Knowledge Graphs (KGs) are structured representations of knowledge that organize information in the form of queryable graphs. In a knowledge graph, entities such as people, places, things, and concepts are represented as nodes, while relationships between these entities are depicted as edges. Knowledge graphs are particularly valuable for reasoning over complex data.
Thanks to their inherent reasoning capabilities, Knowledge Graphs have become crucial in the world of AI, especially for building systems that require modeling complex relationships within data. They are particularly beneficial in building Retrieval-Augmented Generation (RAG) applications, where, instead of relying solely on vector databases, knowledge graphs are used to index and reason over documents, creating a richer context for large language models (LLMs).
In this step-by-step guide, we'll explore how to create a RAG application using LangChain, integrating knowledge graphs to enhance data retrieval and generation capabilities.
Understanding Knowledge Graphs
Knowledge graphs are structured representations of information that organize data into entities and their relationships, forming a network of interconnected knowledge. This allows for a more natural understanding of how different pieces of information relate to each other, similar to how humans connect concepts.

These graphs are widely used in applications such as search engines, recommendation systems, and natural language processing, as their structured approach to modeling information enhances the accuracy and relevance of results.
Key Components of a Knowledge Graph
- Entities (Nodes): These are the objects or concepts in a knowledge graph, such as "Albert Einstein," "Physics," or "Theory of Relativity."
- Relationships (Edges): These connect the entities and define how they are related. For example, an edge could represent the relationship "invented by" between "Theory of Relativity" and "Albert Einstein."
- Attributes: These are properties or characteristics of entities. For instance, the entity "Albert Einstein" might have attributes like "date of birth" and "occupation."
- Ontology: This is a schema that defines the types of entities and relationships in the graph, ensuring consistency in how knowledge is represented.
Knowledge graphs are typically stored and queried using Cypher queries. One key aspect of the Cypher query language is that it is highly readable and explainable, and can be easily understood by both machines and humans. They can also be visualized easily, making them useful for creating explainable AI systems.
The best way to see this is through an example Cypher query around Albert Einstein.
Below, we will first create ‘nodes’ in a knowledge graph using Cypher queries:
// Create nodes for Albert Einstein and related entities
CREATE (einstein:Person {name: "Albert Einstein", birthDate: "1879-03-14", placeOfBirth: "Ulm, Germany", occupation: "Theoretical Physicist", nationality: ["German", "Swiss", "American"]})
CREATE (relativity:Theory {name: "Theory of Relativity"})
CREATE (nobel:Award {name: "Nobel Prize in Physics", year: 1921})
CREATE (mileva:Person {name: "Mileva Marić"})
CREATE (princeton:Institution {name: "Princeton University"})
CREATE (photoelectric:Concept {name: "Photoelectric Effect"})
CREATE (newton:Person {name: "Isaac Newton"})
Next we will create relationships between the nodes:
// Create relationships between Albert Einstein and related entities
CREATE (einstein)-[:DEVELOPED]->(relativity)
CREATE (einstein)-[:AWARDED {year: 1921}]->(nobel)
CREATE (einstein)-[:SPOUSE]->(mileva)
CREATE (einstein)-[:WORKED_AT]->(princeton)
CREATE (einstein)-[:CONTRIBUTED_TO]->(photoelectric)
CREATE (einstein)-[:INFLUENCED_BY]->(newton)
This will result in a graph that looks like the one below:
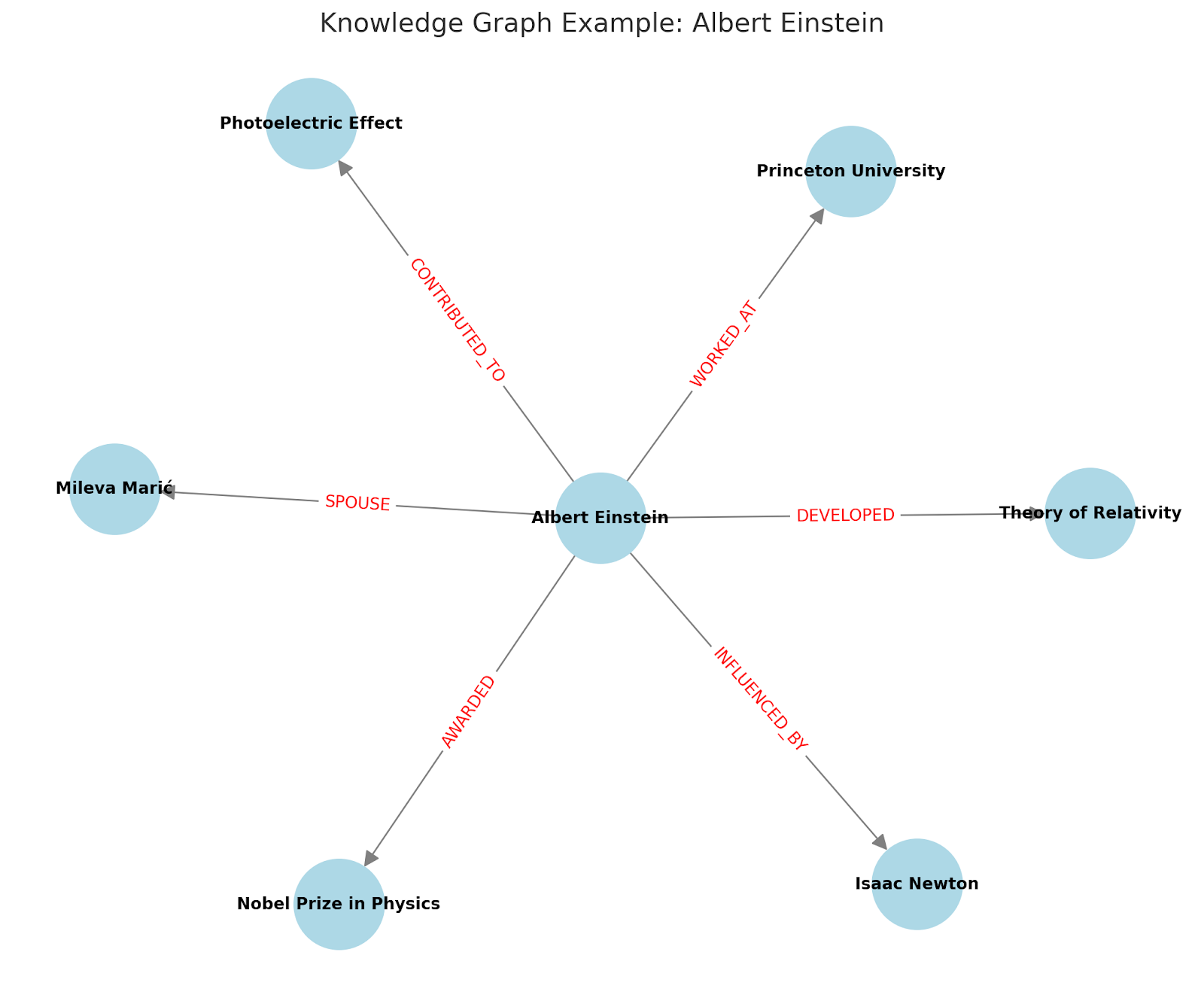
This shows the nodes and the relationships. Note that the attributes associated with each node haven't been visualized here.
How Do Knowledge Graphs Help in RAG
Retrieval-Augmented Generation (RAG) is a technique that combines information retrieval with generative models to produce more accurate and contextually relevant outputs.
In a RAG setup, a large language model (LLM) is paired with an information retrieval system that searches a database or document repository for relevant context or knowledge. This retrieved information is then prompted as context to the LLM, which it uses to generate its response. This approach ensures that the output is not only coherent but also grounded in factual and contextually appropriate information, even on data that might not have been part of the LLM’s training dataset. RAG systems, therefore, have emerged as a powerful tactic to leverage an LLM’s capabilities for internal content or knowledge base of a company.
RAG systems commonly use vector databases for the retrieval of documents. Vector databases work by first converting data into vector embeddings—high-dimensional numerical representations of data—and then using them to perform similarity searches to retrieve relevant information.
However, a significant challenge with vector databases is that these vector embeddings are inherently abstract and difficult for humans to visualize or interpret. This lack of transparency can make it challenging to understand why certain documents were retrieved or how the relationships between different pieces of data were established.
Knowledge Graphs (KGs) offer a distinct advantage in this regard. Unlike vector databases, KGs explicitly model entities and the relationships between them in a more intuitive, graph-based structure that is easier for humans to visualize and understand. This structured representation allows for more semantically rich retrieval and reasoning, enabling the RAG system to not only retrieve relevant documents but also provide contextually meaningful relationships between entities.
By leveraging the reasoning capabilities of KGs, RAG systems can produce outputs that are not only more accurate and context-aware but also more transparent and easier to interpret, making them particularly valuable in complex domains where understanding the connections between concepts is crucial.
How to Build RAG Using Knowledge Graph
Now that we understand KG-RAG or GraphRAG conceptually, let’s explore the steps to create them.
To do this, we will use cloud GPU nodes on E2E Cloud. This will allow us to locally deploy the LLM and the knowledge graph, and then build a RAG application.
Prerequisites
First, sign up to Myaccount on E2E Cloud. Once that’s done, launch a cloud GPU node. If you are using a large LLM like Llama 3.1, use A100 or L4OS. Alternatively, pick a cloud GPU node that works for the LLM of your choosing. Also, add your SSH keys while launching the node.
Once that’s done, SSH into the node:
$ ssh root@
You should now create a user using adduser (or useradd) command.
$ adduser username
Also, give the user sudo permission using visudo.
$ visudo
Add the following line in the file:
username ALL=(ALL) NOPASSWD:ALL
Deploying Neo4j
We will now deploy Neo4j, which is a powerful graph database (and also includes vector handling capabilities). We will assume Debian distribution. If you are installing in another Linux distribution, follow the steps here.
Method 1 - Using Docker
You can use Docker to install Neo4j using the following command.
docker run \
--name neo4j \
-p 7474:7474 -p 7687:7687 \
-d \
-e NEO4J_AUTH=neo4j/password \
-e NEO4J_PLUGINS=\[\"apoc\"\] \
neo4j:latest
Method 2 - Using apt
You can also deploy using apt-get in the following way:
$ sudo add-apt-repository -y ppa:openjdk-r/ppa
$ sudo apt-get update
Now let’s add the repository to our apt list:
$ wget -O - https://debian.neo4j.com/neotechnology.gpg.key | sudo gpg --dearmor -o /etc/apt/keyrings/neotechnology.gpg
echo 'deb [signed-by=/etc/apt/keyrings/neotechnology.gpg] https://debian.neo4j.com stable latest' | sudo tee -a /etc/apt/sources.list.d/neo4j.list
sudo apt-get update
We can now find out which versions of Neo4j are available using the following command:
$ apt list -a neo4j
We can pick from the versions listed, and install in the following way:
$ sudo apt-get install neo4j=1:5.23.0
This will start the Neo4j graph database, which we will use to store the knowledge graph.
Let’s store the values in a new .env file, which we can use in our code later.
NEO4J_URI="YOUR_NEO4J_URL"
NEO4J_USERNAME="YOUR_NEO4J_USERNAME"
NEO4J_PASSWORD="YOUR_NEO4J_PASSWORD"
Installing Ollama and LLM
One of the easiest ways to create an LLM endpoint is through TIR. You can follow the steps here to do so.
However, here we will use Ollama to leverage the same cloud GPU node. Install Ollama like this:
$ curl -fsSL https://ollama.com/install.sh | sh
Then, you can pull and serve the LLM easily.
$ ollama pull llama3.1
$ ollama run llama3.1
We can now use the Llama 3.1 model as our LLM.
Installing the Dependencies
Create a workspace folder, and then create a Python virtual environment:
$ python3 -m venv .env
$ souce .env/bin/activate
Let’s install the dependencies.
$ pip install python-dotenv
$ pip install streamlit
$ pip install langchain
$ pip install langchain-community
$ pip install langchain-ollama
Importing Python Modules
Before getting into the code, let’s import all the libraries and modules that we need.
import os
import streamlit as st
from dotenv import load_dotenv
from langchain_community.graphs import Neo4jGraph
from langchain.chains import GraphCypherQAChain
from langchain_ollama.llms import OllamaLLM
load_dotenv()
Initiating Knowledge Graph and LLM
It's time to initiate the Neo4j knowledge graph and the LLM.
graph = Neo4jGraph(
url=os.getenv("NEO4J_URI"),
username=os.getenv("NEO4J_USERNAME"),
password=os.getenv("NEO4J_PASSWORD"),
)
llm = OllamaLLM(model="llama3.1")
Creating the Knowledge Graph
As a demonstration of the approach, we will use a CSV. Here are the first 6 rows.

You can download the full CSV here.
To insert the data into the Neo4j database, firstly load the nodes representing each entity (e.g., name, email, location) using Cypher queries.
Then, define the relationships between these entities (e.g.,LIVES_IN) to establish how they are connected within the graph.
Finally, execute the Cypher queries in Neo4j using the following code that parses the CSV, iterates through the rows, and creates the nodes.
users_query = """
LOAD CSV WITH HEADERS FROM 'https://raw.githubusercontent.com/vansh-khaneja/test5/main/amazon_prime_users.csv' AS row
MERGE (person:Person {name: COALESCE(row.`Name`, 'Unknown'), email: COALESCE(row.`Email Address`, 'Unknown Email')})
MERGE (location:Location {name: COALESCE(row.`Location`, 'Unknown Location')})
MERGE (plan:SubscriptionPlan {name: COALESCE(row.`Subscription Plan`, 'Unknown Plan')})
MERGE (deviceNode:Device {name: COALESCE(row.`Devices Used`, 'Unknown Device')})
MERGE (person)-[:USES]->(deviceNode)
MERGE (person)-[:LIVES_IN]->(location)
MERGE (person)-[:SUBSCRIBED_TO]->(plan)
MERGE (person)-[:HAS_USER_ID]->(:UserID {id: COALESCE(row.`User ID`, 'Unknown ID')})
MERGE (person)-[:HAS_USERNAME]->(:Username {name: COALESCE(row.`Username`, 'Unknown Username')})
MERGE (person)-[:HAS_BIRTH_DATE]->(:BirthDate {date: COALESCE(row.`Date of Birth`, 'Unknown Date')})
MERGE (person)-[:HAS_GENDER]->(:Gender {type: COALESCE(row.`Gender`, 'Unknown Gender')})
MERGE (person)-[:MEMBERSHIP_STARTED_ON]->(:MembershipStartDate {date: COALESCE(row.`Membership Start Date`, 'Unknown Start Date')})
MERGE (person)-[:MEMBERSHIP_ENDED_ON]->(:MembershipEndDate {date: COALESCE(row.`Membership End Date`, 'Unknown End Date')})
MERGE (person)-[:HAS_PAYMENT_INFO]->(:PaymentInformation {info: COALESCE(row.`Payment Information`, 'Unknown Payment Info')})
MERGE (person)-[:HAS_RENEWAL_STATUS]->(:RenewalStatus {status: COALESCE(row.`Renewal Status`, 'Unknown Status')})
MERGE (person)-[:HAS_USAGE_FREQUENCY]->(:UsageFrequency {frequency: COALESCE(row.`Usage Frequency`, 'Unknown Frequency')})
MERGE (person)-[:HAS_PURCHASE_HISTORY]->(:PurchaseHistory {history: COALESCE(row.`Purchase History`, 'Unknown History')})
MERGE (person)-[:HAS_FAVORITE_GENRES]->(:FavoriteGenres {genres: COALESCE(row.`Favorite Genres`, 'Unknown Genres')})
MERGE (person)-[:HAS_ENGAGEMENT_METRICS]->(:EngagementMetrics {metrics: COALESCE(row.`Engagement Metrics`, 'Unknown Metrics')})
MERGE (person)-[:GAVE_FEEDBACK]->(:Feedback {ratings: COALESCE(row.`Feedback/Ratings`, 'No Feedback')})
MERGE (person)-[:INTERACTED_WITH_SUPPORT]->(:CustomerSupport {interactions: COALESCE(row.`Customer Support Interactions`, 'No Interactions')})
"""
graph.query(users_query)
This will create a knowledge graph with different relations based on the entity and relations provided above in the Cypher query. You can visualize it as below:
Graph of All the User Data

Graph of annual/monthly subscription purchased.

If you have a piece of unstructured text, you can also use an LLM to generate the Cypher queries to create the knowledge graph. Try it out!
Response Generation
Once the knowledge graph (KG) is created, we can develop a function that takes a user query as input and returns a response. This function will use a language model to generate a Cypher query, which fetches results from Neo4j. The language model then rephrases the answer based on the query and the context provided.
All of this is managed through a predefined chain provided by LangChain.
def response_generation(query):
chain = GraphCypherQAChain.from_llm(graph=graph, llm=llm, verbose=True)
answer = chain.run(query + f"return with proper naming conventions what you get as input rephrase it with this {user_query}")
return answer
User Interface
To make the app more interactive, we will be using Streamlit to create the frontend. Streamlit allows users to input queries, visualize, and interact with the Neo4j database through a simple Python-based web interface.
st.set_page_config(page_title="Knowledge Graph Chatbot", page_icon=":robot_face:")
st.markdown("""
Knowledge Graph Chatbot
""", unsafe_allow_html=True)
st.markdown("""
A RAG chatbot created with langchain and knowledge graphs using llama3.
""", unsafe_allow_html=True)
st.markdown("
", unsafe_allow_html=True)
user_query = st.text_input("Enter your question:", placeholder="E.g., Which devices is uesd by Alice to watch shows?")
if st.button("Ask"):
bot_response = response_generation(user_query)
st.markdown(f"""
Answer :
{bot_response}
""", unsafe_allow_html=True)
Output
This is the final view of our chatbot. When a user enters a query in the input box, the query is converted into a Cypher query, which is then executed against the knowledge graph to retrieve context. This context is passed to a language model, which generates a rephrased response based on the user’s query. Finally, the answer is displayed on the screen.
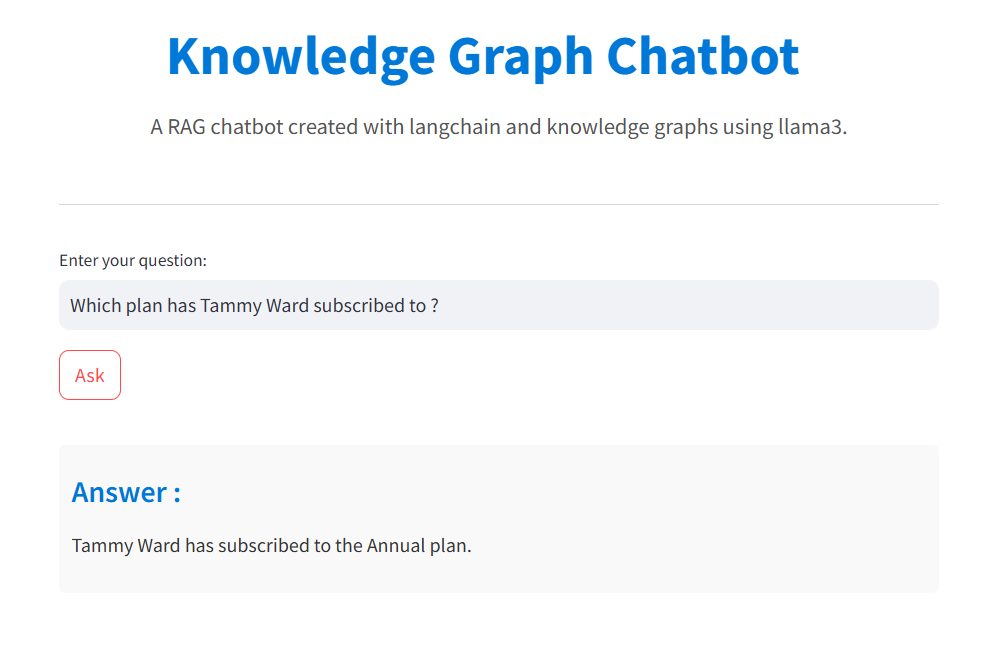
As you can see, the LLM responds back by leveraging the context data stored in the knowledge graph.
Conclusion
In conclusion, building a Retrieval-Augmented Generation (RAG) system using knowledge graphs and LangChain offers a powerful approach to enhance information retrieval and generate contextually relevant responses. By using the structured relationships in knowledge graphs and the capabilities of LangChain, you can create applications that not only retrieve information efficiently but also generate accurate and context-aware outputs.
This guide provides an overview of the steps involved, enabling you to implement RAG solutions that meet the demands of modern applications. With these modern techniques, the potential for innovation in natural language processing and data retrieval is vast, providing the way for more intelligent and interactive systems.
To get started with building knowledge graph-powered RAG applications using LLMs, sign up to E2E Cloud today.