Under the umbrella of data science fields, natural language processing (NLP) is one of the most important subfields. NLP is a computer science field that gives computers the ability to understand the natural language(s) of humans. Although NLP has gained a lot of traction in 2023, it is, interestingly, a field that is as old as the computer itself. Today, speech technologies are as popular as written text or video technologies.
In this blog, we will start with the history of NLP and move on to its contemporary applications.
An Introduction to NLP
NLP first appeared as machine translation in the 1950s. These early machine translation efforts helped in decoding messages during World War II. Although the findings were disappointing, researchers at the time believed that machine translation could convert Russian into English. This turned out to be the foundation of developing advanced technologies much later. Prior to the 1980s, the main driving force behind NLP was a convoluted system of manual set of instructions, which was labor-intensive and difficult to implement using any technology. These strategies underpin the NLP that is practiced today.
In 2019, Google introduced AutoML Natural Language as an extension to its AutoML platform [1]. Communicating with machines is eventually becoming as simple as interacting with a human. The ultimate goal of NLP is to help computers understand language as well as we do. As we move forward, NLP will continue to boost industries like machine learning, robotics, healthcare, financial services, linked automobiles, intelligent homes, and more.
This article dives into the evolution of NLP and the latest applications that incorporate NLP to automate human lives. It highlights the core concepts and techniques that enable NLP to communicate with humans. To understand this better, take a look at the latest techniques and innovations used in building an NLP application.

Fig. 1: Overview of NLP in the sphere of AI.
What Is Natural Language Processing (NLP)?
Natural Language Processing is a subfield of Artificial Intelligence (AI) which assists computers and machines to understand human language. It enables computers to interpret and manipulate human language in order to automate the tasks between machines and humans.
Businesses who have begun to use NLP are seeing an increase in performance and satisfaction among their clients. NLP is especially beneficial for companies that have a significant amount of data, since it helps them to interpret the data and produce meaningful business insights. Additionally, it offers consumers a customized service via chatbots, which boosts client retention ratios and profitability. Fig. 2 showcases various real-world applications like chatbots, virtual assistants, question-answering, sentiment analysis, text summarization, language translation and social media analyses. These are the most popular and widely used applications among diverse domains ranging from business, education, healthcare, and finance to automobiles, marketing and advertising.

Fig. 2: Most widely used real-word applications of NLP.
Key NLP Techniques and Algorithms
NLP requires various techniques and algorithms to precisely analyze and understand human language. Fig. 3 mentions the most frequently used fundamental techniques in NLP.

Fig. 3: The fundamental techniques of NLP.
Step #1. Set Up the Development Environment
Choosing the Right Programming Language and Frameworks
Python is a robust programming language which has the potential to be developed by NLP methods for computational languages. NLP applications were previously available to professionals who were proficient in implementing techniques, languages, machine learning, computing and mathematics. Python offers an ideal environment and set of tools that standardize text processing, allowing researchers to set their sights on creating superior NLP applications. Python empowers designers with a wide range of NLP tools and packages that helps in building a variety of NLP techniques.
Python has become a chief leading language when it comes to Machine Learning tasks or any NLP task. Fig. 4 describes the most popular libraries offered by Python, which include NLTK or Natural Language Toolkit, spaCy, Gensim, TensorFlow and PyTorch.
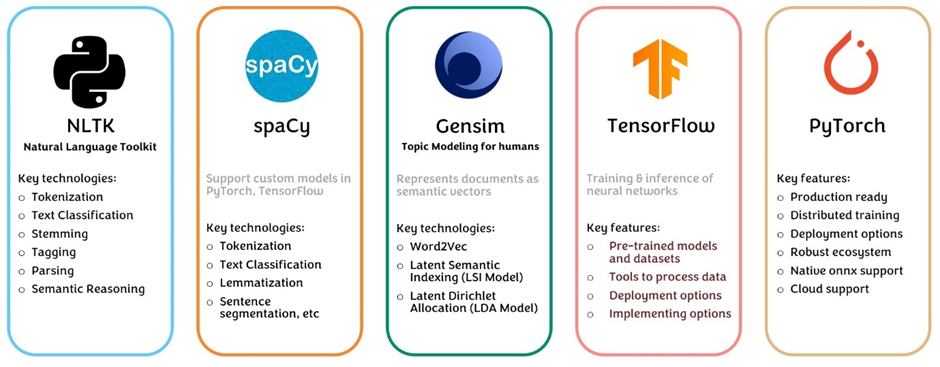
Fig. 4: Most popular libraries in Python.
Installing and Configuring
Set up Python and required dependencies, install libraries and tools (IDE, virtual environment).
Make sure python or anaconda is installed on your computer. The next step is to install NLTK, which is the most important library while building any NLP application.
Text Data Processing (described in the section below)
Write in brief about data collection, corpus creation, web scraping techniques, text cleaning, and preprocessing.
Step #2. Building NLP Models

Fig. 5: Building blocks of an NLP application.
Text Data Processing
Some of the commonly used text pre-processing techniques are tokenization, stopwords removal, stemming and lemmatization, and parts of speech (POS) tagging.
- Tokenization
Machines find it challenging to comprehend the semantics and context of a sentence or paragraph. Tokenization is the process of breaking down words or sentences into semantic units known as ‘tokens’. This enables us to deal with shorter chunks of text, which can be easily understood even when read independently from the context of the text. Fig. 6 explains the process of tokenization.
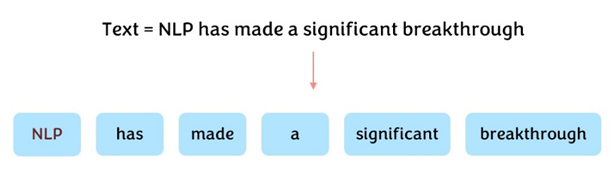
Fig. 6: The process of tokenization.
- Stop Words Removal
Stop Words Removal is a text pre-processing technique that eliminates words with zero significance so that the model can focus on meaningful words. Eliminating such words does not change the meaning or the context of a sentence. While they must be eliminated to avoid junk space in the database and consequently enhance the computation time.
Stop words include - the, a, I, an, be, you, had, has, were, was, will, does, is - and many more. Fig. 7 illustrates the elimination of stop words.
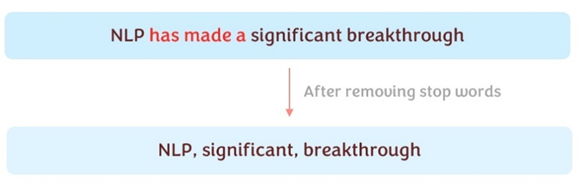
Fig. 7: Stop words removal.
- Stemming & Lemmatization
It is the process of analyzing variation of words to reveal their origin or root word. This pre-processing technique helps in understanding the context of a sentence.
Stemming reduces the words to their root form. It eliminates duplicate words with identical relevance to ensure tasks are performed more accurately. It works by removing the last letters of the word. Fig. 8 illustrates the working of stemming by removing the last letters of a word.
Lemmatization also reduces the words to their original form, but they perform analysis in a group of words to find its root word, which is also known as ‘Lemma’.
Lemma is a word that represents a group of words (‘recover’ as in Fig. 8). This group of words is called ‘lexeme’ (recover, recovering, recovers, recovered as in Fig. 8).

Fig. 8: Stemming and Lemmatization.
- Word Embeddings
Word Embeddings is an NLP technique where words are converted into numbers or vectors. To convert text into numerical format, significant techniques are required which are called Vectorization or Word Embeddings in NLP. There are various word embedding methodologies, which include TF-IDF (Term Frequency Inverse Document Frequency), BOW (Bag-of-Words), Count Vectorization, N-grams Vectorization, Word2Vec and GloVe.
o Word2Vec
- The process of ‘word embeddings’ involves turning each word into a numerical representation of the word (a vector).
- Each word is converted into a single vector, which is then trained in a manner resembling a neural network.
- Based on a word's usage in the text, Word2Vec can infer a word's meaning with a high degree of accuracy.
o GloVe
- GloVe represents a global vector, which is an unsupervised learning technique that generates word vector representations.
- The advantage of GloVe is that it integrates global statistics to build word vectors, while Word2Vec depends on local statistics (local context knowledge about words) for generation of word vectors.
- It is based on word-context matrix factorization algorithms. In GloVe, a co-occurrence matrix is used to determine the semantic relationship between words.
NLP Model Selection and Architecture Design
Model Selection is the process of selecting an appropriate machine learning model or deep learning model for an NLP application. The dataset will be divided into training and testing sections, based on which the model will be trained and then tested.
Training NLP Models
NLP pre-trained models are useful for NLP tasks like translating text, predicting missing parts of a sentence or even generating new sentences. NLP pre-trained models can be used in many NLP applications such as chatbots and NLP API, etc.
Performance Evaluation Metrics
To evaluate and interpret natural language data, such as text or speech, NLP requires an integration of machine learning techniques. The most common evaluation metrics include accuracy, precision, recall and f1-score.
TP: True Positive Prediction
TN: True Negative Prediction
FP: False-Positive Prediction
FN: False-Negative Prediction
Accuracy = (TP + TN) / (TP + TN + FP + FN)
Precision = (TP) / (TP + FP)
Recall = (TP) / (TP + FN)
F1-Score = 2 * (Precision * Recall) / (Precision + Recall)
Step #3. Deploying the NLP App
Deploying an NLP application is carried out once the model is trained and tested. Using MLOps tools, a model's training and inference process can be monitored to assure model consistency.
A key part of machine learning technology is MLOps, which is concerned with accelerating the process of bringing machine learning models into production and concurrently keeping track of them. Some MLOps platforms include TensorFlow, MLFlow, Amazon SageMaker, Microsoft Azure, Google Cloud, etc. As described in Fig. 4, TensorFlow and PyTorch offer great deployment options, where NLP applications can be deployed easily.
Step #4. Building the Pipeline
Integration with Web Applications - same as building an NLP pipeline.
Step #5. Evaluating and Improving NLP Applications
Performance Evaluation Metrics (described in the section above).
Handling Real-World Challenges
NLP is being used extensively by businesses to develop corporate solutions that are beneficial in streamlining activities, enhancing profitability, and automating workflows. NLP incorporates a variety of spheres, such as Computing Science, Linguistics, Artificial Intelligence, Machine Learning and Deep Learning. With the help of these technologies, machines have been able to analyze and understand human language along with meaning, sentiments and intent. Some real-world applications that a human comes across in daily lives are virtual assistants (like Siri), autocorrect spellings in devices, speech-to-text search provided by Google, and many more.
Have you thought, ‘How can models make certain decisions?’
One of the major challenges here is explainability, which has been driving the attention of researchers. As a result, NLP models have become complicated to understand. It has become a challenge to understand and interpret how certain decisions are made by a model.
Conclusion
NLP is a lucrative field with a promising future. It has been a constantly expanding technology that attempts to connect machines and human behavior. Future growth in this industry is going to be overpowering due to the ongoing innovation and research.
There is a great demand for experienced specialists and skilled professionals because this subject has been the focal point of AI. This is a fantastic opportunity to advance your skills if you're enthusiastic enough to learn and build an NLP application.
The Role of E2E Cloud
On E2E Cloud, you can train, build and launch your NLP application on TIR, the GPU and CPU-backed Machine Learning platform powered by Jupyter Notebook. Free credits are available for all new users, along with a choice of frameworks and GPUs to launch with. Try it out today.
References
1.Ekaba Bisong, Building Machine Learning and Deep Learning Models on Google Cloud Platform: A Comprehensive Guide for Beginners, Apress, 2019, pp. 599-612.