Introduction
Robotics has traditionally operated within a realm of specialization. Each robot, whether used in an industrial setting or for service-oriented tasks, is specifically designed and programmed for its intended function. However, as we move further into the era of advanced technology, there's an increasing demand for robots that can handle a wider variety of tasks without requiring specialized models for each one1.
The challenge lies in the fact that, historically, a unique model is trained and tailored to each specific robot and its environment or task. Altering any parameter often means retraining the model from scratch, a procedure that's not only resource-intensive but also inefficient. This is the problem that the RT-X initiative aims to address.
The core vision of RT-X is not just the development of a universally adaptable robot. It's about synthesizing knowledge from the entire robotic ecosystem into a unified, scalable model. Through collaborative efforts spanning 33 academic laboratories and incorporating data from 22 distinct robot types, the RT-X project aspires to transform this vision into a practical solution for the future of robotics.
Scaling Up Learning through Open X-Embodiment Repository
With the rapidly advancing pace of technology, robots have found applications in various sectors, from industrial automation to healthcare and domestic chores. However, a significant limitation of most robotics systems lies in their specialization. Each robot, fine-tuned for a specific task, often struggles to adapt to new challenges outside its trained domain. Such narrow specialization inherently restricts the versatility and applicability of robotic solutions.
In light of this, the aspiration to train a robot that can function across lots of tasks, environments, and conditions emerges as a logical next step. The answer lies in pooling collective robotic knowledge. The field of robotics has long been dominated by proprietary systems and isolated datasets. To break this mold and promote a more collaborative approach to robotics research, the Open X-Embodiment Repository was introduced. This open-source repository provides a centralized platform where researchers and developers can access a wealth of data and resources related to diverse robotic platforms.
Central to this repository is the Open X-Embodiment Dataset. This dataset stands out due to its vastness, encompassing over 1 million robot trajectories from 22 different robot types. By consolidating data from 60 existing robot datasets, sourced from 34 leading robotic research labs globally, the Open X-Embodiment Dataset offers an unparalleled breadth of robotic knowledge and actions. To ensure the data remains accessible and user-friendly, it's formatted using the RLDS data format, making it compatible with most deep learning frameworks.
Furthermore, the repository offers pre-trained model checkpoints. These checkpoints, specifically the RT-X model, are designed for researchers to build upon, offering a foundation for further exploration and adaptation.In essence, the Open X-Embodiment Repository isn't just a collection of data. It's a call to the global robotics community, inviting researchers to collaborate, innovate, and push the boundaries of what's possible in the realm of robotic learning.
By moving away from the paradigm of individualized training for each robot type and environment, the RT-X approach offers a promising solution. The ability to train a universal model that learns from multiple embodiments not only reduces the need for repetitive task-specific training but also enhances the adaptability and efficiency of robotic systems as a whole.
Foundations: RT-1 and RT-2 Models
Before diving into the nuances of RT-X, it's essential to understand the foundational models upon which it builds: RT-1 and RT-2.
RT-1: Designed with robotic control in mind, RT-1 operates on a Transformer architecture, a novel approach in machine learning. With a focus on real-world robotic control at scale, this model utilizes visual input and processes it through a pre-trained model like EfficientNet. Alongside visual data, natural language instructions guide the robot's actions, with these instructions transformed into embeddings. The culmination of these processes allows RT-1 to make informed decisions about robot actions in its environment2.
RT-2: Differing from RT-1, RT-2 is a vision-language-action (VLA) model. It merges visual and language data, casting robot actions as natural language tokens. The benefit? By translating actions to text tokens, RT-2 can tap into the vast domain of pre-trained vision-language models, enhancing its versatility. This model's unique approach means it can be fine-tuned for robotic control, making it adept at understanding and executing complex tasks based on visual and linguistic inputs3.
Potential of RT-X
Historically, each robot was designed with a specific purpose in mind. If a robot was built for industrial manufacturing, its abilities were limited to that environment. However, with RT-X, there's a shift in this paradigm. Instead of training individual models for each specific task or robot type, RT-X promotes the idea of a unified model that's equipped to handle a multitude of tasks across different robotic platforms.
But what makes RT-X stand out? The key is its ability to amalgamate data from diverse robotic platforms. Using the vast Open X-Embodiment Dataset, RT-X is trained across 22 different robot types. This cross-training means the model isn't just limited to the capabilities of one robot type but has insights from a myriad of platforms.
The initial results have been promising. For instance, the RT-1-X variant of the model displayed a significant improvement over traditional, robot-specific models. In evaluations, RT-1-X showed a 50% higher success rate compared to conventional methods. Similarly, the RT-2-X variant demonstrated impressive capabilities, especially in understanding spatial relationships and adapting to varied tasks.
In a nutshell, RT-X isn't just another robotics model. It signifies a step towards a future where robots are not bound by their specific design or initial training but can adapt, learn, and operate across a wide range of scenarios.
Evaluating Emergent Skills with RT-X
In robotics, adaptability remains one of the most sought-after capabilities. While traditional models have been adept at performing tasks they were explicitly trained for, the real challenge lies in their ability to exhibit emergent skills—those not explicitly taught during training but inferred from the data they've been exposed to. RT-X, in its innovative design, promises a step forward in this direction.
For RT-X, this evaluation was centered around its performance on tasks involving objects and skills that were unfamiliar. For instance, while the Google Robot might have extensive training data from the RT-2 dataset, certain tasks were difficult for it. Yet, when RT-2-X, a variant of RT-X, was put to the test, it showcased a profound ability to adapt and perform. In fact, in comparative evaluations, RT-2-X demonstrated a whopping threefold improvement over RT-2 in emergent skills.
The true strength of RT-X's emergent skill capabilities was further underscored through a series of ablation studies:
Training Diversity: When RT-2-X was trained excluding the Bridge dataset, its performance on hold-out tasks saw a significant dip. This pointed to a vital insight—the diversity of data from various robots played a pivotal role in enhancing emergent skills.
Decoding Design Decisions: Various design elements of RT-2-X were considered including factors like image history, web-based pre-training, and model size. Larger models, such as the 55B variant, displayed a superior ability to transfer knowledge across robotic datasets compared to their smaller counterparts.
Spatial Understanding: One of the standout features of RT-2-X is its advanced spatial understanding. The model could discern subtle linguistic nuances, like the difference between move apple near cloth and move apple on cloth, and modify its actions accordingly. Such an ability underscores the model's potential in understanding and adapting to real-world scenarios.
Results and Findings
The RT-X model, with its unique design considerations and vast training data, has brought forth some compelling results that set new benchmarks in robotics. Here's a breakdown of its primary achievements:
RT-1-X: In comparative evaluations, RT-1-X showcased an impressive 50% improvement over the existing state-of-the-art methods. This significant boost in performance underscores RT-1-X's advanced learning capabilities and adaptability.
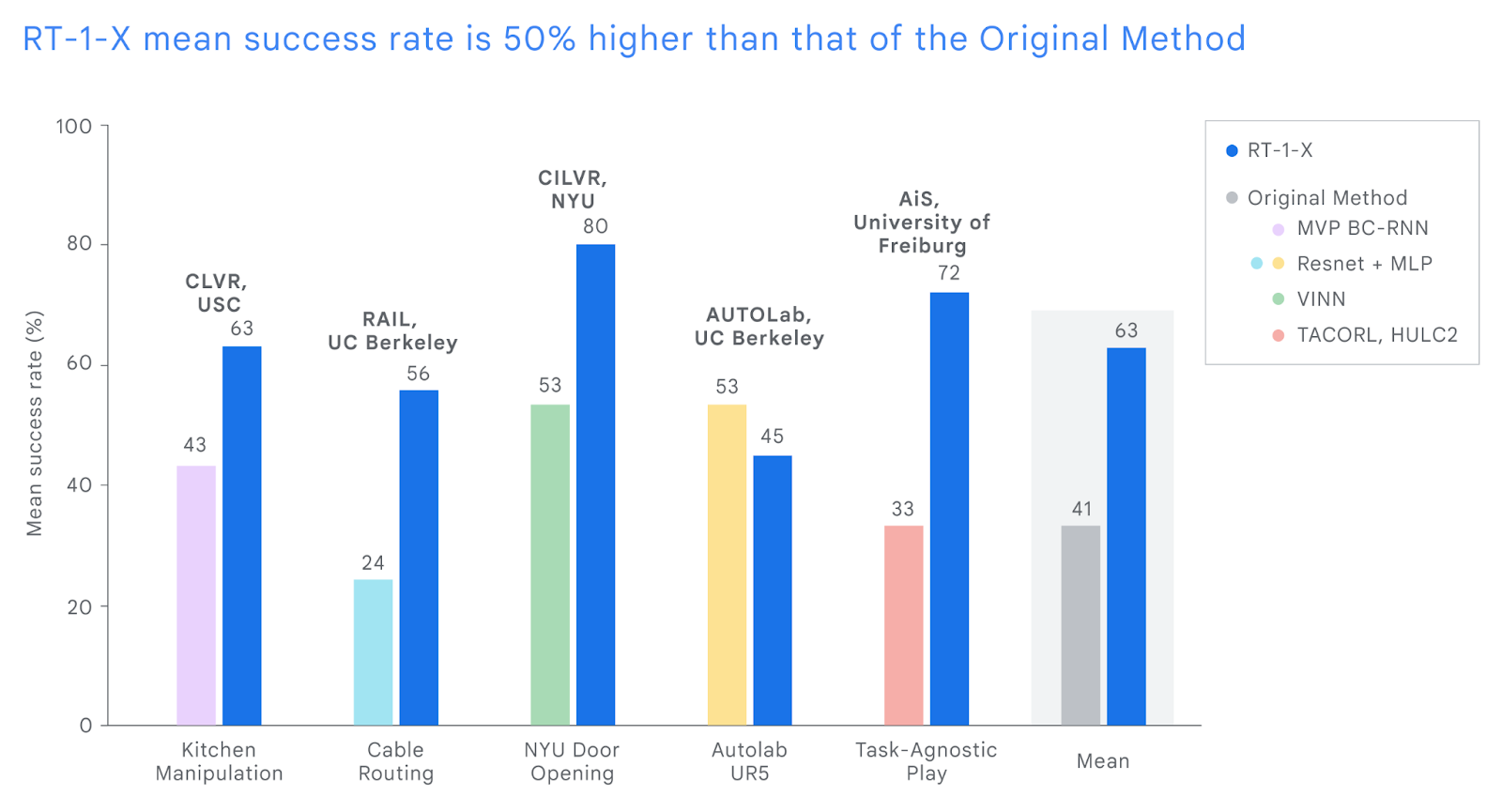
RT-2-X: An equally notable achievement was the ability of RT-2-X to generalize across different robotic tasks. When pitted against models that were trained only on data from specific robotic embodiments, RT-2-X exhibited a threefold improvement in generalization capabilities. This makes it a formidable tool in diverse robotic environments, highlighting its versatility.
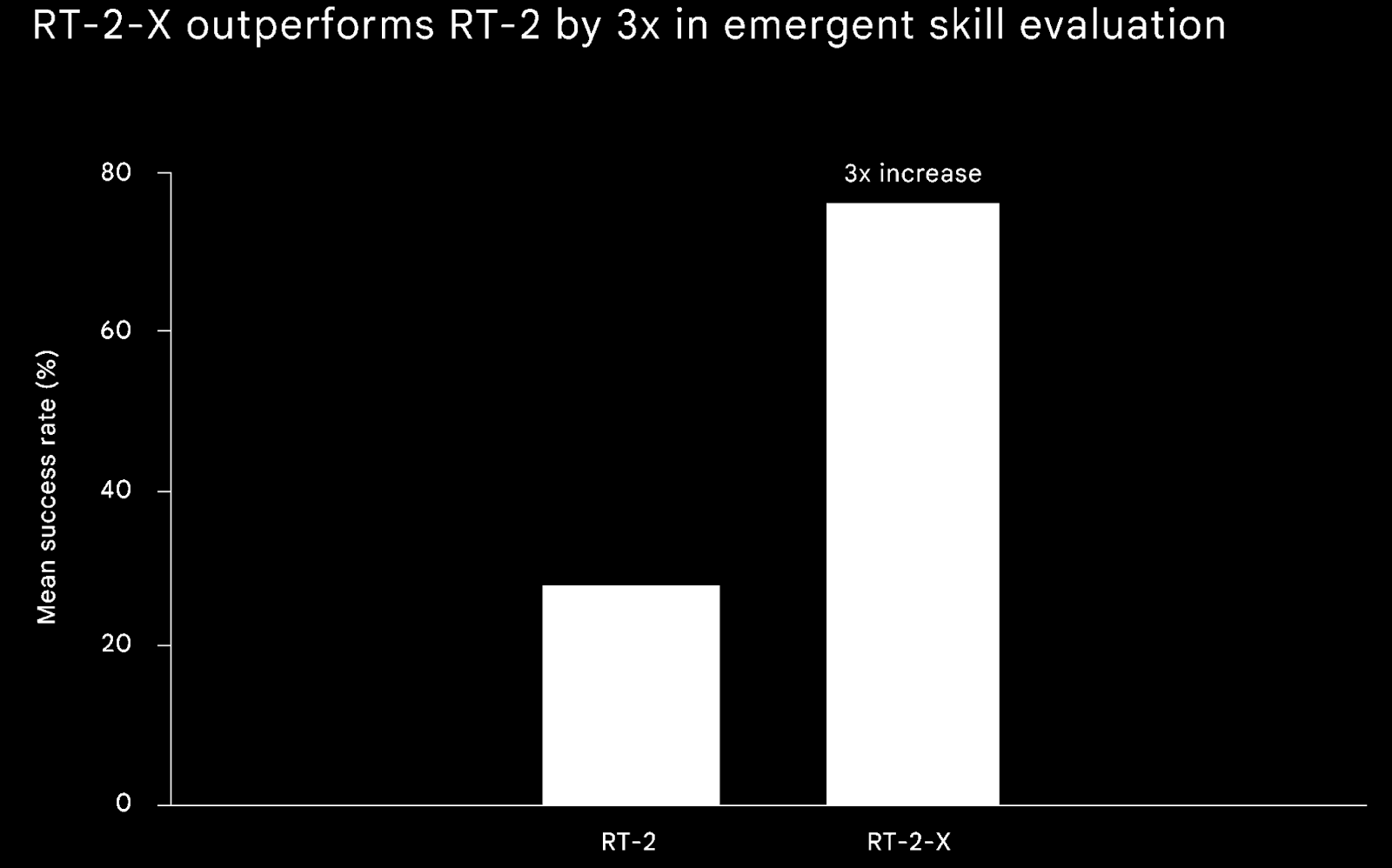
Conclusion
It is essential to introspect, identify current limitations, and chart the way forward.
Current Limitations of RT-X
Like any pioneering technology, RT-X isn't devoid of challenges. While it excels in many domains, there are scenarios where its adaptability might be constrained. For instance, it doesn't always consider robots with vastly different sensing and actuation modalities. Also, the model hasn't been fully tested for its generalization to entirely new robots, leaving room for future enhancements.
Future Prospects and the Potential of X-Robot Learning
The horizon looks promising for X-robot learning. RT-X has laid a robust foundation, and the potential of a truly X-embodied robot generalist is within sight. As datasets grow richer and more diverse, and as models become even more sophisticated, the capabilities of such systems are only set to expand. The dream is a future where robots can seamlessly adapt, learn, and perform tasks beyond their initial programming.
While RT-X has broken new ground, the journey of exploration is far from over. To truly realize the dream of universally adaptable robots, there's a pressing need for more research. This encompasses studying how positive transfer can be better optimized, understanding the intricacies of cross-embodiment generalization, and refining models to be even more responsive to diverse robotic tasks.
References
1. Chebotar, Y. & Yu, T. RT-2: ‘New Model Translates Vision and Language into Action.’ DeepMind https://www.deepmind.com/blog/rt-2-new-model-translates-vision-and-language-into-action (2023).
2. Brohan, A. et al. ‘RT-1: Robotics Transformer for Real-World Control at Scale.’ (2022).
3. Brohan, A. et al. ‘RT-2: Vision-Language-Action Models Transfer Web Knowledge to Robotic Control.’ (2023).