Introduction
Deep learning has revolutionized the field of artificial intelligence, enabling machines to learn and make complex decisions like never before. As this technology continues to advance, it becomes crucial to have efficient tools that simplify the deep learning process, especially for advanced-level users. AutoKeras is an innovative and user-friendly automated machine learning (AutoML) framework designed specifically for deep learning. It aims to streamline the model development process, making it accessible to seasoned practitioners seeking to maximize their productivity and achieve exceptional results.
AutoKeras stands out for its remarkable adaptability, as it caters to various deep learning tasks, including image classification, text classification, and regression. It empowers users with little or no expertise in deep learning to effortlessly build and fine-tune sophisticated neural networks, automating several tedious tasks that otherwise demand extensive knowledge and time. This step-by-step guide will discuss the core functionalities of AutoKeras, unlocking its potential for advanced-level developers. The blog will explore AutoKeras' distinctive features, explain its significance in the realm of deep learning, and provide real-world use cases that demonstrate its practical applications.
Understanding AutoKeras
AutoKeras is an open-source Automated Machine Learning (AutoML) library mainly designed for deep learning tasks. It simplifies the complex process of designing and training deep neural networks, making it accessible to all levels of users. With AutoKeras, users can automate several key steps in the deep learning workflow, from data preprocessing to architecture search and hyperparameter tuning.
Key Features and Benefits
- User-Friendly Interface: AutoKeras provides an intuitive and user-friendly interface that allows users to create and train deep learning models with minimal effort. It eliminates the technical complexities, enabling users to focus on their specific tasks.
- AutoML for Deep Learning: AutoKeras automates the design and configuration of neural network architectures, which can be time-consuming and challenging, even for experienced deep learning practitioners. This automated approach saves valuable time and computational resources.
- Neural Architecture Search (NAS): One of the main features of AutoKeras is its NAS algorithm. It performs an efficient search in the neural architecture space to identify optimal architectures for a given dataset and task, resulting in models tailored to specific requirements.
- Complete Package: AutoKeras offers an end to end solution, covering all the workflows of deep learning, right from data preparation to model deployment. It easily integrates different stages, ensuring a smooth and efficient process.
- Customizable and Extensible: While AutoKeras automates many aspects of deep learning, it also provides users with the flexibility to customize and fine-tune their models. Users can modify model architectures, add custom layers, and define specific hyperparameters.
- Scalability: AutoKeras is designed to scale efficiently, making it suitable for both small-scale experiments and large-scale production systems.
Real-World Use Cases and Applications
- Image Classification: AutoKeras can be used in automating the design of convolutional neural networks (CNNs) for tasks like image classification, where it efficiently learns meaningful features from images.
- Text Classification: For tasks involving Natural Language Processing (NLP), AutoKeras can automatically create and optimize deep learning models, such as recurrent neural networks (RNNs) and transformers, for text classification.
- Regression: AutoKeras can be applied to regression tasks, such as predicting numeric values, by automatically generating appropriate neural network architectures.
- Transfer Learning: AutoKeras supports transfer learning, allowing users to use pre-trained models and adapt them to their specific tasks, reducing training time and resource requirements.
AutoKeras offers a powerful and efficient solution for automating deep learning tasks. Its user-friendly interface, neural architecture search capabilities, and flexibility make it an ideal choice for advanced-level users looking to use the benefits of AutoML in their deep learning projects.
Installation and Setup
To begin using AutoKeras for deep learning projects, users need to follow the installation and set-up process. AutoKeras is compatible with both Windows and Linux systems and supports Python 3.6 and higher. Below are the steps to install and set up AutoKeras:
Install AutoKeras: To install AutoKeras using pip, compiler must be open and the following command must be executed:
Install Dependencies: AutoKeras requires several dependencies to function correctly. It is recommended to create a virtual environment to avoid conflicts with other packages. If a user hasn't set up a virtual environment, they can do so using the following steps:
a. Install virtualenv
b. Create a new virtual environment
Windows
Linux
Install AutoKeras and Required Dependencies:
Once the virtual environment is activated, install AutoKeras and its dependencies:
To ensure that AutoKeras is installed correctly, run a quick test by importing it in a Python environment:
If there are no errors, AutoKeras is successfully installed.
Preparing the Deep Learning Environment
Before delving into the usage of AutoKeras, users must ensure they have a functional deep learning environment. This involves having Python, TensorFlow, or PyTorch (depending on the preferred framework), and other essential libraries like NumPy and Matplotlib installed. TensorFlow or PyTorch can be installed using the following command:
For TensorFlow
For Pytorch
GPU Support
For faster training, particularly with large datasets and complex models, it is advisable to consider using a GPU, although this is optional. The users must ensure that the required GPU drivers are installed, along with the corresponding GPU version of TensorFlow or PyTorch. With these steps completed, AutoKeras is successfully installed and the deep learning environment is set up. The following sections will walk through practical examples and applications of AutoKeras, enabling readers to deepen their understanding and proficiency in utilizing this advanced AutoML tool for deep learning.
Data Preparation
Data preparation is a crucial step in any machine learning project, including those with AutoKeras. Properly preparing the data ensures that the model can effectively learn from the dataset and make accurate predictions. Here are the key data preparation steps when using AutoKeras for deep learning:
- Data Collection: The first step is to gather the data relevant to the deep learning task. Depending on the project, this data could be images, text, audio, or any other type of data suitable for deep learning.
- Data Cleaning: Data cleaning involves handling missing values, removing duplicates, and addressing any inconsistencies in the data. Ensuring the quality and cleanliness of the data is essential for accurate model training.
- Data Formatting: AutoKeras requires the input data to be in specific formats depending on the type of deep learning task. For image classification, the images should be organized in separate folders for each class, while for text classification, the text data may need to be tokenized and encoded.
- Data Augmentation: Data augmentation involves generating additional training data by applying random transformations to the existing data. This technique can help increase the diversity of the training set, leading to improved model generalization.
- Data Normalization: Data normalization scales the numerical features to a common range (e.g., [0, 1] or [-1, 1]). Normalization helps prevent certain features from dominating the training process and ensures that the model converges faster.
- Data Splitting: Divide the dataset into training, validation, and testing sets. The training set is used to train the model, the validation set helps in tuning hyperparameters, and the testing set evaluates the final model performance. A common split ratio is 80% for training, 10% for validation, and 10% for testing.
- Data Loading in AutoKeras: Once the data is prepared, the user need to load it into AutoKeras using the appropriate data loaders. AutoKeras provides built-in data loaders for common tasks like image classification and text classification.
Image Classification
Text Classification
Building AutoKeras Models
AutoKeras simplifies the process of building deep learning models by automating the selection of model architectures and hyperparameter tuning. Depending on the type of deep learning task, such as image classification, text classification, or regression, AutoKeras automatically determines the best model architecture suited for the data. Here's a step-by-step guide to building AutoKeras models:
Initialize the AutoKeras Task
Users can select the task they want to perform, such as image classification or text classification, by initializing the corresponding AutoKeras task. An example for image and Text classification is given below:
Image Classification
Text Classification
Search for the Best Model Architecture
Use the fit() method to search for the best model architecture. The fit() method automatically searches for different neural network architectures and hyperparameters, maximizing the performance metric specified.
Image Classification
Text Classification
Get the Best Model
Once the training is complete, the best model can be found by AutoKeras:
Image Classification
Text Classification
Users can view a summary of the best model and its architecture using the summary() function. This function provides an overview of the model's layers, output shapes, and the number of trainable parameters.
Now that the best model is identified, it can be used to make predictions on new data:
The best model can also be saved for future use. This can be done by:
It can be loaded once again:
Hyperparameter Tuning
Hyperparameter tuning is a crucial step in optimizing the performance of deep learning models. It involves searching for the best combination of hyperparameters to achieve the highest accuracy and efficiency. AutoKeras automates this process using a technique called Neural Architecture Search (NAS), which explores various hyperparameter configurations to identify the optimal model architecture for a given dataset and task.
AutoKeras defines search spaces for different hyperparameters, including learning rate, number of layers, neurons per layer, and activation functions, among others. The search space determines the range of values that AutoKeras explores during hyperparameter tuning. By default, AutoKeras uses Bayesian optimization, which is a probabilistic model-based optimization method that efficiently searches the hyperparameter space by learning from previous trials. Bayesian optimization helps guide the search to promising regions, making it more efficient than random search.
Model Training and Evaluation
After hyperparameter tuning and obtaining the best model architecture with AutoKeras, the next crucial step is to train and evaluate the model using the best hyperparameters. Proper model training and evaluation are essential to ensure that the model performs well on unseen data and generalizes effectively to real-world scenarios. The training is done as shown in the previous sections. The evaluation is done as shown below:
Interpreting AutoKeras Models
Understanding the learned model architectures is vital for gaining insights into AutoKeras' decision-making process. AutoKeras provides methods to visualize model summaries and interpret important features and parameters.
- Model Summaries and Details: The summary() method offers an overview of the best model's architecture, revealing layer details, trainable parameters, and connections. Graphical representations, such as TensorBoard or Keras' plot_model(), further aid in comprehending the model's structure.
- Interpreting Important Features: For image classification, AutoKeras supports saliency maps, Grad-CAM, and LIME explanations, highlighting influential image regions. For text classification, analyzing word embedding unveils how the model represents words in internal structures.
- Feature Importance Analysis: AutoKeras allows feature importance analysis for tabular data. Techniques like permutation importance or SHAP values identify impactful features, aiding in feature selection and dataset understanding.
Using these tools, advanced users can interpret AutoKeras models effectively and gain valuable insights, promoting trust in their deep learning models' decision-making processes. AutoKeras' interpretability makes it an invaluable asset for building high-performing models while comprehending their inner workings.
Transfer Learning with AutoKeras
Transfer learning is a powerful technique that uses knowledge gained from pre-trained models to enhance the performance of new models on different tasks or datasets. AutoKeras supports transfer learning, enabling users to benefit from the representations learned by existing models and adapt them to their specific tasks. Here's how to perform transfer learning with AutoKeras:
AutoKeras supports various pre-trained models for different types of tasks, such as image classification, object detection, and natural language processing. These models are trained on massive datasets, learning generic features that can be valuable for many downstream tasks. Transfer learning is crucial in deep learning, especially for tasks with limited data or resource constraints. It uses knowledge from pre-trained models, trained on large datasets, to enhance model performance on new tasks. By transferring learned features, models can generalize better and achieve higher accuracy with minimal training data and resources.
AutoKeras supports various pre-trained models for tasks like image classification, object detection, and natural language processing. These models have learned generic features from extensive datasets, making them valuable for diverse downstream tasks. In AutoKeras, users can easily load pre-trained models and fine-tune them on specific datasets for image and text classification tasks. Fine-tuning involves adapting the model to new data while preserving its learned representations.
Fine-tuning entails customizing pre-trained models for new tasks. By freezing some layers and training only the last few, models adapt to new data while benefiting from the knowledge of the pre-trained model. Transfer learning in AutoKeras accelerates model development and improves performance, making it indispensable for deep learning tasks with limited resources or data.
Sample Code for Fine-tuning a Pre-trained Model in AutoKeras:
Limitations of AutoKeras
AutoKeras is a powerful tool for automating the model selection and hyperparameter tuning process, but it does have certain limitations. Understanding these limitations and employing advanced techniques can further optimize its performance for complex tasks.
- Custom Architectures: AutoKeras may not support highly customized model architectures beyond its predefined blocks. In such cases, users might need to use other deep learning frameworks like TensorFlow or PyTorch to build and train custom models.
- Complex Data Pipelines: Handling complex data preprocessing pipelines might require additional manual coding outside of AutoKeras. Some datasets may necessitate custom data augmentation or transformations, which might not be fully supported by AutoKeras.
- Resource Constraints: While AutoKeras aims to simplify the model selection process, exhaustive hyperparameter tuning can be computationally expensive. For tasks with severe resource constraints, fine-tuning or transfer learning might be a more practical option.
Advanced Techniques for AutoKeras
AutoKeras is a powerful automated machine learning library, and by employing advanced techniques, users can further optimize its performance and tailor it to specific deep learning tasks. Here are some advanced techniques and hacks for AutoKeras optimization:
Custom Block Definition
Advanced users can define custom blocks with specific layer configurations using Keras. This approach allows incorporating domain-specific knowledge and building complex architectures beyond AutoKeras' predefined blocks. Custom blocks enable users to fine-tune the model's architecture according to the task's requirements.
Hyperparameter Search Space Customization
AutoKeras performs an automated hyperparameter search during the model selection process. By customizing the hyperparameter search space, users can further tune the model based on their domain knowledge. However, this requires a deeper understanding of the model and may increase the search space complexity, impacting computational resources.
Ensemble Learning
Ensemble learning is a powerful technique that combines predictions from multiple models to produce a more robust and accurate final prediction. Advanced users can combine ensemble learning with AutoKeras by training multiple models with different hyperparameter settings and combining their outputs. This can help reduce overfitting and improve the overall performance of the model.
Sample Code: Image Classification
This example demonstrates how to perform image classification using AutoKeras. We will use the famous CIFAR-10 dataset, which contains 60,000 32x32 color images of 10 different classes.
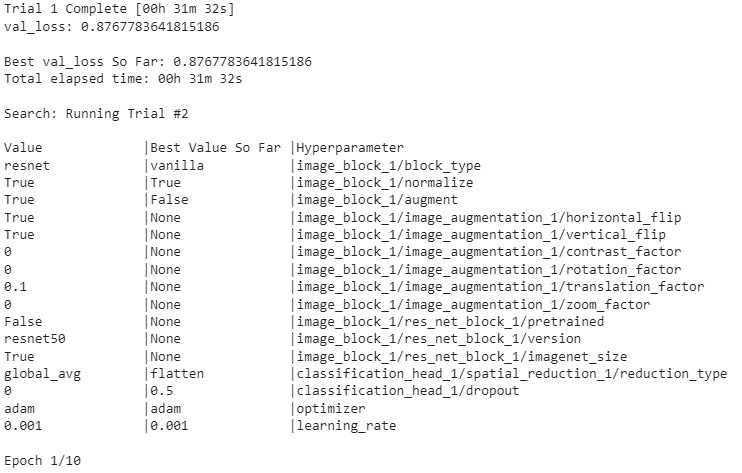
The model is initialized and trained using AutoKeras. During training, AutoKeras automatically searches for the best model architecture and hyperparameters. Once the training is complete, the best model architecture is exported, and its performance is evaluated on the test data. The test accuracy is printed, indicating how well the model performs on unseen data. The code then makes predictions on a subset of sample images from the test data using the trained model. It prints the predicted class and the true class for each sample image, allowing us to analyze how well the model classifies these specific samples.
Conclusion
In this comprehensive guide, readers have been introduced to the power of AutoKeras in deep learning and its automated approach to model selection and hyperparameter tuning. The step-by-step walkthrough demonstrated how image classification can be efficiently performed using AutoKeras with the CIFAR-10 dataset. This guide caters to advanced users, offering valuable insights into the streamlined implementation of deep learning tasks with AutoKeras.
AutoKeras proves to be an invaluable tool as it eliminates the need to manually design architectures and fine-tune hyperparameters. Instead, it empowers researchers and practitioners to focus on the core aspects of their projects, resulting in optimized models for various domains, including image classification, object detection, and natural language processing. The library's ability to use pre-trained models and employ transfer learning enhances its usefulness, especially in scenarios with limited data or resource constraints. The readers can further explore AutoKeras and try it out for their advanced projects. Its adaptability and efficiency offer a convenient way to enhance deep learning capabilities, pushing the boundaries of their research and applications.
This entire workflow has been tried and tested on E2E Cloud. Login to e2enetworks.com to try it out yourself and let us know if you have any feedback.