Introduction
Language models have advanced tremendously in the field of natural language processing, enticing people with their capacity to produce text that resembles human speech and understand complex distinctions between languages. Large language models have made revolutionary innovations in the domain of AI, enhancing various applications like language translation, content generation and chatbots [1]. These increasing advancements in models makes it challenging for them to deal with LLMs during their training and thus there is a need to enhance the efficiency and speed of the system. Researchers have a broad range of opportunity with regulating and improving attention mechanisms. Pay attention and keep reading for a bit longer to advance yourself in the world of transformers.
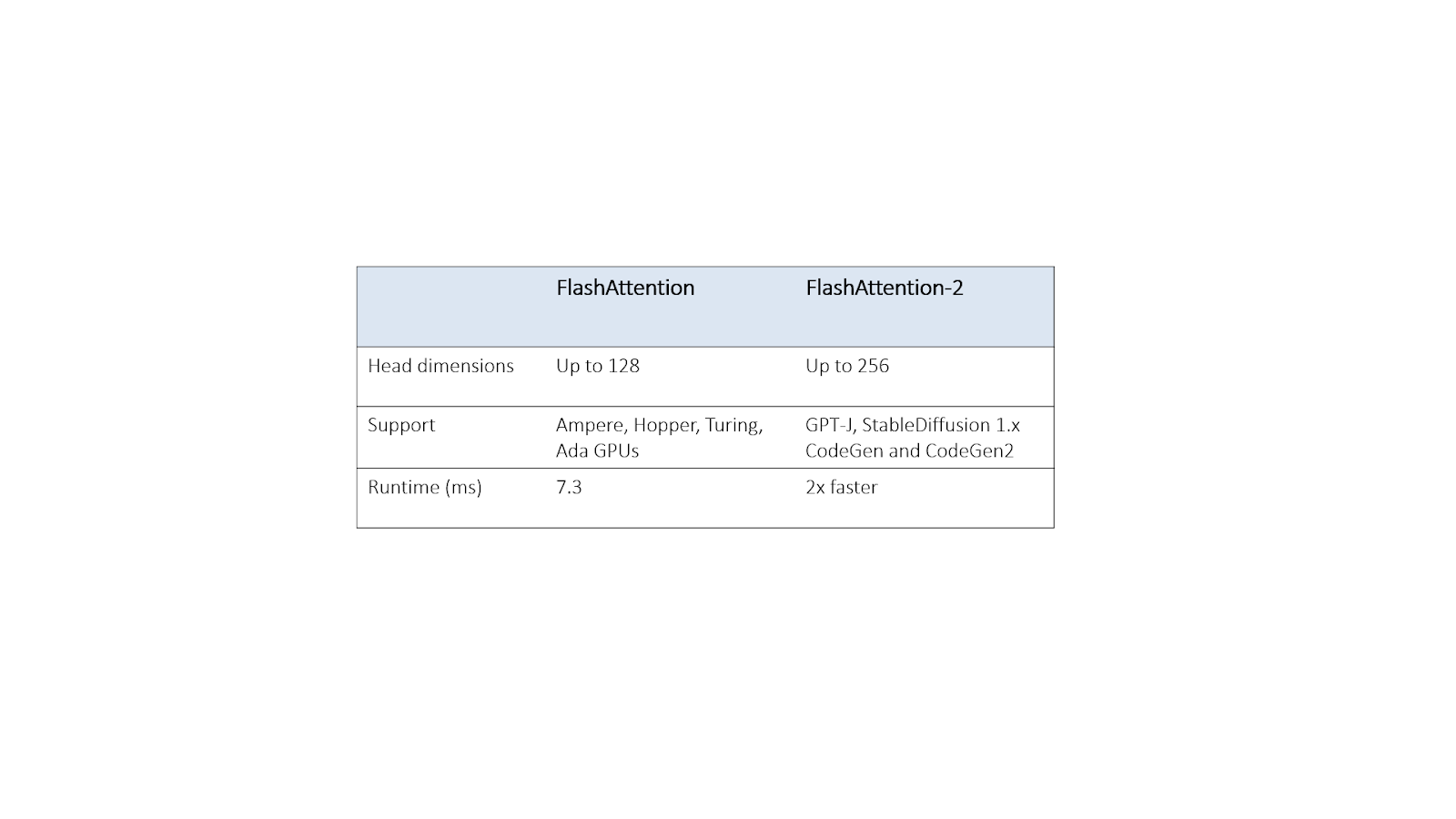
Transformers struggle with lengthy sequences such as full paragraphs or articles, because of following two reasons:
- They have extremely high computational requirements.
- The time and memory demands of self-attention scale quadratically (quadratic complexity) with sequence length.

Understanding ‘Attention’ Mechanism
The complex architecture of neural networks in deep learning models makes it difficult for the neural networks to pass the relevant information to the next layers. To address this limitation in models, an attention mechanism was discovered.
Let us understand this with an example:
Consider an LLM which is created for language translation. And a complete huge paragraph is given to the model for language translation. But the model might find it challenging to remember the long sequences of words in the paragraph. At such times, ‘attention’ mechanism memorises the context of each word in the sentence and assigns each word its attention weight with respect to all the other words in the sentence. This enhances the performance of the model and helps in memorising the context and meaning of the sentence.
Quadratic Complexity - a Challenge?
In self-attention, each component of the sequence serves as both a query and a key, causing all components to focus on one another. This increases the number of computations quadratically.
Wall clock speedup is frequently used to assess the degree of improvements or progress in hardware or software. It becomes essential for models to achieve the wall clock speedup, in order to optimize the complexity of the model. Another challenge is the absence of IO awareness, which is responsible for a significant number of reads and writes between GPU memory levels.
Need of a Modern Mechanism: FlashAttention

In 2022, FlashAttention was introduced as an advancement to vanilla attention by researchers at Stanford University [2]. It leverages the IO-awareness and brings forth the fast and memory-efficient ‘exact attention’. However, many strategies had been proposed that resembled self-attention mechanisms, but they either perform slowly or don't speed up the wall clock at all.
FlashAttention reframes the computation of attention to accomplish remarkable wall clock speedups and enhance memory efficiency. It utilizes the IO-awareness approach that uses input-output (IO) properties to reduce the computation time of attention from quadratic to linear complexity and minimize memory consumption. As described in Fig. 2, FlashAttention aims to achieve better training speedup and memorise lengthy sequences.
Did FlashAttention Benefit LLMs?
Recently, FlashAttention made a groundbreaking performance when it was incorporated by Falcon-40B. Falcon-40B is a significant development in the sphere of large language models (LLMs). It speeds up 3x faster on GPT-2 (sequence length 1k) and 2.4x faster on lengthy arena (sequence length 1k – 4k). Additionally, inference processing on Falcon-40B is 5X faster than on GPT-3.
Since FlashAttention is 2-4x faster than vanilla implementation, it has been incorporated by several organizations, including by various transformer-based applications. For example, FlashAttention was used by many open-source LLMs resulting in faster inference, lower memory consumption and speed up during pre-training of models. When compared to Hugging Face implementation and Megatron-LM, GPT-2 small and medium resulted up to 3x and 1.7x faster, respectively. For example, kernel provides up to 12x faster transformers inference and has a speedup over the Hugging Face baseline.
How is FlashAttention improving the inference time and speeding up the training? Let’s dive into this and examine various enhancements as illustrated in Fig. 3 below.

The graph in Fig. 4 below denotes the memory savings which are directly proportional to the sequence length – as standard attention has quadratic memory sequence length and FlashAttention has linear memory sequence length [2]. Sequence lengths of 2K and 4K result in memory reductions of 10X and 20X, respectively.
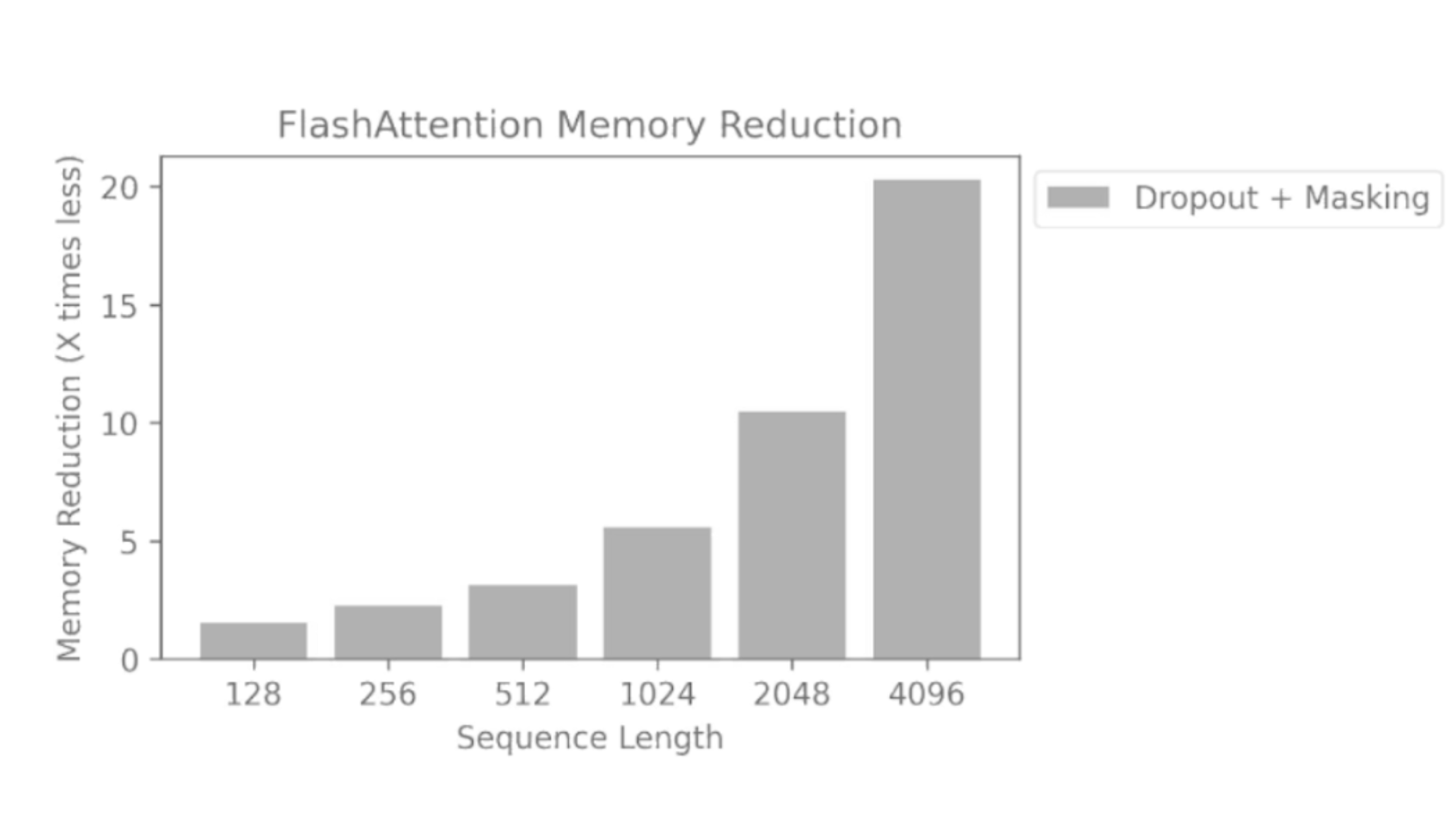
Futuristic Advancement: FlashAttention-2
FlashAttention-2 has been newly developed as an advanced version of FlashAttention. It is 2x faster than FlashAttention and 5-9x faster than the standard attention, hitting training speed of 225 TFLOPs/s on the A100 [3]. It enables us to train models with 16k long sequences (context) for the same cost as we could have originally trained a model with 8k context.

FlashAttention-2 introduces two new features mentioned below:
- Head dimensions up to 256
FlashAttention-2 supports head dimensions up to 256, whereas FlashAttention-v1 supports up to 128 head dimensions. This enables models like StableDiffusion 1.x, GPT-J, CodeGen and CodeGen2 to use FlashAttention-2 to achieve speedup and optimize memory.
- Multi-query attention and grouped-query attention
These attentional variations minimize the size of the KV cache during inference and can result in a noticeably improved performance for inference by having several heads of query interacting with a single head of key and value.
Fewer Non-Matmul FLOPs
FlashAttention-2 minimizes the number of non-matmul FLOPs by tweaking the FlashAttention algorithm. A different approach to it is that each non-matmul FLOP costs 16 times as much as a matmul FLOP. We aim to spend as much time as possible on matmul FLOPs to maintain high throughput.
Better Parallelism
FlashAttention supports batch size and headcount parallelization. To process one attention head, one thread block is required, and altogether it has (batch_size * number of heads) thread blocks. It is effective only when this number is high as most of the computational resources on GPU can be utilized at maximum efficiency. To effectively utilize the multiprocessors on the GPU, they are further parallelized over the sequence length dimension in the context of long sequences (which typically requires small batch sizes or few heads). This leads to significant speedup.
Better Work Partitioning
It's crucial to divide the work among different wraps (32-threads processing together) within each thread block. This division process is enhanced using FlashAttention-2 to minimize interaction and synchronization among the wraps, leading to reduced read/write operations to shared memory. Speed is boosted by reducing read/write operations on shared memory.
Future Scope
Using FlashAttention-2 will boost up the speed of the training, finetuning, and inference of current models. It can be used to understand extensive manuals and reports, high-resolution visuals, audio data, and video content. The development team intends to work with people to make FlashAttention broadly usable on a variety of devices (like H100 GPUs and AMD GPUs as well as new data formats like FP8) in the near future. The immediate next step is to optimize FlashAttention-2 for H100 GPUs in order to take advantage of the new hardware features.
Conclusion
FlashAttention reflects a revolutionary improvement in attention mechanisms of transformers. It makes it possible to improve performance while also significantly reducing computing expenses. This advancement enables models to quickly process lengthy sequences, enhancing their scalability and versatility. To a great extent, the future of FlashAttention resides in its capacity for creativity and in its ability to propel improvements in attention mechanisms, making them more productive, profitable and interpretable for a variety of applications.
References:
- Zhang, C., Bauer, S., Bennett, P., Gao, J., Gong, W., Hilmkil, A., ... & Vaughan, J., ‘Understanding Causality with Large Language Models: Feasibility and Opportunities’, arXiv preprint arXiv:2304.05524, 2023.
- Dao, T., Fu, D., Ermon, S., Rudra, A., & Ré, C., ‘FlashAttention: Fast and Memory-Efficient Exact Attention with IO-awareness’, Advances in Neural Information Processing Systems, 35, 16344-16359, 2022.
- Dao, T., ‘FlashAttention-2: Faster Attention with Better Parallelism and Work Partitioning’, arXiv preprint arXiv:2307.08691, 2023.