Introduction
In this piece, we will delve into the intriguing world of AI and its propensity to 'hallucinate' in outputs. We will unravel the reasons behind such occurrences, explore the inherent traits of Large Language Models, and highlight potential strategies, such as RLHF, to mitigate these challenges. As we navigate the complexities of AI's capabilities and limitations, we'll also touch upon the broader implications and ethical considerations that come with it. Join us on this exploration and gain a deeper understanding of the balance and intricacies of precision and generalization within AI systems.
Understanding Hallucinations in AI: Definition and Context
In the realm of artificial intelligence (AI), 'hallucination' describes instances where a machine learning model generates results that are either incorrect, unrelated, or lack a basis in its training data. For example, an image recognition algorithm might produce descriptions that include elements not actually present in the image. Similarly, a natural language model such as GPT-4 could create text that contains inaccuracies or is illogical within the context in which it is generated.
Real-World Examples to Illustrate Hallucination
Hallucinations in AI can manifest in a variety of ways, from minor errors to potentially dangerous situations. Here are a few examples:
- Self-driving cars misidentify a plastic bag as a pedestrian: This could lead to unnecessary braking or swerving, which could potentially cause an accident.
- Language models suggest medical advice that is not backed by scientific evidence: This could have serious consequences for the person's health.
- Chatbot designed to provide customer service fabricates information when it doesn't know the answer to a query: This could mislead customers and damage the company's reputation.
In addition to these specific examples, hallucinations in AI can also take more subtle forms. For example, a language model may generate text that is grammatically correct but factually incorrect, or a computer vision system may misidentify objects in images.
It is important to be aware of the potential for hallucinations in AI, especially when developing and deploying AI systems in critical applications. There are a number of techniques that can be used to mitigate the risk of hallucinations, such as using high-quality training data, carefully evaluating system performance, and implementing human-in-the-loop safeguards.
In the following two sections, we'll demystify the phenomenon of hallucinations in AI. First, we'll break it down in layman's terms before delving into the technical aspects. After that, we'll explore whether hallucination is a fundamental characteristic of Large Language Models by examining their statistical properties and discussing some mathematical examples.
Unraveling the Mystery: Why Does Hallucination Happen?
A Simple Explanation for Everyone
Picture yourself as an AI model that has been trained on countless books since its inception. Despite this extensive 'reading,' there might be certain ideas or concepts that you haven't fully grasped. When posed a question about one of these unclear topics, you might attempt to guess an answer. However, occasionally those guesses can be significantly inaccurate. This provides a simple way to understand what hallucination means in AI. Next, let's delve into the more technical details.
Diving Deeper: The Technical Nuances
Hallucinations in AI models can often be attributed to a mix of the following factors:
Data Quality:
Let Q be the quality of the data, quantified using metrics like accuracy, consistency, and bias. Lower values of Q indicate poor quality, which may lead to the model learning inaccuracies.

Here, the Bias Factor and Inconsistency Factor would add penalties for biased or inconsistent data.
Model Architecture:
Let's consider the neural network's architecture, defined by layers L and nodes N. Some architectures (e.g., deep networks with many layers L > 10 but fewer nodes N < 50) could be more susceptible to hallucinations.

Where

is the hallucination factor and
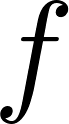
is a function representing the architecture's contribution to hallucinations.
Overfitting and Underfitting:
Let
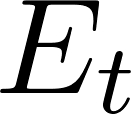
be the error on the training set and
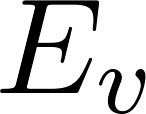
be the error on a validation set. Overfitting is often observed when
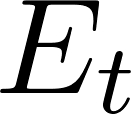
is very low, but
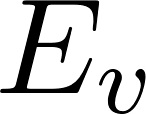
is high. Underfitting is when both
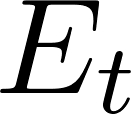
and
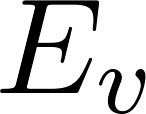
are high, indicating the model failed to capture the underlying trends in the data.
For Overfitting:

For Underfitting:

By understanding these technical nuances and incorporating mathematical reasoning, one can gain a more precise understanding of why hallucinations occur in AI systems.
Small Example of Hallucination: A Simple N-Gram Language Model and Its Limitations
Now let us see an example to better understand this concept of hallucination in AI. The Python code snippet uses the Natural Language Toolkit (NLTK) to create a basic N-gram model—a rudimentary type of language model—that generates sentences based on bigrams, or pairs of adjacent words. The training data for the model consists of a text string that is noticeably biased towards talking about apples, although it does contain single sentences about oranges, bananas, and grapes. When we ask the model to generate a sentence starting with the word 'Oranges,' the output starts off appropriately but then diverges to discuss apples. This transition reflects the model's propensity to 'hallucinate,' which in this context means deviating from the topic at hand (oranges) to favor a subject that is more prevalent in its training data (apples). The example demonstrates the critical role of training data in shaping the model's output, highlighting how biases can lead to unexpected or skewed results—a phenomenon we're referring to as 'hallucination.'
Code:
Output:
Oranges are tasty. I eat apples
The output sentence, 'Oranges are tasty. I eat apples,' starts off with a statement about oranges but then pivots to discuss apples. This is an example of what we refer to as 'hallucination' in AI language models. Even though the model was prompted to generate a sentence beginning with 'Oranges,' it quickly transitioned to talking about apples, which shows the influence of the biased training data focused mainly on apples.
In a broader context, the term 'hallucination' here signifies the model's tendency to deviate from the intended subject matter due to underlying biases or limitations in its training data. Despite being tasked to talk about oranges, the model inadvertently drifts to apples, illustrating how its training data skews its outputs.
This serves as a small but clear-cut example that even in a basic model, biases in the training data can lead to unexpected or skewed outputs. Such outputs can be considered a form of 'hallucination,' underscoring the importance of diverse and balanced training data to achieve more accurate and contextually appropriate results.
Is Hallucination an Inherent Trait of Large Language Models?
The Statistical Nature of LLMs
At the heart of every Large Language Model (LLM) lies the concept of predicting the likelihood of each possible next word given a particular sequence or context. This is an inherently statistical process that offers both remarkable capabilities and notable limitations.
The Role of Probability Distributions
The most fundamental question an LLM tries to answer is: Given a context

, what is the probability
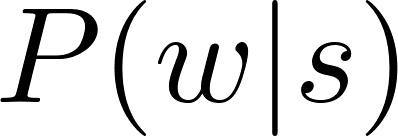
of the next word

appearing? In simpler, early-generation models like N-gram models, this probability would be estimated directly from the occurrences in the training data. For example, in a bigram model:

Here,

is the number of times the sequence
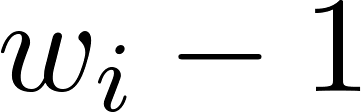
,
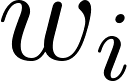
appears in the training data, and

is the number of times the word
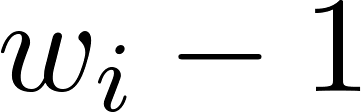
appears.
The Neural Perspective: Softmax Function
Modern LLMs, however, take a more sophisticated approach. They utilize deep neural networks to approximate the probability

. The neural network transforms the context

through multiple layers, resulting in a set of raw scores or 'logits' for each possible next word. These logits are then converted into probabilities using the softmax function:

Where

is the size of the vocabulary, and

is the base of the natural logarithm. The softmax function essentially squashes the raw logits into a probability distribution over the vocabulary.
The Softmax Function and Hallucinations
The implications for hallucinations are multifold:
Dominant Patterns: The training data heavily influences the logits, and therefore the softmax probabilities. If the model frequently observed a specific word following a particular context, it would have a high probability in the softmax output.
Rare Events: If a factually accurate next word was rarely seen in training, its softmax probability could be low, making it unlikely to be generated by the model.
Temperature Settings: The 'temperature' parameter can adjust the softmax probabilities. A higher temperature leads to more random outputs, potentially increasing hallucinations, while a lower temperature makes high-probability events even more likely but at the cost of diversity.
Balancing Act: Precision vs. Generalization
The statistical nature of LLMs implies a delicate balance. On the one hand, the model must be general enough to generate diverse and coherent outputs. On the other hand, it should be precise and cautious to avoid hallucinations and inaccuracies. This balance is the ongoing challenge in the development and fine-tuning of LLMs.
The Risks and Implications of AI Hallucination
When we talk about hallucinations in AI, it's not merely an academic concern or an intriguing quirk in machine learning. The consequences can have serious real-world ramifications, especially as AI models find applications in critical sectors like healthcare, legal systems, and finance. Here's a closer look at how AI hallucinations can impact these sectors:
Potential Consequences in Real-world Applications: When AI Hallucinates
Healthcare: A Matter of Life and Death
Imagine you're in a hospital, and an AI-powered diagnostic tool is used to analyze your X-rays or MRI scans. These systems are trained on massive datasets, but if the data is skewed or if the model architecture is prone to hallucination, you could receive an incorrect diagnosis. For instance, if a machine learning model trained primarily on data from younger patients is used to diagnose an older patient, it may 'hallucinate' the symptoms and recommend inappropriate treatment. In healthcare, incorrect diagnoses can result in ineffective treatments, wasted resources, and even loss of life.
Real-life Example: IBM Watson and Cancer Treatment
IBM's Watson was hailed as a revolutionary tool in oncology, aimed at providing personalized cancer treatment plans. However, there have been reports where Watson made unsafe or incorrect recommendations, primarily due to the data it was trained on. Though not a 'hallucination' in the strictest sense, it's an example of how data quality and model limitations can result in real-world harm. You can read more about this case here.
Legal Systems: The Scales of Justice Tipped
AI is increasingly being used to assist in legal proceedings, from document sorting to predictive policing. A model prone to hallucinations could severely distort legal outcomes. Suppose an AI tool designed to predict criminal behavior is fed biased data—say, it contains arrest records skewed towards a particular ethnic group. Such a model could 'hallucinate' that individuals from that group are more likely to commit crimes, leading to prejudiced outcomes that can ruin lives.
Real-life Example: COMPAS Algorithm
The Correctional Offender Management Profiling for Alternative Sanctions (COMPAS) algorithm, used in the U.S. for risk assessment in sentencing and bail decisions, has been criticized for racial bias, effectively illustrating how biased data can lead to distorted outputs. You can read more about this case here.
Finance: Your Savings at Risk
From trading bots to credit score predictions, AI's footprint in finance is growing. A hallucinating model could offer misguided financial advice, putting your hard-earned money at risk. For example, if a robo-advisor is trained predominantly on bull market data, it may 'hallucinate' that risky assets are safer than they actually are during a bear market.
Real-life Example: 2010 Flash Crash
Although not directly caused by AI hallucination, the 2010 Flash Crash saw the stock market plummet within minutes, largely due to algorithmic trading models executing rapid-fire trades. Had these algorithms been designed with a broader understanding of market conditions, such an event might have been avoided.
Hypothetical Real-world Example: Flash Spike in Cryptocurrency
Imagine an AI trading bot trained primarily on historical data during a bull market for a particular cryptocurrency. If the model hallucinates based on this data, it might aggressively buy the cryptocurrency under the false assumption that its value will only increase. This could artificially inflate the asset's price, leading to an unstable 'bubble.' When the bubble bursts, it could result in massive financial losses for investors who followed the AI's advice.
While we don't have a documented case specifically citing AI hallucination in finance, this hypothetical example aims to illustrate the potential risks involved. Given the speed and stakes of financial markets, even a small hallucination by an AI system can have significant, rapid consequences. Therefore, it's crucial to continue research and development into making these algorithms as robust and reliable as possible.
Ethical Considerations and Accountability
Who is responsible when an AI model hallucinates?
Developers, users, organizations, and regulators all play a role.
Developers create the AI and thus bear some responsibility. However, they often work within limits like tight deadlines or resource constraints, which could impact the AI's reliability.
Users who rely on AI outputs might face the direct consequences of hallucinations but have limited control over the model's development or data quality.
Organizations that deploy AI technologies act as the bridge between developers and users. They make the decision to incorporate AI into their systems and may even profit from it.
Regulatory Bodies are still developing regulations for AI, but they have the potential to establish standards that could minimize risks like hallucination.
In summary, accountability for AI hallucination is a shared responsibility that requires a coordinated approach to address the ethical and practical complexities involved.
Tackling the Challenge: Mitigating Hallucinations in AI Outputs
Next, let's delve into addressing the issue of hallucination in AI—is it a solvable problem? We'll explore existing research methods aimed at mitigating this issue and look at current advancements being made in the AI field to combat hallucinations.
Solution: Introduction to RLHF (Reinforcement Learning from Human Feedback)
One promising avenue of research that has gained considerable attention is Reinforcement Learning from Human Feedback, commonly abbreviated as RLHF. In this approach, the AI model is fine-tuned based on iterative feedback from human reviewers. It's an ongoing, dynamic process designed to make the model's outputs align more closely with human perspectives and expectations.
Research Papers on RLHF
Several research papers have delved into the intricacies of RLHF, testing its effectiveness across various AI models and applications. For instance, a paper by OpenAI titled 'Fine-Tuning Language Models from Human Preferences' explores how RLHF can be applied to large language models to reduce instances of problematic or hallucinated outputs. Now, let's dive deeper into the aforementioned paper for a more comprehensive understanding.
Key Takeaways from the Paper
The paper, published by OpenAI, seeks to improve the reliability of large language models by fine-tuning them based on human feedback. One of the key innovations here is the gathering of comparative data: human reviewers rank different model-generated outputs by quality and appropriateness. The AI model is then fine-tuned to produce outputs that align more closely with the highest-ranked human preferences.
Methodology
The methodology involves multiple iterations where the model is initially trained to predict which output a human would prefer when presented with alternatives. Once the model is fine-tuned based on these predicted preferences, it undergoes further review and iteration. This cycle repeats, allowing for continuous improvement.
Relevance to AI Hallucination
What makes this paper particularly relevant to our discussion is its direct tackle on the issue of hallucination. By aligning the model's understanding with human expectations and norms, the rate of generating hallucinated or problematic outputs is reduced. The fine-tuning process helps the model learn from its mistakes, creating a feedback loop that makes the AI increasingly reliable over time.
Limitations and Future Directions
However, the paper also acknowledges the limitations of RLHF, including the challenges of maintaining a consistent set of human preferences and the computational costs of continuous fine-tuning. Yet, it sets the stage for future research by highlighting the potential of RLHF as a scalable and effective method for improving the safety and reliability of AI systems.
In essence, the 'Fine-Tuning Language Models from Human Preferences' paper offers a promising framework for reducing hallucinations in AI, although more research is needed to address its limitations and explore its full potential. The link to the paper is available in the article's references section at the conclusion. If you're beginning to explore the issue of hallucination in AI, this paper is essential reading.
Other Strategies and Solutions
While Reinforcement Learning from Human Feedback (RLHF) is a promising approach, it's not the only one. Researchers are exploring various other techniques to improve the robustness and reliability of AI models. Let's delve into some of these alternative strategies:
Ensemble Methods
One such approach is ensemble methods, which involve using multiple AI models and aggregating their outputs to make a final decision. By combining the predictions of different models, the chances of hallucination may be reduced.
Advantages:
- Reduced Risk of Hallucination: Using multiple models can average out the individual errors, reducing the risk of hallucination.
- Better Generalization: Ensemble methods often result in better generalization to new data.
Data Augmentation
Another strategy is data augmentation, which involves artificially expanding the training dataset to include edge cases or underrepresented examples. This can be particularly useful for training image or text-based models where hallucination is a concern.
Advantages:
- Richer Training Data: Adding edge cases and variations can result in a model that's less likely to hallucinate.
- Improved Accuracy: More representative data often leads to more accurate models.
Prompt Engineering
Prompt engineering involves carefully designing the input prompts to guide the AI model towards generating more accurate and reliable outputs. Although this may not eliminate hallucinations, it can mitigate their frequency and severity.
Advantages:
- Focused Outputs: A well-designed prompt can guide the model to produce more relevant and accurate content.
- User-Friendly: Prompt engineering often doesn't require any modification to the AI model itself, making it a more accessible solution for end-users.
Example:
- Bad Prompt:
'How can I double my money in a day?'
This prompt could result in advice that is speculative, risky, or even illegal.
- Improved Prompt:
'What are some generally accepted investment strategies for long-term financial growth?'
This rephrasing guides the AI towards providing more responsible financial advice, anchored in commonly accepted investment strategies.
In summary, while no single method can entirely eliminate the risk of hallucination in AI, employing a combination of these strategies can substantially mitigate the issue. From ensemble methods to data augmentation and prompt engineering, each has its own set of advantages and potential applications.
Ongoing Research
Active research is underway not just to improve the accuracy and robustness of AI models but also to make these systems more transparent. Transparency in AI algorithms will allow for better scrutiny, thereby offering another layer of checks against hallucinations. Moreover, it opens up the possibility for real-time corrections and refinements, which could be crucial for applications in sensitive areas like healthcare, finance, or legal systems.
Conclusion
Despite the remarkable progress in machine learning techniques and methods designed to curb the problem of hallucination, achieving total mitigation remains a formidable challenge. One of the primary reasons for this complexity is the dynamic and evolving nature of real-world data. With variables such as societal changes, economic fluctuations, and even natural phenomena continuously altering the data landscape, AI models require constant updates and vigilance to stay relevant and accurate. By understanding the intricacies of hallucination in AI, we can better prepare for a future where these technologies are increasingly integrated into all aspects of our lives. By thoroughly exploring and addressing the issue of hallucination, we are not just making AI more reliable but are also preparing ourselves for a future where AI technologies will increasingly become an integral part of our daily lives—from personalized medicine to autonomous vehicles and beyond. Being prepared for the challenges and being equipped to address them is the best way to make the most of the benefits that AI promises to offer.
References
Here are some potential references you might find useful for further exploration of the topic:
- Fine-Tuning Language Models from Human Preferences
- IBM’s Watson supercomputer recommended ‘unsafe and incorrect’ cancer treatments, internal documents show
- Can the criminal justice system’s artificial intelligence ever be truly fair?