The 2020 pandemic marked a pivotal moment in modern history, highlighting the urgent need to make health advice accessible to people of all backgrounds. Over the past two years, Generative AI technologies, particularly large language models (LLMs), have demonstrated remarkable potential in broadening access to knowledge and information through human-like language. The question now is: can we leverage LLMs to make healthcare more accessible?
In this article, we will walk you through the process of building a Healthcare AI chatbot that can be integrated into your healthcare app or offered as an add-on service to your customers. We will use an LLM that has been fine-tuned specifically for the healthcare domain, ensuring it understands the specialized vocabulary and knowledge required.
We will also showcase the steps to deploy it on E2E Cloud, which is India’s most price-performant AI cloud offering the advanced cloud GPUs you need for leveraging cutting-edge LLMs from Hugging Face and other sources. We will also explain the importance of data sovereignty and why a MeitY-empanelled CSP like E2E Cloud is the ideal platform for healthcare AI solutions.
Why Create Healthcare Assistant Chatbots
Integrating LLMs into the healthcare domain has the potential to revolutionize the medical industry. It can:
- Increase accessibility and availability of general medical advice.
- Enhance patient engagement.
- Provide 24/7 responses, especially for simple queries, when medical professionals are unavailable or occupied with more urgent cases.
According to NASSCOM, AI in healthcare is projected to contribute between $25 billion and $30 billion to India's GDP by 2025. The World Economic Forum states that it can add $1 trillion to India’s economy by 2035.
Importance of Using a Data Sovereign Architecture
However, healthcare is one of the domains where protecting sensitive information is critical. To ensure this, the businesses operating in this domain typically focus on creating data sovereign applications.
Data sovereignty refers to the principle that data is subject to the laws and regulations of the country in which it is collected or stored. For healthcare chatbots, data sovereignty is important for several reasons:
- Data Security: Storing data within a specific jurisdiction, you can leverage local legal protections and frameworks designed to safeguard data security.
- Cross-Border Data Transfers: If data needs to be transferred across borders, ensure that such transfers comply with international agreements and regulations.
To ensure data sovereignty, companies must deploy the technology stack powering their healthcare AI application within the appropriate jurisdiction and choose a cloud provider that complies with the stringent regulatory requirements demanded by the healthcare sector.
E2E Cloud excels in this area. Our MeitY empanelment ensures compliance with stringent regulatory requirements. With data centers located in India and our cloud adhering to Indian IT laws, you can trust that your data will remain under your control when building on E2E Cloud.
It's important to remember that security and data privacy are shared responsibilities. Ensure you are also adhering to the relevant laws and regulations.
A Guide to Building a Healthcare AI Chatbot
To build this chatbot, we will use Llama3-OpenBioLLM-70B. Llama3-OpenBioLLM-70B model is designed specifically for medical purposes and specially tuned over medical data. With the power of open-source Llama3 and training on over 70 billion parameters, it is one of the best open-source models available for medical use cases.
Prerequisites and Initial Setup
First, sign in or sign up to E2E Myaccount. Once you have signed up, you can launch a GPU instance. You will need a GPU with over 32 GB RAM, or you will need a quantized version (lower accuracy, but also lower RAM requirements).
For this experiment, we suggest using an A100 or H100 Cloud GPU. When you want to scale it later, you can opt for InfiniBand-powered cluster configurations like 4xH100, 8xH100, or even 64xH100.
Once you have set up your cloud GPU and added your public SSH Key, you can get started with the steps below.
The diagram below shows our implementation architecture.

Setting Up the Environment
The first step is to install all the necessary libraries that will be needed to create an efficient healthcare chatbot. We will use LangChain as our framework, and Qdrant as our vector store. We will also use Gradio to create a UI.
First, create a virtual environment.
Next, install the libraries.
Once the installation process is completed, we need to import them into the project. Open VS Code, install remote explorer, and start with the following code:
Loading the Dataset
For this project, we have used a medical dataset from Kaggle. It contains a set of around 1600 medical questions with their respective answers. Let's load the CSV file into our project using pandas.
Now load all the questions and answers into two separate lists for further processing.
Embedding Model
Next load the embedding model from Hugging Face using transformers and convert all the questions into their respective vector embeddings to store them in the vector database.
Storing Embeddings in the Database
We are using Qdrant as the vector store database to store the embeddings generated for the questions.
The first step is to initiate the database client.
Next, we need to create a collection in the database where all the questions will be stored in the form of vector embeddings.
Once the collection is created, upload the respective question embeddings into the database along with their IDs.
Semantic Search
Semantic similarity search will be used here to find documents that match the user query. To perform a similarity search, you have to first convert the user query into vector embeddings, and compare that with the vector embeddings of the dataset already stored (from Kaggle).
Here’s how you can do it.
Constructing the LLM Prompt
To make the response more user-friendly, we will craft the prompt to respond succinctly.
Since we will be using the language model Llama3-OpenBioLLM-70B, the prompt format is that of Llama3.1 specification, and we will ask the LLM to respond based on the context provided. The context will be fetched from the vector database depending on the user query and semantic search.
Creating the Front End
We will be using Gradio to create the front end, where users can put in their queries and get the responses displayed in the web app. In real life, you would be integrating it with your own branded UI.
Results
The final result of our medical chatbot looks like this:
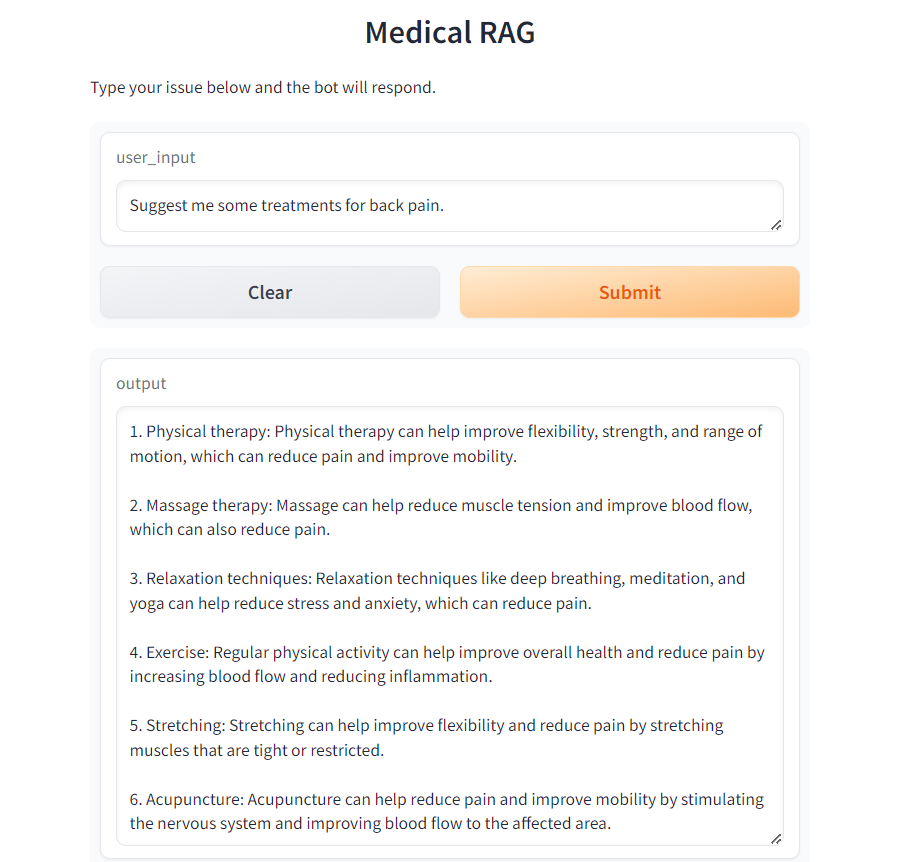
Ensuring Security and Compliance
Before launching the chatbot in production, some security measures should ideally be followed:
- End-to-End Encryption: Ensure that data exchanged between the user and the chatbot is encrypted.
- Regular Security Audits: Review and update security practices to address any vulnerabilities or threats.
- Multi-Factor Authentication: Implement MFA to enhance security by requiring users to provide two or more verification factors like passwords and one-time codes before granting access.
Conclusion
Building a Healthcare AI chatbot leveraging LLMs offers a powerful tool for making healthcare information more accessible, personalized, and timely. By following the steps outlined in this guide, you can create a robust chatbot that enhances patient engagement and supports medical professionals with routine queries.
However, building and deploying such a solution requires a platform that ensures both performance and compliance, particularly with the stringent regulatory requirements in the healthcare sector. E2E Cloud offers the perfect cloud infrastructure with its advanced cloud GPUs, MeitY empanelment, outstanding price-performance ratio, and commitment to data sovereignty.
Ready to take your healthcare AI solution to the next level? Sign up to E2E Cloud today and start building with the confidence that your data is secure, compliant, and hosted on India’s most price-performant AI cloud. You can also reach out to us at sales@e2enetworks.com.