloud offers a wide variety of other GPUs to suit different needs and budgets, ensuring that you can find the perfect fit for your project.