I’m sure each of us would have interacted with a chatbot. It might have been to get the status of your order on an e-commerce platform or while chatting with a health helpline number to get a diet plan, and so on. Using Chatbots or Conversational AI technologies can help businesses save a lot of human hours that go into customer service and answering queries. With the advent of Large language Models (LLMs) that are able to comprehend human language with context, the possibilities in the domain of conversational AI bots are at an all time high.
Fine-tuning an LLM on Custom Data
As LLMs are trained on huge amounts of data, they are better at interpreting human language and the intention behind what an user wants. We can leverage this to our advantage by fine-tuning these LLMs on our custom data. During fine-tuning, a LLM will learn the custom training data you provide, incremental to the knowledge it has gained during pre-training. Fine-tuning can help in making chatbots for specific domains like healthcare support, technical documentation support bot, to name a few. The use-cases are varied.
In this article, I’ll explain how to train an LLM with pre-trained weights on a custom dataset along with a code walkthrough.
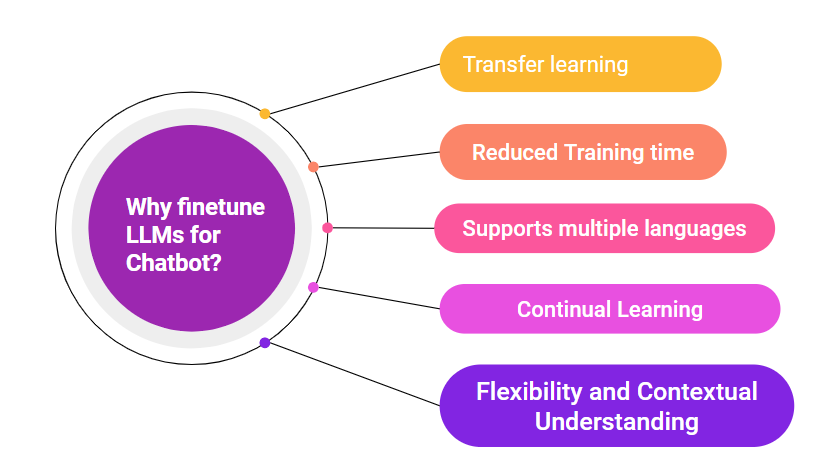
Fig. 1: 5 reasons why you should fine-tune LLMs to make a Chatbot.
Introduction to Llama Large Language Model
I’ll be using Meta’s Large Language Model, Llama. Meta has released a family of large language models that are trained on huge text corpuses and can be used for various NLP tasks like conversational AI, text summarization, sentiment classification, and more. I’ll be choosing the ‘Llama-2-7b-hf’ model available on the Hugging Face platform, which is trained on 7 billion parameters. This is also optimised for dialogue use cases, which suits our needs. There are also Llama models that are pre-trained on 30-70 billion parameters – you can check the list here if you want to see all the options.
Tips Before Starting the Project
We’ll be doing the project using Python on E2E Networks. You need to be familiar with some of the basics of NLP like tokenization. I suggest reading up on the working of transformer models for a better understanding. We’ll be using the pre-trained model weights and datasets from the Hugging Face hub. More relevant concepts include Neural Network Training Pipeline and Hyper Parameter Fine-Tuning.
Step 1: Setting up the E2E Node
Create an Ubuntu 22.04 GPU node on E2E.
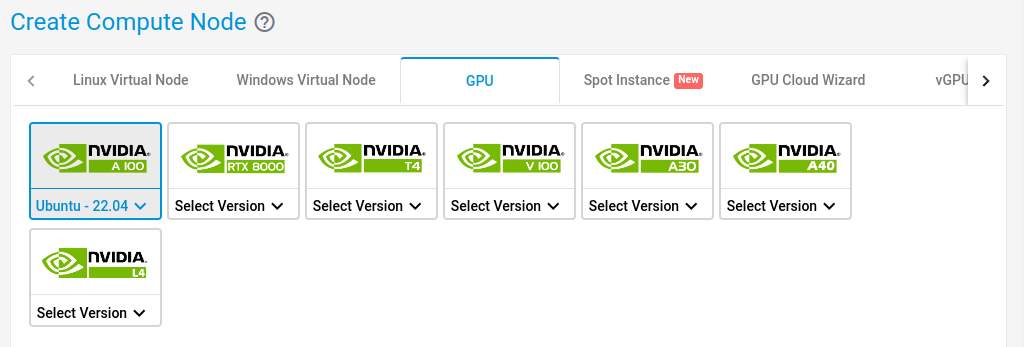
Select a 40GB Machine and hit Create.
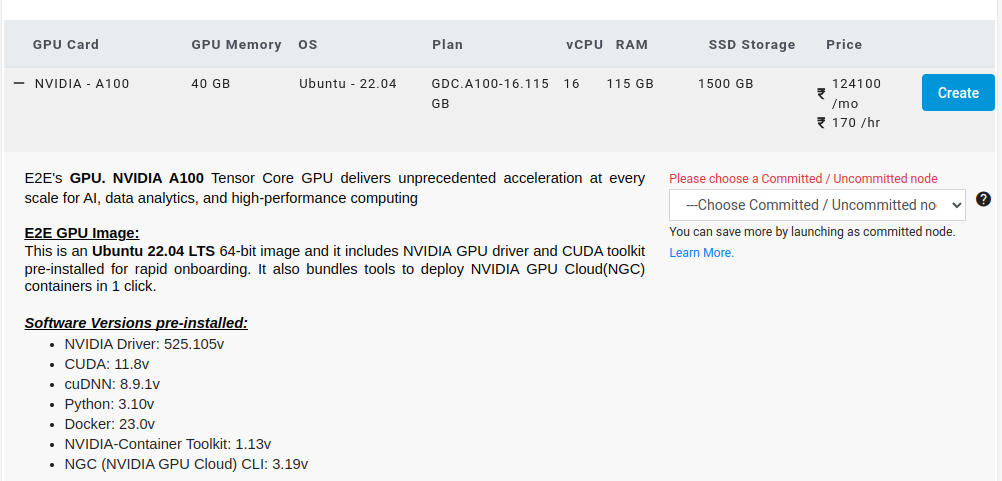
Check on Enable Backup and hit Create.
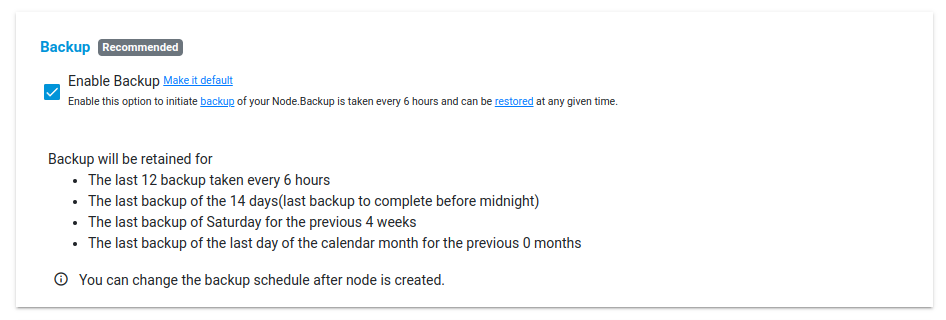
The node will now be created with the following specifications:
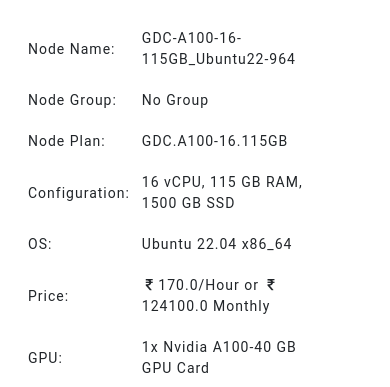
Log in to the E2E server using ssh via terminal:
After you are logged in, cd into /mnt:
Step 2: Install and Import the Libraries
The first step in any Machine Learning project is to install and import the libraries you would require. Let us take a quick look at the different packages we would be working with. First, ‘datasets’ package, which we’ll use to load and work with the datasets available on Hugging Face hub. To perform fine-tuning, we’ll be using a simple and efficient approach with the LoRa tuner. It’s a method that uses an optimised and minimum number of parameters to fine-tune, and can help you save up on the computational resources. To use this, we import the LoraConfig class from the ‘peft’ package. We use Pytorch for the training framework. Run the below code from your terminal.
When you execute the pip command, you should be able to see the packages being collected and installed as shown below.
Once all the packages are successfully installed, next run the below snippet to import the modules and classes you want to use from each package.
We use the torch module for the training pipeline. The ‘transformers’ library is a package of Hugging Face and provides us with various classes to help in LLM fine-tuning. Let’s take a quick look at each component.
- AutoModelForCausalLM: This class will help us to load any pre-trained model available in Hugging Face. It is specific for casual language modelling.
- AutoTokenizer: Tokenization is the process of converting the text input into tokens, which can be further provided to transformer models. There are many tokenizers available to do this task, which can be loaded by the ‘AutoTokenizer’ class.
- BitsAndBytesConfig: It is to configure the parameters for the Bits and Bytes model, which is specialised to perform at low-resource availability.
- TrainingArguments: This functionality provides us an easy way to configure the different parameters we would need to tune, like the number of epochs, learning rate, and so on.
Step 3: Set Up the Quantization Configuration
As the next step, we define arguments for the Bits and Bytes model, which is used to reduce the size of large models, like the Llama-7b in our case.
Wondering What It Does?
The Llama model, by default, is used in 16-bit precision. The Bits and Bytes model will quantize the Llama model’s weights to 4-bit precision. This is a very handy technique when you have limited resources. You need to set the ‘load_in_4bit’ parameter as ‘True’ to enable this.
Step 4: Prepare the Llama Model for Fine-tune
The next step is to load our pre-trained model, for which we use the AutoModelForCausalLM class. This class has a function ‘from_pretrained()’, which can be called to provide our input arguments. The first argument we pass is the model name, which is ‘meta-llama/Llama-2-7b-hf’. Note that you need to apply and fill a form to get access to use this model version released by meta. But, there are alternative public models of Llama 7b made available by various researchers in Huggingface (daryl149/llama-2-7b-hf).
The next parameter is the quantization configuration which we defined in the previous section. To load the model configuration and pre-trained weights from Hugging Face, you also need to pass the Hugging Face token. You can create your token for free! Sign up on Hugging Face if you don’t have an account, go to ‘Settings > Access Token’ and click on Create as shown in the below screenshot.
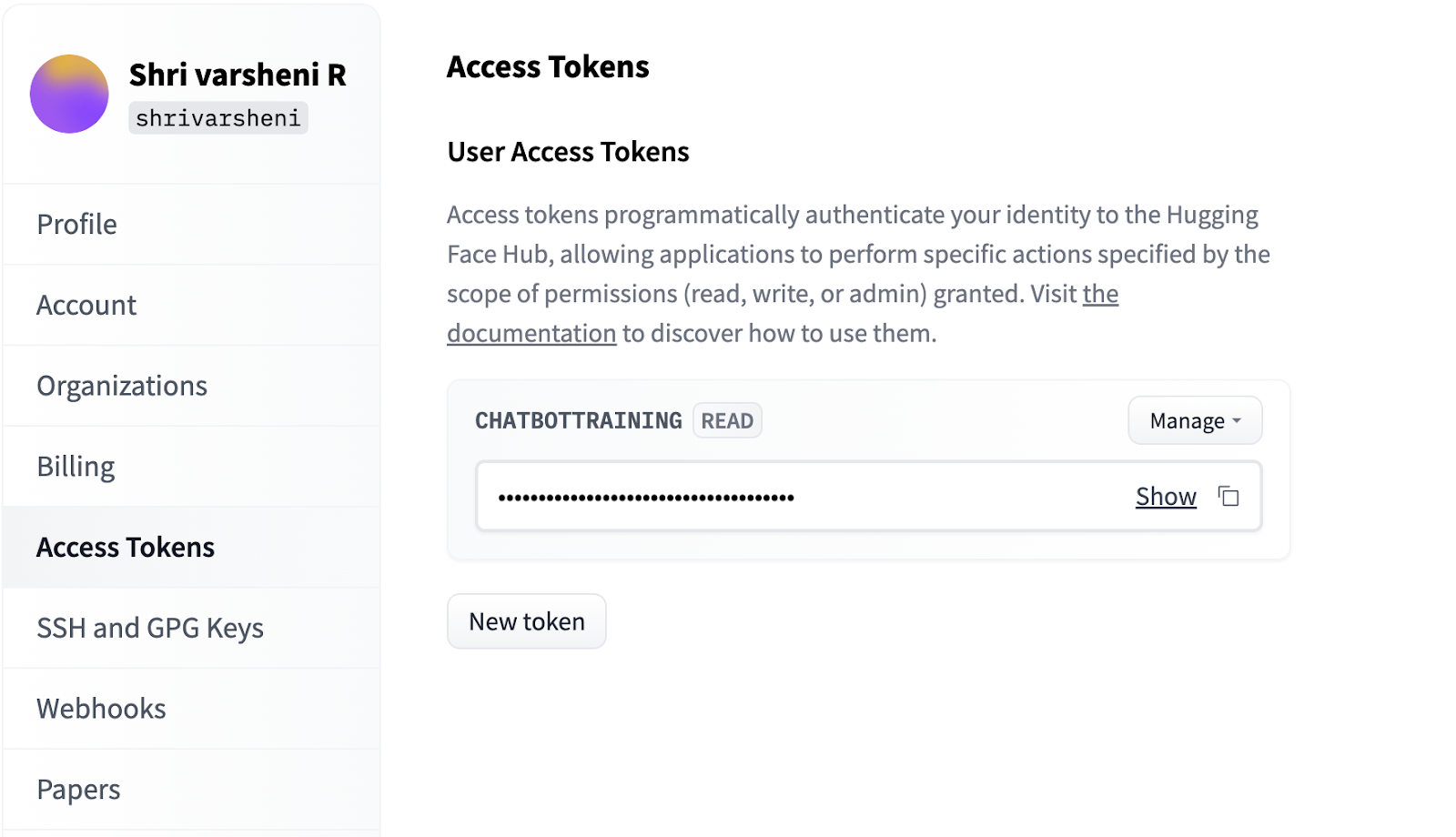
Once you have the token, run the below code. Ensure to replace the ‘PASTE YOUR TOKEN’ with your generated token before executing.
When you run the code, you should see the progress of download of the model files as shown above.To indicate that we want the model to use the pre-trained weights and not start from scratch, we set the pretraining_tp as 1. We have also initialised a tokenizer, to encode the text inputs into desirable format for the model. When we use the AutoTokenizer class with the model name as input, a tokenizer specific to our LLM will be initialised. For a tokenizer, we can specify a pad token to pad the input text to a fixed length. I have specified my pad token as EOS (End of Sentence) token.
Step 5: Use the LoRa Tuner
As we are using the LoRa tuner for fine-tuning, we need to specify the configuration for it. There are a few important parameters you should take note of:
- lora_alpha: This parameter is to control by how much the LLM will adapt to the new task we are fine-tuning on. The higher you set its value to, the higher will the adaptation be and the number of trainable parameters.
- lora_dropout: It is a regularisation parameter. It works just like how we add dropout layers in neural network training to avoid overfitting. This helps the model to not memorise the training examples, and generalise well to new data.
- r: This denotes the attention dimension, which will control the size of the attention window used by the LoRa tuner.
- task_type: It is used to specify the kind of task we are fine-tuning for. The options are casual language modelling (causal_lm) and classification. In our case, we choose the former.
Run the below code to define the Lora tuner.
Step 6: Train the LLM
Now that we have prepared our model and fine-tuner, let's decide on the training arguments and get to training. The important parameters here are the following:
- Learning rate: It is a hyperparameter that will decide the pace at which your model learns. It is advised to choose a slow rate to avoid overfitting and obtain better convergence.
- Warmup_ratio: Before you exercise you do a warm-up, don’t you? Similarly, we want to start slow and then catch up to the learning rate defined over the initial few epochs. I have set it to 0.05, which means the learning rate will be increased over 5% of the training epochs and then it becomes stable.
Next, we load the dataset of our choice by using the ‘load_dataset’ functionality that comes with the datasets package of Hugging Face. I’m choosing a dataset on famous English quotes. This will help us build a bot that people can chat with while looking for inspirational quotes for lectures, social media content, and more.
Run the above code and you should be able to see the progress of download of your dataset as shown. It may take some time to extract 100% of files based on how huge your dataset is.
And here comes the final step! We can use the SFTTrainer class to wrap our model, quantization configuration, dataset and training arguments as shown below. If you call the train() function, the training would get started. When the training is completed, you can save it to your output directory.
Setting `pad_token_id` to `eos_token_id`:0 for open-end generation
Books are your door to wisdom. Room without books is like body without
You can then load the saved model from the directory anytime you want.
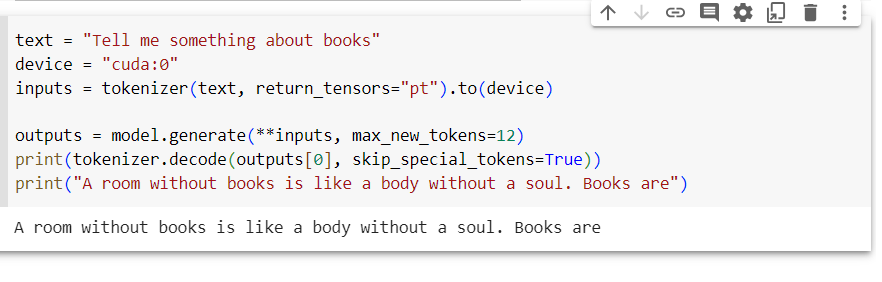
Conclusion
In this blog, we went through the process of how to leverage transfer learning and fine-tune the Llama LLM on a custom Hugging Face dataset. If you have domain specific data, you can convert it into a CSV file and upload it on Hugging Face, and still use the functionalities available. Note that we use quantization techniques to reduce the model size, but it depends upon the size of the LLM and the dataset you are working with. If the size is large, it is advisable to work with multiple GPUs. Similar to this, you can implement LLM-powered chatbots for a wide range of tasks.