With written information growing exponentially, the need to break down lengthy texts into manageable, easily understandable sentences or paragraphs is essential for companies to streamline and collate data. It can take several days for an individual to sift through several hundred pages, filter out irrelevant material, and write an easy-to-understand summary. Additionally, sensitive documents, where there is no scope for error, may require extra care.
This is where Text Summarisation plays an important role.
What Is Text Summarisation?
Text Summarisation generates a short and accurate summary of a longer document. It is the process of breaking down lengthy and complex text into smaller chunks summarizing the content. The idea is to find the most essential and most relevant information and present it in a human-readable and comprehensive way, without changing the meaning of the text.
Text Summarisation does not just reduce human effort; it also reduces the time required to understand longer pieces of information without losing their essence.
Text Summarisation is most useful when the text has a lot of raw facts, so that important information can be filtered from them. The NLP models can summarize long documents and represent them in small, simpler sentences. News, factsheets, and mailers fall under these categories.
Types of Text Summarisation
Text summarisation uses several techniques and algorithms to give outputs. Broadly, summarisation can be categorized into three types:
- On the basis of Input Type
- On the basis of Output Type
- On the basis of Purpose
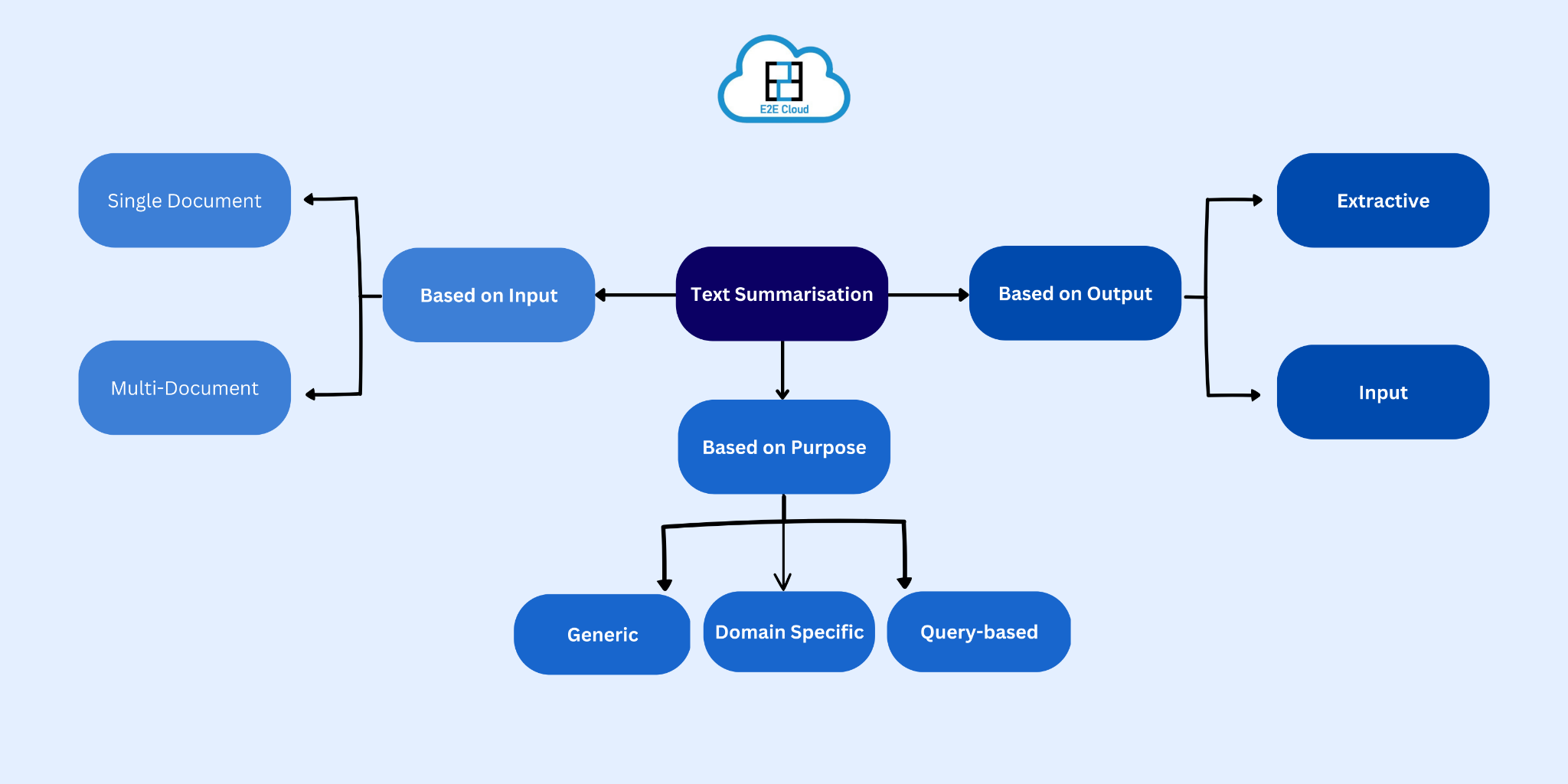
On the Basis of Input type
One way of classifying Text Summarization is based upon input from the source text.
Single Document Summarization: The input, in this case, is from a single document.
Multi Document Summarization: Input is collected from several documents and can be lengthy.
Multi-document summarization can be challenging since it requires the ability to understand the relationship between multiple sources of text and synthesize that information.
On the Basis of Output type
There are two types of output-based classification.
Extractive: Extraction-based Summarisation pulls and combines a subset of words that represent the most important points from a document. To understand this better, we can think of it as a highlighter, which allows us to see the main point of the source text. In Machine Learning, extractive summarization usually involves weighing the essential sections of sentences and using the results to generate summaries.
Abstractive: In contrast to Extractive Summarization, Abstractive Summarisation does not just pick out phrases from the source text. Instead, it uses techniques such as Natural Language Generation (NLG) to come up with a summary that is a unique representation of the source text. It understands the meaning and context of the text and then generates the summary. It requires a deeper understanding of the content and the ability to generate new text without changing the meaning of the source information.
On the Basis of Purpose
On the basis of purpose, we can classify summarisations into three types:
Generic: Generic summarizers make no assumptions about the content of the text. They simply summarize the original source content.
Domain-specific: In this type, domain knowledge is utilized to write a more accurate summary. For example, research papers of a certain domain can be used as source text. They can combine specific context, knowledge and language to write the summary using the source texts.
Query-based: The main focus of this type of summarisation is natural language inquiries. Similar to search engine results, it summarises articles that solve complex topics pertaining to the input data. For example, when we ask a question through Google’s search engine, it shows us web pages or articles that answer our questions.
Benefits of NLP Text Summarisation
NLP text summarisation offers several benefits in various applications, including:
- Saving Time and Effort:
NLP Text Summarization can automatically generate concise summaries of lengthy documents, saving time and effort. This is especially useful in situations where a large amount of text needs to be processed.
- Improved Information Retrieval:
Summarized texts can serve as abstracts or excerpts, making it easier for users to quickly scan and retrieve relevant information from a large corpus of documents. This can enhance information retrieval tasks, such as document search, recommendation systems, or information extraction, by providing users with concise and meaningful summaries of the content.
- Scalability and Automation:
NLP Text Summarisation can be applied to large volumes of text in an automated and scalable manner. This makes it suitable for processing massive amounts of data in real time, such as in news websites, social media feeds, or online forums, where manual summarisation would be time-consuming and impractical.
- Extraction of Key Information:
NLP Text Summarization can also extract and highlight some key information, such as important concepts, keywords, or entities, from the source text, which can be useful for information extraction tasks.
- Customisation and Personalisation:
It can also be customised and personalised to specific domains, industries, or user preferences. This allows for tailored summarisation results that align with specific requirements and can be used in applications such as content curation, news aggregation, or personalized information retrieval.
Datasets Available
Several datasets are available to deploy a model based on text summarisation with NLP. Some of the most popular datasets are:
Gigaword Dataset
This dataset contains over 4 million articles that range across topics such as politics, business, sports, entertainment and more. Each article is typically several hundred words long and comes with a headline, which serves as a summary or a short description of the main content of the article.
WikiHow Dataset
The WikiHow Dataset consists of over 230,000 articles that cover various domains, such as cooking, gardening, technology, health, and more. Each article in the dataset contains multiple steps or instructions, with each step represented as a paragraph. The dataset also includes human-generated summaries, which serve as abstractive summaries of the original articles.
Opinosis Dataset
The Opinosis Dataset is specifically focused on opinion-based text. It was introduced by Kavita Ganesan in 2010 and has been used in Natural Language Processing (NLP) research for abstractive text summarisation tasks. The dataset consists of a collection of product reviews from multiple domains. The dataset also includes human-generated summaries, which serve as abstractive summaries of the opinions expressed in the reviews.
How2 Dataset
It is designed for the task of video-to-text generation, where the goal is to generate textual descriptions or captions of video clips. The dataset contains videos with accompanying transcriptions and translations in multiple languages. The How2 Dataset is publicly available for research purposes and has been widely used in NLP research for video captioning, machine translation, and other related tasks.
MATINF
MATINF contains 1.07 million question-answer pairs with human-labeled categories and user-generated question descriptions. Based on such diverse information, MATINF is applicable to three major NLP tasks, including classification, question answering, and summarization.
Deploying a Model Based on Text Summarisation with NLP
To deploy a model based on NLP Text Summarisation, you can sign in to your My Account portal on the E2E Networks website. After logging in, you will see the following screen. Click on Compute and select GPU from the dropdown menu.

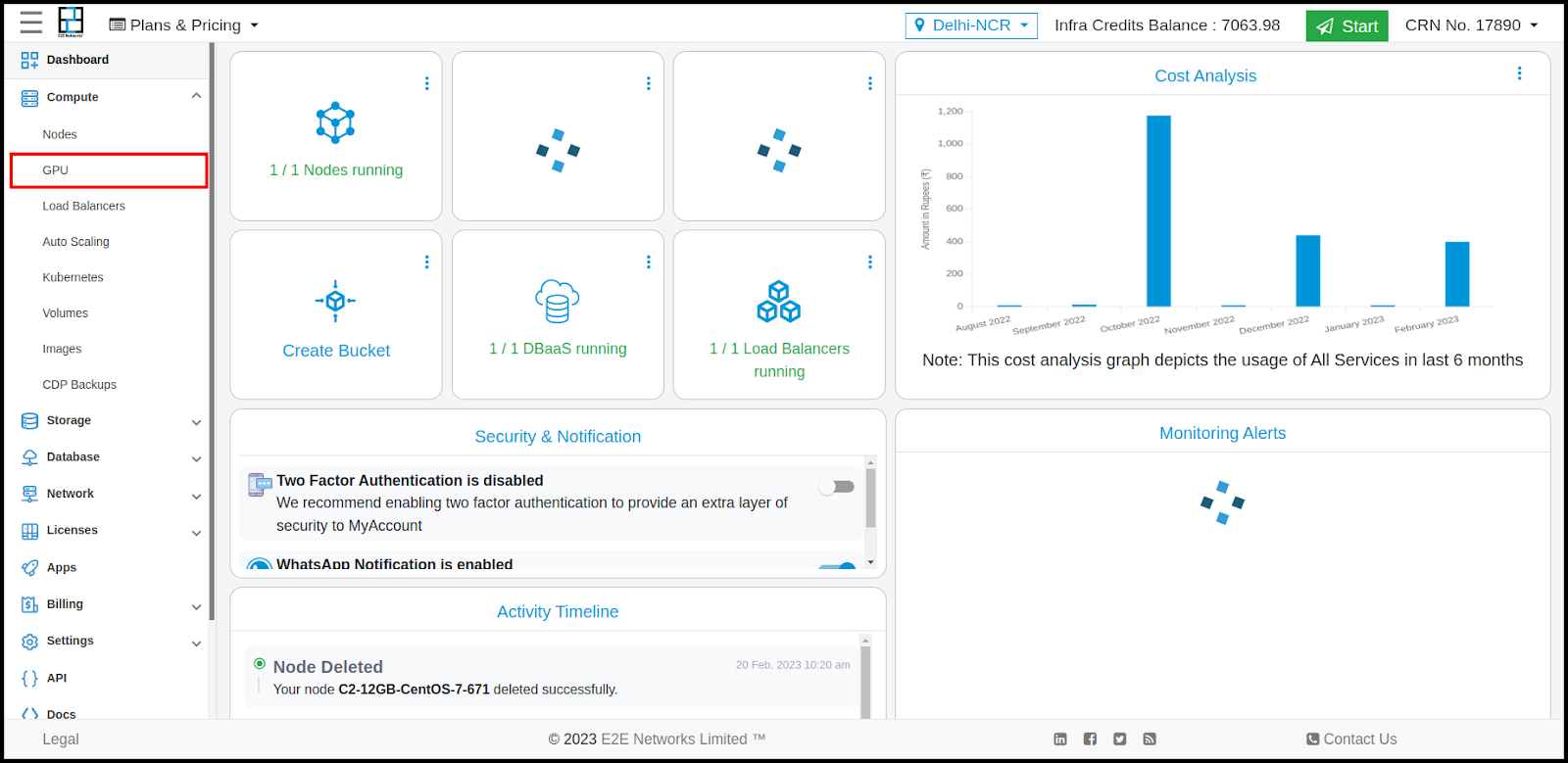
You will see the following screen when you choose GPU Cloud Wizard. Under Cloud Wizard, you will find options for choosing the ML Framework that you want to deploy your model on.

You can select the framework best suited for your requirements, and click on Next.
You can now select whether you want a committed node or an hourly billed node.
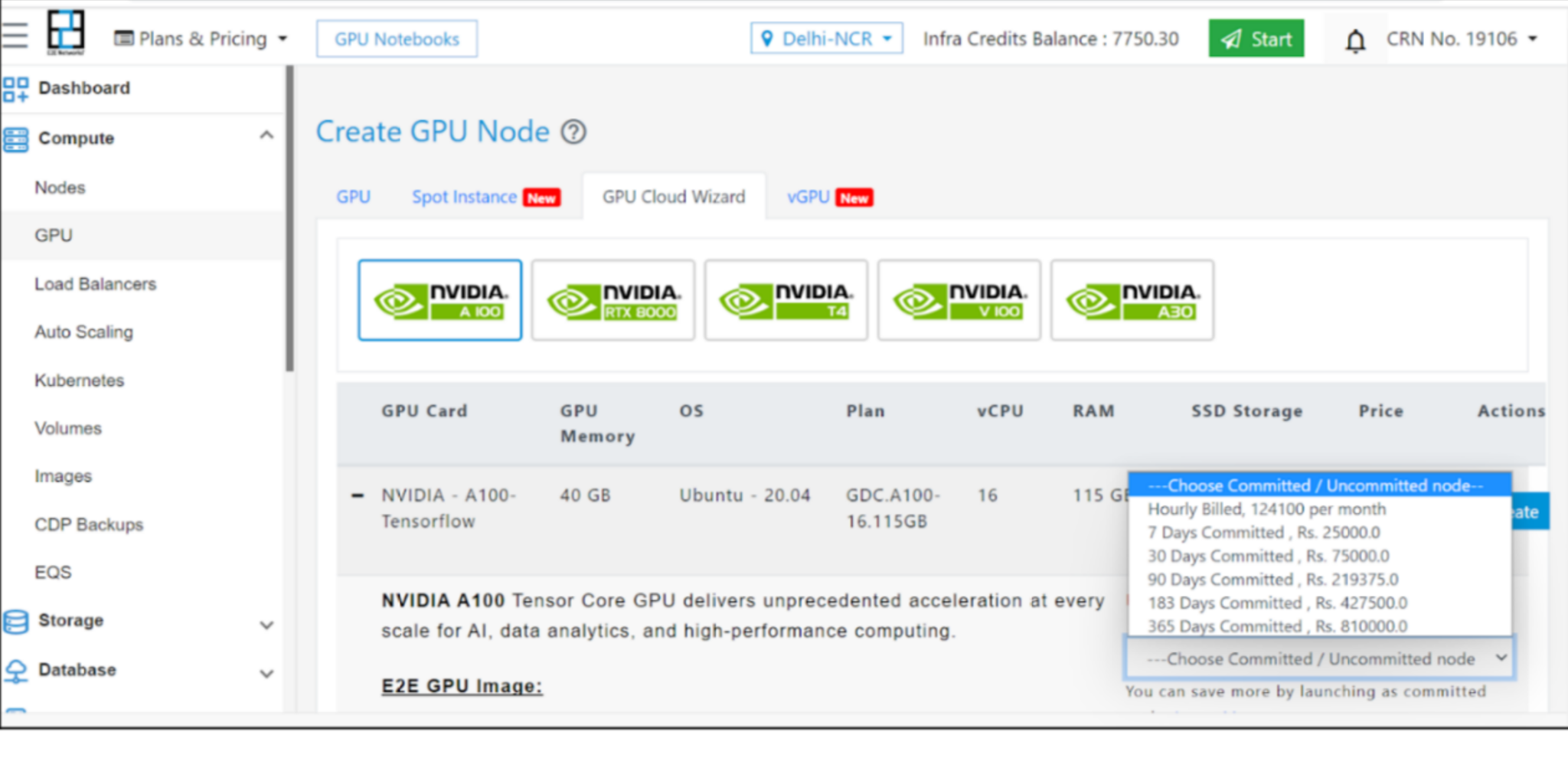
Click on Create to launch the machine.
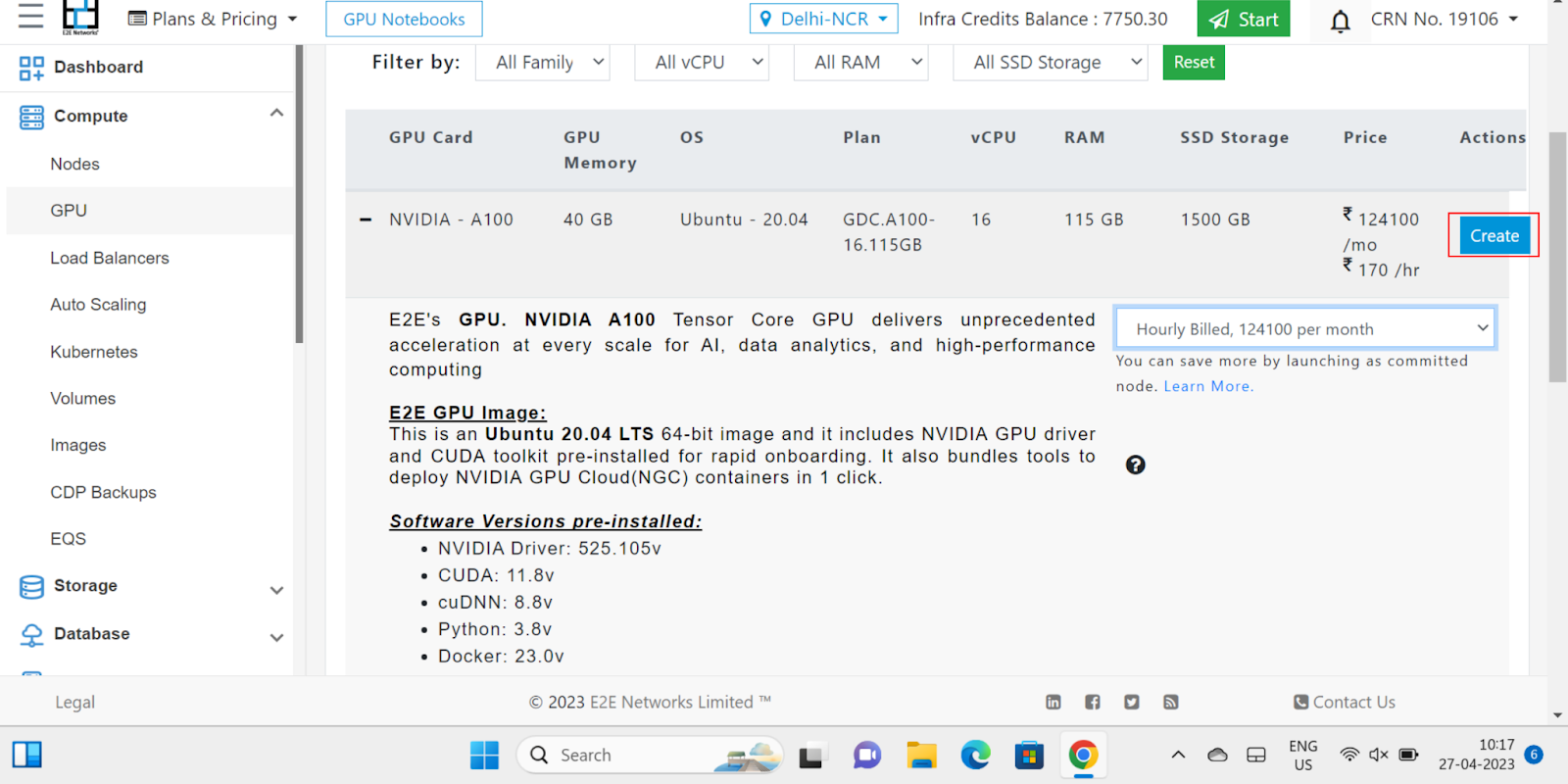
You can see your running machine on the dashboard.

For more information, please get in touch with sales@e2enetworks.com.