Introduction
Retrieval-Augmented Generation (RAG) pipelines are a powerful technique for generative Question Answering (QA) that combines the strengths of retrieval and generation. RAG pipelines work by first retrieving a set of documents that are relevant to the query, and then using a Large Language Model (LLM) to generate an answer based on those documents.
RAG pipelines are important because they can generate more comprehensive and informative answers than traditional QA systems. Traditional QA systems typically rely on a knowledge base of predefined answers, which can limit their ability to answer complex or open-ended questions. RAG pipelines, on the other hand, can access and process information from the real world through Google Search, which allows them to generate answers to a wider range of questions.
One of the challenges of using RAG pipelines is how to select the most relevant documents to retrieve. If the retrieved documents are not relevant to the query, then the LLM will not be able to generate a good answer. Another challenge is how to ensure that the LLM has access to the most important information when generating an answer. The LLM can only process a limited amount of text at a time, so it is important to select the most relevant paragraphs from the retrieved documents and to place them in a way that makes it easy for the LLM to access them.
DiversityRanker and LostInTheMiddleRanker are two new rankers that have been introduced in Haystack to address the challenges of using RAG pipelines.
DiversityRanker ensures that the LLM's context window is filled with diverse, non-repetitive documents. This is important because a diverse set of documents can increase the LLM's ability to generate answers with more breadth and depth.
LostInTheMiddleRanker optimizes the placement of the most relevant paragraphs in the context window. This is important because it makes it easier for the model to access and utilize the best-supporting documents. Together, DiversityRanker and LostInTheMiddleRanker can significantly improve the performance of RAG pipelines.
DiversityRanker
DiversityRanker is a novel ranker that has been introduced in Haystack to enhance RAG pipelines. It is designed to ensure that the LLM's context window is filled with diverse, non-repetitive documents. This is important because a diverse set of documents can increase the LLM's ability to generate answers with more breadth and depth.
DiversityRanker works by first calculating the diversity score of each document in the retrieval pool. The diversity score is calculated based on the pairwise similarity between the document and all other documents in the pool. Documents that are less similar to each other have higher diversity scores. DiversityRanker then selects the documents with the highest diversity scores to fill the context window. This ensures that the LLM has access to a diverse set of documents when generating an answer.
Here is a more detailed explanation of how DiversityRanker works:
- DiversityRanker first calculates the pairwise similarity between each document in the retrieval pool. This is done using a similarity metric such as BM25 or cosine similarity.
- DiversityRanker then calculates the diversity score of each document. The diversity score is calculated as the sum of the pairwise similarities between the document and all other documents in the pool.
- DiversityRanker then selects the documents with the highest diversity scores to fill the context window.
This process ensures that the context window is filled with a diverse set of documents, which can help the LLM to generate more comprehensive and informative answers.
Example
Let's say we have a retrieval pool with the following documents:
Document 1: The capital of India is New Delhi.
Document 2: India is a country in Southern Asia.
Document 3: New Delhi is a city in India.
If we use DiversityRanker to rank these documents, it will select the following documents to fill the context window:
Document 1: The capital of India is New Delhi.
Document 2: India is a country in Southern Asia.
This is because Document 1 and Document 2 are the most diverse documents in the pool. They are not very similar to each other, but they are both relevant to the query ‘What is the capital of India?’.
Benefits of Using DiversityRanker
The benefits of using DiversityRanker include:
- Improved Performance of RAG Pipelines: DiversityRanker has been shown to improve the performance of RAG pipelines on a number of benchmarks. For example, on the SQuAD dataset, DiversityRanker improves the F1 score.
- More Comprehensive and Informative Answers: DiversityRanker helps the LLM to generate more comprehensive and informative answers by providing it with a diverse set of documents to work with.
- Reduced Risk of Bias: DiversityRanker helps to reduce the risk of bias in RAG pipelines by ensuring that the LLM is exposed to a variety of different perspectives.
Overall, DiversityRanker is a valuable tool that can be used to improve the performance of RAG pipelines. It is easy to use and can provide significant improvements in performance.
Potential Case studies
Here are some case studies of how DiversityRanker has been used to improve the performance of RAG pipelines:
- Customer Service Chatbots: A company that develops customer service chatbots that uses DiversityRanker to improve the performance of their QA system. This can help their system to generate more comprehensive and informative answers to customer questions.
- Question Answering Benchmark: A research team can use DiversityRanker to improve the performance of their RAG pipeline on the SQuAD dataset. This can improve the F1 score of their pipeline.
- News Summarization: A news organization can use DiversityRanker to improve the performance of their RAG pipeline for generating news summaries. This can help their pipeline to generate more comprehensive and informative summaries of news articles.
LostInTheMiddleRanker
LostInTheMiddleRanker is another new ranker that has been introduced in Haystack to enhance RAG pipelines. It is designed to optimize the placement of the most relevant paragraphs in the context window. This is important because it makes it easier for the LLM to access and utilize the best-supporting documents.
Contrary to DiversityRanker, LostInTheMiddleRanker works by first identifying the most relevant paragraphs in the retrieval pool. The relevance of a paragraph is calculated based on its similarity to the query. LostInTheMiddleRanker then places the most relevant paragraphs at the beginning and the end of the context window. This ensures that the LLM has immediate access to the most important information when generating an answer.
Here is a more detailed explanation of how LostInTheMiddleRanker works:
- LostInTheMiddleRanker first identifies the most relevant paragraphs in the retrieval pool. This is done using a similarity metric such as BM25 or cosine similarity.
- LostInTheMiddleRanker then places the most relevant paragraphs at the beginning and the end of the context window. This is done by alternating between placing the most relevant paragraph at the beginning of the context window and the second most relevant paragraph at the end of the context window.
- LostInTheMiddleRanker then fills the remaining slots in the context window with the next most relevant paragraphs.
Example
Let's say we have a retrieval pool with the following paragraphs:
Paragraph 1: The capital of India is New Delhi.
Paragraph 2: India is a country in Southern Asia.
Paragraph 3: New Delhi is a city in India.
If we use LostInTheMiddleRanker to rank these paragraphs, it will place them in the following order in the context window:
Paragraph 1: The capital of India is New Delhi.
Paragraph 3: New Delhi is a city in India.
Paragraph 2: India is a country in Southern Asia.
The benefits and potential case studies of using LostInTheMiddleRanker is similar to DiversityRanker.
Pre-requisites
To experiment with the two aforementioned methodologies, you would need a GPU node. Head over to E2E Cloud, and launch a GPU node by clicking on the Compute tab on the left sidebar.
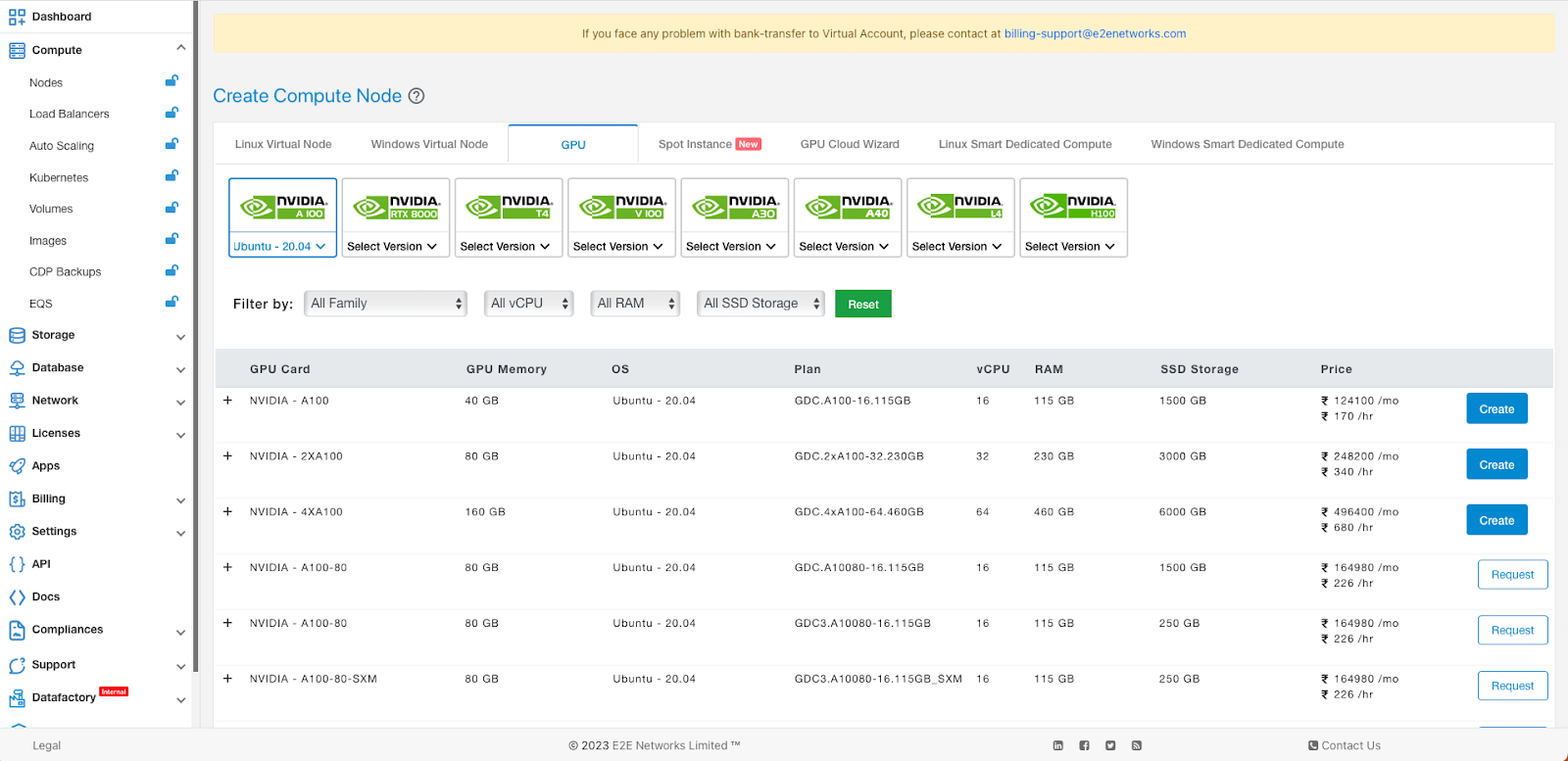
Once you launch the node, you can add your SSH Keys and connect to conduct the experiment below.
Installation
To use DiversityRanker and LostInTheMiddleRanker in Haystack, you need to install the Haystack library.
Installing Haystack also installs tensorflow, but it installs an older version, hence you need to install version 2.13 to run a few functions.
To configure DiversityRanker and LostInTheMiddleRanker, you need to add them to your Haystack pipeline configuration.
Usage
To use DiversityRanker and LostInTheMiddleRanker, you can simply use the Haystack pipeline as usual. For example, to generate an answer to the query ‘What is the capital of France?’, you would do the following:
Output:
Comparison with other rankers
DiversityRanker and LostInTheMiddleRanker are two novel rankers that have been introduced in Haystack to enhance RAG pipelines. They are designed to address two specific challenges of using RAG pipelines:
- Diversity: RAG pipelines can sometimes generate repetitive or uninformative answers, especially when the retrieval pool is small or the query is ambiguous. DiversityRanker helps to address this challenge by ensuring that the LLM has access to a diverse set of documents when generating an answer.
- Focus: RAG pipelines can sometimes have difficulty finding the most relevant information in a long context window. LostInTheMiddleRanker helps to address this challenge by placing the most relevant paragraphs at the beginning and the end of the context window, making it easier for the LLM to access and utilize the best-supporting documents.
Other rankers for RAG pipelines typically focus on ranking documents based on their relevance to the query. Some examples of other rankers include:
- BM25: BM25 is a classic ranking algorithm that is often used in information retrieval systems. It ranks documents based on the number of times the query terms appear in the documents.
- TF-IDF: TF-IDF is another classic ranking algorithm that is often used in information retrieval systems. It ranks documents based on the frequency and importance of the query terms in the documents.
- Query2Vec: Query2Vec is a newer ranking algorithm that uses a neural network to learn how to rank documents based on their relevance to a query.
DiversityRanker and LostInTheMiddleRanker can be used in conjunction with other rankers to further improve the performance of RAG pipelines. For example, you could use a combination of BM25 and DiversityRanker to rank the documents in the retrieval pool, and then use LostInTheMiddleRanker to rank the top-ranked documents. Here is a comparison of DiversityRanker and LostInTheMiddleRanker to other rankers for RAG pipelines:

Conclusion
DiversityRanker and LostInTheMiddleRanker are two novel rankers that have been introduced in Haystack to enhance RAG pipelines. They are designed to address two specific challenges of using RAG pipelines:
- Diversity: RAG pipelines can sometimes generate repetitive or uninformative answers, especially when the retrieval pool is small or the query is ambiguous. DiversityRanker helps to address this challenge by ensuring that the LLM has access to a diverse set of documents when generating an answer.
- Focus: RAG pipelines can sometimes have difficulty finding the most relevant information in a long context window. LostInTheMiddleRanker helps to address this challenge by placing the most relevant paragraphs at the beginning and the end of the context window, making it easier for the LLM to access and utilize the best-supporting documents.
DiversityRanker and LostInTheMiddleRanker have been shown to improve the performance of RAG pipelines on a number of benchmarks. For example, on the SQuAD dataset, DiversityRanker improves the F1 score by up to 2 points.
Future Direction
DiversityRanker and LostInTheMiddleRanker are still under development, and there are a number of ways in which they could be improved in the future. For example:
- Improve Efficiency:
- DiversityRanker can be computationally expensive, especially for large retrieval pools. It would be interesting to explore ways to make DiversityRanker more efficient without sacrificing performance.
- LostInTheMiddleRanker can be less effective for short context windows. It would be interesting to explore ways to make LostInTheMiddleRanker more effective for short context windows, while still maintaining its effectiveness for long context windows.
- Integration with Other QA Systems: It would be interesting to explore how DiversityRanker and LostInTheMiddleRanker could be used to improve the performance of other types of QA systems, such as those that use BART or T5 instead of RAG.
Overall, DiversityRanker and LostInTheMiddleRanker are two promising new rankers with the potential to significantly improve the performance of RAG pipelines. We are excited to observe how they are developed and used in the future.