The union of Artificial Intelligence (AI) and Software as a Service (SaaS) has emerged as a transformative technology that is reshaping the future of SaaS. In this blog, we explore the synergy between AI and SaaS, dispelling concerns about one overshadowing the other.
We have also provided links, wherever relevant, to tutorials that provide a step-by-step guide to using and deploying the corresponding AI technology.
A Deep Dive into Emerging AI Technologies
Before we dive into how SaaS workflows will shift in the near future, let’s first run through the new AI models that have emerged recently, the most powerful of which being Generative AI.
Generative AI, a rapidly evolving field within artificial intelligence, is designed to generate new content by learning from existing data. This technology encompasses a broad range of applications, including the creation of text, images, music, code, and more. It operates using advanced machine learning techniques, with transformer models like GPT (Generative Pre-trained Transformer) and neural networks forming the core of its architecture.
Generative AI's applications are remarkably diverse. In the field of text and natural language processing, it powers large language models (LLMs) capable of generating coherent and contextually relevant text, assisting in tasks like machine translation and content creation. When applied to code, these models can assist in software development by generating source code and suggesting fixes (known as AI coding assistants). In the realm of images and video, generative AI has made significant strides, with models like Stable Diffusion capable of producing high-quality art from textual descriptions in both audio and video formats. Similarly, in audio, it has enabled the creation of natural-sounding speech synthesis and music generation based on text descriptions.
Some of the most powerful open-source Generative AI models that have emerged in recent times are:
Mixtral 8x7B Language Model
Mixtral 8x7B, developed by Mistral AI, is notable for its powerful and fast performance, adaptable to a wide range of use cases. The model's efficiency is highlighted by its ability to match or outperform the Llama-2 70B model (discussed below) in various benchmarks, while being six times faster. This efficiency is further accentuated by its capability to handle an extensive context of 32,000 tokens and support multiple languages, including English, French, Italian, German, and Spanish.
The underlying architecture of Mixtral 8x7B is a decoder-only sparse mixture-of-experts network, which allows it to increase parameters while managing cost and latency effectively. This approach is critical in scaling the model's performance across different tasks and languages. Moreover, Mixtral 8x7B demonstrates improvements in reducing hallucinations and biases, showcasing more truthful responses and less bias compared to models like Llama-2. Its proficiency in multiple languages is also confirmed through its success in multilingual benchmarks.
A notable aspect of Mixtral 8x7B is its use of the Mixture of Experts (MoE) framework. This architecture includes multiple ‘expert’ networks, each designed to handle specific types of data or tasks. A ‘gating network’ dynamically directs input data to the most appropriate expert, allowing the network to increase its capacity without a corresponding surge in computational demand. This conditional activation makes the process efficient by concentrating computational resources where they are most needed.
To understand how to train and fine-tune this model, you can try out the steps here (but replace the model itself). We will be releasing our guide on the Mixtral 8x7B powered RAG pipeline in an upcoming blog, so stay tuned.
Llama-2 Language Models
Llama-2 is an auto-regressive language model based on an optimized transformer architecture. The model comes in various parameter sizes, including 7 billion, 13 billion, and 70 billion parameters. Its training involved a new mix of publicly available online data, totaling 2 trillion tokens, with fine-tuning data including over one million human-annotated examples. The larger models, such as the 70B version, utilize Grouped-Query Attention (GQA) for improved inference scalability.
The fine-tuned version, known as Llama Chat, employs supervised fine-tuning (SFT) and reinforcement learning with human feedback (RLHF) to align with human preferences for these attributes. Llama-2 demonstrates superior performance on many external benchmarks, excelling in areas such as reasoning, coding, proficiency, and knowledge tests. The model has been evaluated on various academic benchmarks, including code, commonsense reasoning, world knowledge, reading comprehension, and math.
Falcon Models
The Falcon models, developed by the Technology Innovation Institute (TII) in Abu Dhabi, represent a significant advancement in the field of generative AI and large language models (LLMs). These models, particularly the Falcon 180B, have been launched to enhance generative AI capabilities, offering powerful tools for a range of applications from chatbots to code generation.
The Falcon 180B, a prominent model in the series, is noted for its exceptional performance and scalability. It is a generative large language model with a staggering 180 billion parameters, making it one of the most powerful and extensive open-access models available. Trained on approximately 3.5 trillion tokens, it includes a diverse dataset comprising mostly web data from RefinedWeb (~85%), along with a mix of curated data such as conversations, technical papers, and a fraction of code. This extensive training has enabled the Falcon 180B to achieve top performance in various benchmarks, surpassing models like Meta’s Llama-2 and OpenAI's GPT-3.5 in tests including reasoning, coding, proficiency, and knowledge. Notably, Falcon 180B ranks highly on the Hugging Face Leaderboard, indicating its efficacy in AI tasks.
For large scale language model requirements, Falcon 180B is the best out there currently, and you can read a guide on deploying and using it here.
Stable Video Diffusion
Stable Video Diffusion (SVD), introduced by Stability AI, represents a significant leap in the realm of generative AI, specifically focusing on video synthesis. This development follows the success of its predecessor, Stable Diffusion, which was centered on image generation. SVD marks a major step in the field of generative video models by transforming static images into dynamic video content through advanced AI algorithms.
SVD is presented in two primary image-to-video models. The first, known as img2vid, is trained to generate 14 frames of motion at a resolution of 576x1024, while the second, img2vid-xt, is a fine-tuned version of the first, capable of generating 25 frames at the same resolution.
These models can generate short video clips from image inputs and are adaptable to various downstream tasks, including multi-view synthesis from a single image. This adaptability makes SVD a versatile tool for numerous sectors like Advertising, Education, and Entertainment. Notably, early evaluations indicate that these models surpass some of the leading closed models in user preference studies, showcasing Stability AI's commitment to delivering competitive AI solutions.
SVD is available for experimentation and research purposes, with the code and model weights accessible on Stability AI's GitHub repository and Hugging Face page. You can read up steps to deploy it on E2E Cloud here.
Stable Diffusion Image
Stable Diffusion, a cutting-edge text-to-image model developed by Stability AI, has seen rapid advancements, with the latest versions showcasing remarkable improvements in image generation capabilities.
Released in November 2022, Stable Diffusion 2.0 marked a significant step forward from its previous version. It introduced new text-to-image diffusion models trained using a novel text encoder, OpenCLIP, developed by LAION. This new encoder significantly enhanced the quality of the generated images compared to earlier releases. The models in this release can generate images with default resolutions of 512x512 pixels and 768x768 pixels, trained on an aesthetic subset of the LAION-5B dataset. Additional features in Stable Diffusion 2.0 included an Upscaler Diffusion model for enhancing image resolution, a depth-guided stable diffusion model for creative applications, and an updated inpainting diffusion model for intelligent and quick image editing.
Following this, Stable Diffusion 2.1 was introduced, further refining the model's capabilities. This version, available in both 768x768 and 512x512 resolutions, was fine-tuned on the 2.0 version with less restrictive NSFW filtering of the LAION-5B dataset. The attention operation in the model defaults to full precision, and the option for fp16 precision is available, though it may cause numerical instabilities. This version continued to build on the robust features of 2.0, further pushing the boundaries of text-to-image synthesis
The most recent and advanced release, as of July 2023, is Stable Diffusion XL 1.0. This version contains 3.5 billion parameters and can yield full 1-megapixel resolution images in seconds across multiple aspect ratios. Notably, it delivers more vibrant and accurate colors, better contrast, shadows, and lighting compared to its predecessors. One of the key highlights of Stable Diffusion XL 1.0 is its improved text generation ability, enabling it to generate images with legible logos, calligraphy, or fonts, a challenge for many text-to-image models. Furthermore, this model supports inpainting, outpainting, and image-to-image prompts, offering users creative flexibility. It is more user-friendly, capable of interpreting complex designs with basic natural language processing prompting.
To understand how to fine-tune Stable Diffusion XL, read this article.
Whisper - Open Source Text-to-Speech
Whisper, developed by OpenAI, represents a notable advancement in automatic speech recognition (ASR) technology. Trained on a massive 680,000 hours of multilingual and multitask supervised data collected from the web, Whisper demonstrates a robustness and accuracy that approaches human-level performance in English speech recognition. This extensive and diverse dataset contributes significantly to the model's ability to handle various challenges, such as accents, background noise, and technical language. Furthermore, Whisper is not only capable of transcription in multiple languages but also offers translation from those languages into English, widening its applicability in global contexts.
The architecture of Whisper is based on a simple yet effective end-to-end approach, utilizing an encoder-decoder Transformer model. Audio input is processed into 30-second chunks, converted into a log-Mel spectrogram, and then passed into the encoder. The decoder is trained to predict the corresponding text caption, including special tokens that enable the model to perform a variety of tasks like language identification, multilingual speech transcription, and speech-to-English translation. This multitasking ability makes Whisper a versatile tool, capable of replacing many stages of traditional speech-processing pipelines. One thing to note is, Whisper's performance varies across languages, with its effectiveness demonstrated through lower word error rates or character error rates in various languages, as evaluated on different datasets.
Read up our guide on deploying Whisper here.
Audio Synthesis
The field of open-source audio generation and audio synthesis technologies has seen remarkable advancements recently. One such project is Audiobox, developed as a successor to Voicebox by Meta. Audiobox integrates audio generation and editing capabilities for speech, sound effects, and soundscapes. It allows users to generate audio using natural language prompts, making it easier to create diverse audio content, such as soundscapes or specific speech styles.
Another notable project is AudioCraft, a part of the broader AudioGen initiative. AudioCraft focuses on music generation, enabling the creation of various music genres through textual prompts. It's built upon the concept of encoding and tokenization of audio, where raw audio signals are converted into a discrete representation, allowing for high-fidelity AI-generated music and sound. AudioCraft is open-source, allowing anyone to experiment with its pre-trained models or train custom models using their datasets.
The Classic SaaS Stack
A typical SaaS tech stack is divided into two primary segments: the front-end and the back-end.
The front-end of a SaaS stack is what the users interact with. It is responsible for the user interface and the overall user experience. Key components in the front-end stack include HTML, CSS (through Tailwind, Bulma, Foundation or other frameworks), and JavaScript (through React, Svelte or Vue frameworks). HTML is used to structure the content on a web page. CSS is employed for styling and polishing the web page, making it more visually appealing and user-friendly. JavaScript enhances interactivity, allowing for dynamic updates and responsive design elements like pop-ups, contact forms, and sitemaps. Front-end technologies ensure that the user interface is convenient, easy to navigate, and clearly structured.
The back-end is the server-side of the stack and where the core logic of the application resides. It includes a combination of frameworks, programming languages, servers, and operating systems. Popular programming languages for back-end development include Python (or Django frameworks), PHP (or Laravel framework), Ruby (with its Rails framework), or others. The back-end also involves databases like MongoDB, MySQL, and PostgreSQL, and DevOps tools like Jenkins, Docker, Kubernetes, and the ELK stack for automating and managing tasks.
Additional Considerations of AI-Powered SaaS Applications
The integration of AI mandates that SaaS companies rethink their architecture, and plan the AI architecture to deploy. There are several key steps to that.
The following factors are essential considerations, no matter which AI model you are planning to use. For instance, to deploy LLM endpoints, you would need to choose the right cloud GPU, hone down on the right architecture for a RAG pipeline, perhaps fine-tune your model, and then deploy it through an AI endpoint that your backend or frontend can access. A similar approach would work for Stable Diffusion or Audio models.
Here’s a list of the factors to consider.
Choice of GPU Provider for Deploying AI Models
First and foremost, AI models require access to advanced cloud GPUs, and therefore choice of the right provider is essential. Note that your choice of the GPU provider can be independent of where your current infrastructure is hosted – this is typical of multi-cloud setups that have emerged of late.
The key factor to consider should be:
- Access to Advanced Cloud GPU Servers: Is your provider able to offer you the best of AI GPUs available in the market? For instance, HGX 8xH100, the most powerful AI supercomputer that is also the most cost-effective in training large models, is currently available only with E2E Cloud, who pioneered this cloud GPU in India.
- Price-Performance Ratio: The other factor to consider is the price-performance ratio you are able to get in the market, as the TCO (total cost of ownership) can add up incrementally over time when you compare more expensive cloud providers.
- Adherence to Data Laws: This is another key factor to keep in mind, as AI regulations are coming. This would mean that your dataset would need to be protected and it should adhere to the laws of the land. Furthermore, you should protect your data and stack from seizure by foreign actors. For this, SaaS architects should look at fully compliant cloud providers who are located in India, as they are governed purely by Indian IT laws.
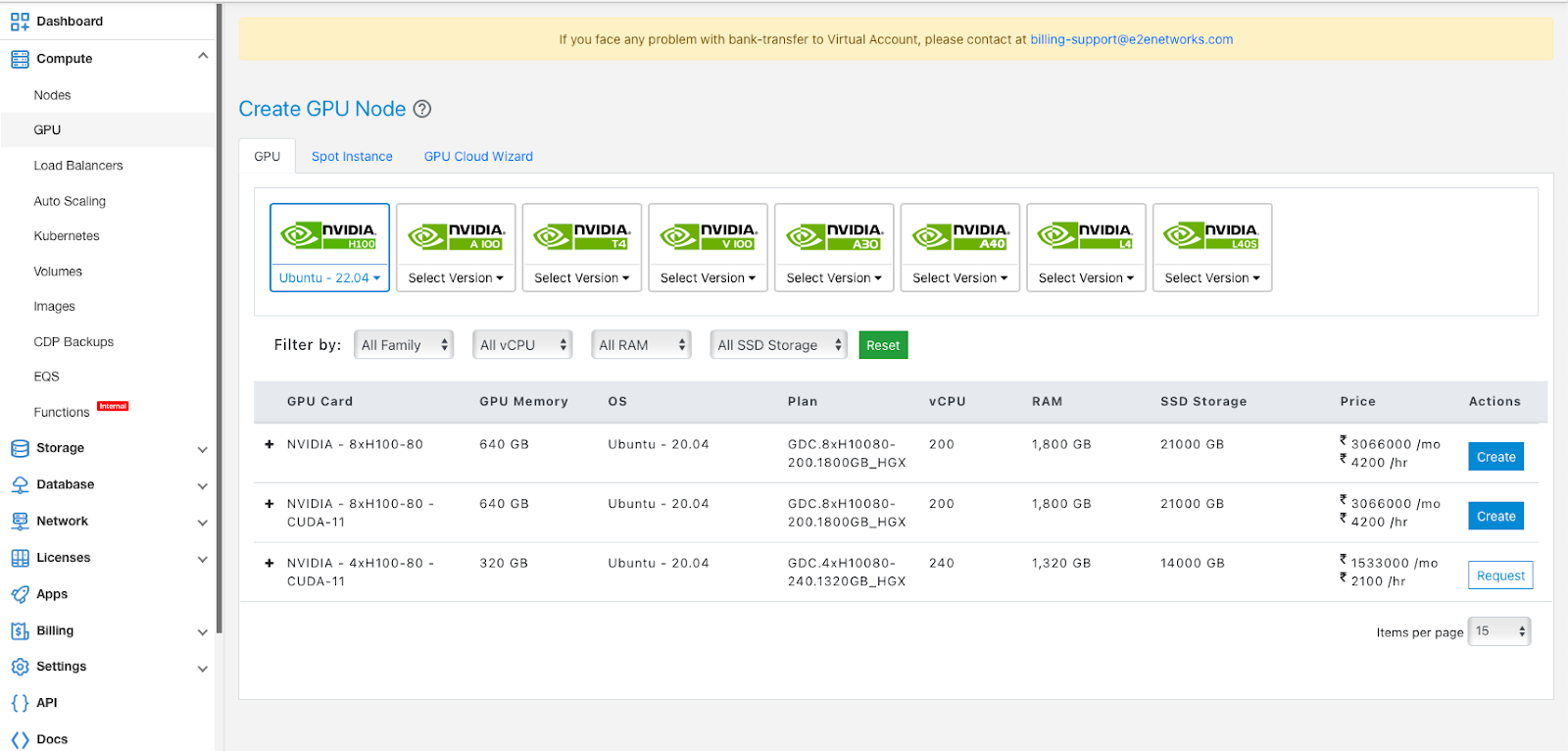
RAG Pipeline, Vector Databases and Knowledge Graphs for Grounding AI Models
Another key factor to keep in mind is that the latest approach to ‘grounding’ the AI to answers that are accurate, in context, relevant, and without hallucination is through use of a technique known as Retrieval Augmented Generation (RAG).
Knowledge graphs and vector databases are two primary technologies considered for implementing RAG in LLMs. Knowledge graphs excel in providing accurate, reliable, and explainable data to LLMs. They are effective in handling complex queries by traversing a graph connected by relationships, thereby returning precise information.
In contrast, vector databases may struggle with complex queries and tend to provide incomplete or less relevant results due to their reliance on similarity scoring and a predefined result limit. They can connect factual pieces of information but sometimes infer inaccurate conclusions. Knowledge graphs stand out for their ability to follow a flow of connected information, resulting in consistently accurate and explainable responses.
However, both these technologies have their unique applications and advantages. Vector databases, optimized for querying complex relationships between data and semantic meanings, represent data as entities (nodes) and their relationships (edges). They can be useful for tasks that involve finding the middle ground within a vast vector space of subjects. On the other hand, knowledge graphs, with their human-readable representation of data, are more transparent and allow users to trace back the pathway of a query, identify misinformation, and make necessary corrections to improve LLM accuracy.
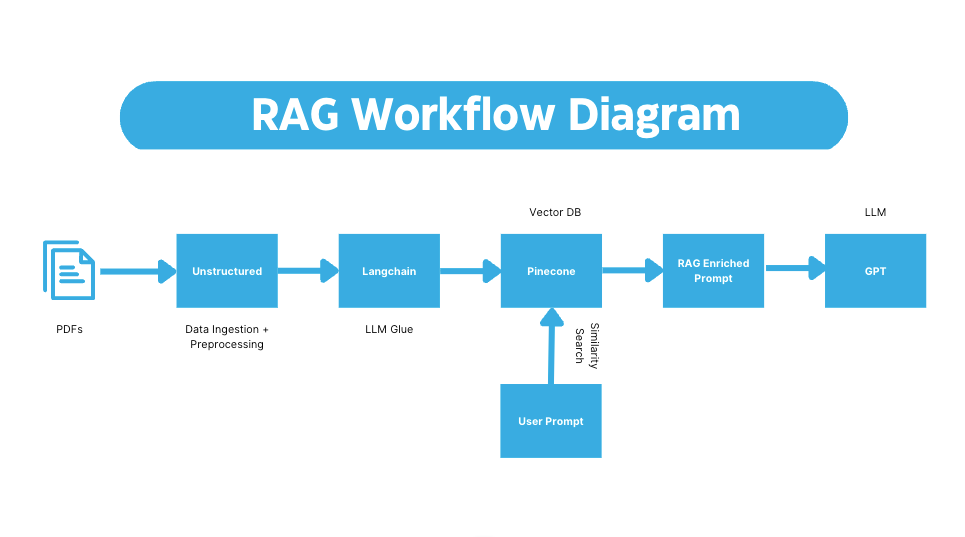
Supervised Fine-Tuning for Higher Accuracy
Supervised Fine-tuning (SFT) involves providing additional question-answer pairs to optimize the performance of LLMs. This fine-tuning can be used for updating the LLM’s internal knowledge, or for task-specific training like text summarization, or for translating natural language to database queries. Fine-tuning approaches help mitigate hallucinations in LLMs but cannot eliminate them completely.
SFT differs from generic fine-tuning in that it focuses on aligning language models to emulate a correct style or behavior rather than solving a particular task. This distinction ensures that the model retains its generic problem-solving capabilities while improving its performance in specific areas. Creating a high-quality dataset for SFT is crucial as the results heavily depend on the diversity and accuracy of the examples in the dataset.
While SFT alone can yield significant improvements, it is often combined with other methods like Reinforcement Learning from Human Feedback (RLHF) for better results. RLHF involves training the model to optimize a reward function based on human feedback, further enhancing the model's alignment with desired outcomes. The combination of SFT and RLHF has proven to be effective in improving the quality and safety of language models, as demonstrated in recent models like Llama-2.
In practical applications, SFT is implemented using tools like the transformer reinforcement learning (TRL) library, which facilitates the fine-tuning process with a few lines of code. This approach is popular in open-source LLM research, showcasing its ease of use and effectiveness. Advanced techniques in SFT, such as parameter-efficient fine-tuning approaches like Low-Rank Adaptation (LoRA), allow for fine-tuning a small part of the model, making it a resource-efficient process suitable for models with billions of parameters.
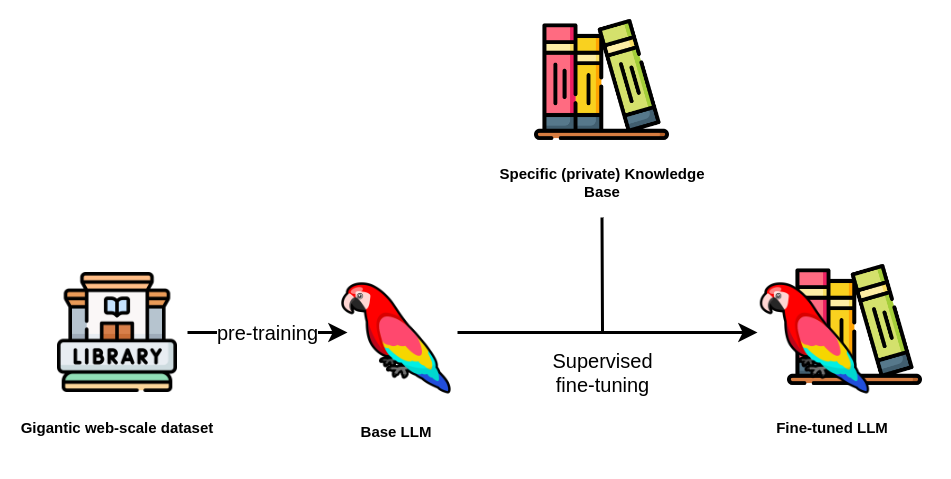
MLOps - The DevOps of Machine Learning
MLOps, short for Machine Learning Operations, is a rapidly evolving discipline that blends machine learning, DevOps, and data engineering. It focuses on the reliable and efficient deployment and maintenance of machine learning models in production environments. The main goal of MLOps is to streamline the entire lifecycle of machine learning models – from integration with model generation, orchestration, and deployment, to diagnostics, governance, and business metrics. This practice is crucial for organizations as it ensures the consistent quality of production models while addressing business and regulatory requirements.
The need for MLOps arises from the unique challenges presented by machine learning models compared to traditional software development. Machine learning models involve complex processes such as data collection, model training, validation, deployment, and continuous monitoring and retraining. MLOps is critical to managing the release of new ML models systematically and simultaneously with application code and data changes. This approach treats ML assets similarly to other software assets in continuous integration and delivery (CI/CD) environments. An optimal MLOps implementation deploys ML models alongside the applications and services they use and those that consume them as part of a unified release process.

Source: Ubuntu Blog
Potential of AI integration into SaaS
So, how does AI enhance the classic SaaS stack?
As we saw in the section on Generative AI, AI models are now capable of generating natural language text, images, video, audio and speech. This potentially changes the very nature of SaaS applications, giving it superpowers that it did not have before.
We envision that AI would form an additional piece, the brain, in the future SaaS stack. Along with frontend, backend, and databases, AI technologies combined would serve as the central intelligence of SaaS applications.
AI’s cognitive capabilities enable applications to learn, adapt, and automate tasks intelligently. It transforms the user-experience, enabling more natural modes of interaction. Let’s first explore the possibilities.
- Enhanced User Experience
- Natural Language Interfaces: AI-driven NLP enhances user interactions, enabling applications to understand and respond to human language. This is particularly useful in chatbots, virtual assistants, and customer support applications.
- Personalized Experiences: AI analyzes user behavior to offer personalized experiences. SaaS applications leverage AI to tailor content, recommendations, and user interfaces based on individual preferences, boosting user engagement.
- Operational Efficiency
- Understanding Data: ML algorithms in SaaS applications analyze large datasets to identify patterns and trends. This is invaluable for optimizing workflows, predicting user behavior, and automating routine tasks.
- Predictive Analytics: AI-powered predictive analytics provides insights into future trends, helping businesses make informed decisions. In SaaS, this can be applied to customer retention, sales forecasting, and resource optimization.
- Advanced Automation
- AI-Driven Automation: SaaS applications integrate AI to automate complex tasks. From automating customer support processes to optimizing data analysis, AI-driven automation enhances efficiency, reduces manual effort, and minimizes errors.
- Robotic Process Automation (RPA): AI-powered bots mimic human actions, automating repetitive tasks within SaaS applications. This not only accelerates processes but also allows human resources to focus on more strategic activities.
- Cybersecurity Reinforcement
- Threat Detection: AI analyzes vast datasets to identify patterns indicative of potential cyber threats. In SaaS, this is crucial for bolstering cybersecurity measures, protecting sensitive data, and ensuring a secure user environment.
- Incident Response: AI's ability to swiftly respond to security incidents minimizes the impact of breaches. SaaS applications equipped with AI-driven incident response mechanisms can detect and mitigate threats in real-time.
- AI-Integrated CRM
AI transforms CRM by providing deep insights into customer behavior. SaaS applications integrated with AI-driven CRM solutions offer personalized customer interactions, optimized sales strategies, and improved overall customer service.
Opportunities for Startups in AI-Integrated SaaS
The intersection of Artificial Intelligence (AI) and Software as a Service (SaaS) presents a fertile ground for startups to innovate, disrupt, and carve a niche in the tech landscape. Here are key opportunities for startups venturing into AI SaaS:
- AI-Driven Vertical Solutions: Startups can focus on developing AI-driven SaaS solutions tailored for specific industries or verticals. By addressing niche challenges with AI-powered insights and automation, startups can establish themselves as specialized providers.
- Automation for Small Businesses: Small and medium-sized enterprises (SMEs) often lack resources for complex software solutions. Startups can seize the opportunity to develop AI-driven automation tools within SaaS platforms, enabling SMEs to enhance efficiency without a significant investment.
- AI-Powered Customer Support: Startups can explore AI applications in customer support within SaaS platforms. Chatbots, virtual assistants, and automated ticketing systems can revolutionize how businesses interact with their customers, offering cost-effective solutions for startups.
- Integration Solutions: Developing AI-driven integration solutions that seamlessly connect existing SaaS applications can be a lucrative opportunity. Startups can address the challenge of interoperability, providing businesses with unified AI-enhanced workflows.
- AI-Enhanced Analytics: Startups can focus on building AI-powered analytics tools embedded in SaaS platforms. Providing users with advanced data analysis, predictive insights, and actionable recommendations can set a startup apart in the competitive SaaS landscape.
- Personalization at Scale: AI enables startups to deliver highly personalized experiences within SaaS platforms. By understanding user behavior and preferences, startups can offer tailored content, recommendations, and interfaces, enhancing user engagement.
- Predictive Analytics for Decision-Making: Startups can leverage AI to offer predictive analytics within SaaS platforms. This enables businesses to make data-driven decisions, forecast trends, and identify opportunities for growth, setting the stage for more informed strategies.
How E2E Networks Helps SaaS Startups
E2E Networks is bullish about the future of AI-powered SaaS and believes that AI has the potential to transform a number of industries. They believe that in the near future, we will see interfaces that are more natural, effective and highly efficient, the holy grail for most SaaS platforms.
To help enable this, E2E offers credits to all startups who are looking to deep dive into AI. These credits can be used to test, train and deploy advanced AI models on their GPU nodes or their proprietary AI platform, TIR. E2E is also working closely with Indian entrepreneurs through workshops, hackathons, and mentorship programs that help them understand the rapidly evolving landscape of generative AI.
Write to E2E if you are interested in using AI in your SaaS stack, and the E2E team will guide you through the various programs they have in place.
Conclusion
SaaS, with its inherent advantages, stands as a resilient and adaptive model for software delivery. The infusion of AI into SaaS applications amplifies these advantages, pushing the boundaries of what's possible in terms of user experience, operational efficiency, and cybersecurity. As businesses navigate the digital landscape, the symbiosis of SaaS and AI is poised to define the next era of technological evolution.
The integration of NLP, ML, Predictive Analytics, AI-Driven Automation, and AI-Powered Cybersecurity into SaaS represents a paradigm shift. These technologies collectively elevate user experience, optimize operations, and fortify the security of SaaS businesses. As AI continues to advance, its impact on SaaS promises continued innovation and a robust foundation for the future of digital services.
To know more, write to sales@e2enetworks.com.