Introduction
Transformers have become the preferred architecture for many machine learning tasks, especially in Natural Language Processing (NLP). They use self-attention mechanisms to process data sequences in parallel, capturing complex dependencies better than previous models like RNNs and LSTMs. This enhancement has been beneficial in translation, question-answering, and other areas. Moreover, Transformers have shown potential in fields beyond NLP, including computer vision, audio processing, and drug discovery.
As Transformers have grown larger and more complex, their computational demands have also increased. Training these models requires large datasets, extensive resources, and significant energy, making them costly and less accessible. Therefore, creating efficient models is crucial for democratizing advanced machine learning, allowing more people to contribute to and benefit from these technologies.
There is an increasing focus on making Transformer models more efficient and accessible by simplifying their architecture. The goal is to reduce the model to its essential components without sacrificing performance. Notable work in this area includes the proposed version of the Transformer block, which is less complex but performs as well or better than traditional models. This development could lead to faster training and fewer parameters, contributing to more sustainable and scalable machine learning models.
The Complexity of Standard Transformer Blocks
The Pre-Layer Normalization Transformer block, commonly used in Transformer models, includes several layers for effective sequence processing. Central to this configuration are the Multi-Head Attention (MHA) mechanisms, which assess the significance of various input data segments. The attention outputs are projected, normalized, and then processed by a feed-forward neural network or Multi-Layer Perceptron (MLP) block. This MLP block usually comprises two layers separated by a non-linear activation function.
Each component in a Transformer block plays a vital role:
- Multi-Head Attention (MHA): It allows the model to focus on different parts of the input sequence, which is crucial for understanding context and relationships within the data.
- Projection: Transforms the attention output into a space that is more suitable for the MLP to process.
- Normalization: Applied before each sub-block (MHA and MLP), it stabilizes the learning by ensuring that the activations across the network don’t vary too much, preventing the so-called internal covariate shift.
- MLP Block: Processes the normalized attention output further to capture complex data patterns.
- Skip Connections: These allow gradients to flow through the network more easily by providing shortcuts for the backward pass during training, helping to mitigate the vanishing gradient problem.
- Pre-Layer Normalization (Pre-LN): Refers to the placement of normalization layers before the MHA and MLP components, which has been found to improve training stability for deeper models.
Each component in Transformers is essential, but their combined complexity results in a network that's resource-intensive and often delicate. Training requires balancing hyperparameters and precise initialization to avoid gradient issues. The complexity leads to high computational costs and the need for powerful hardware, which isn't always accessible. Small changes in the architecture can significantly impact performance, limiting the model's adaptability. Furthermore, this complexity can obscure how the models function, complicating troubleshooting and interpretation. With the growing demand for both powerful and practical models, there's an effort to simplify the Transformer architecture without compromising its capabilities, leading to the development of more streamlined Transformer blocks.
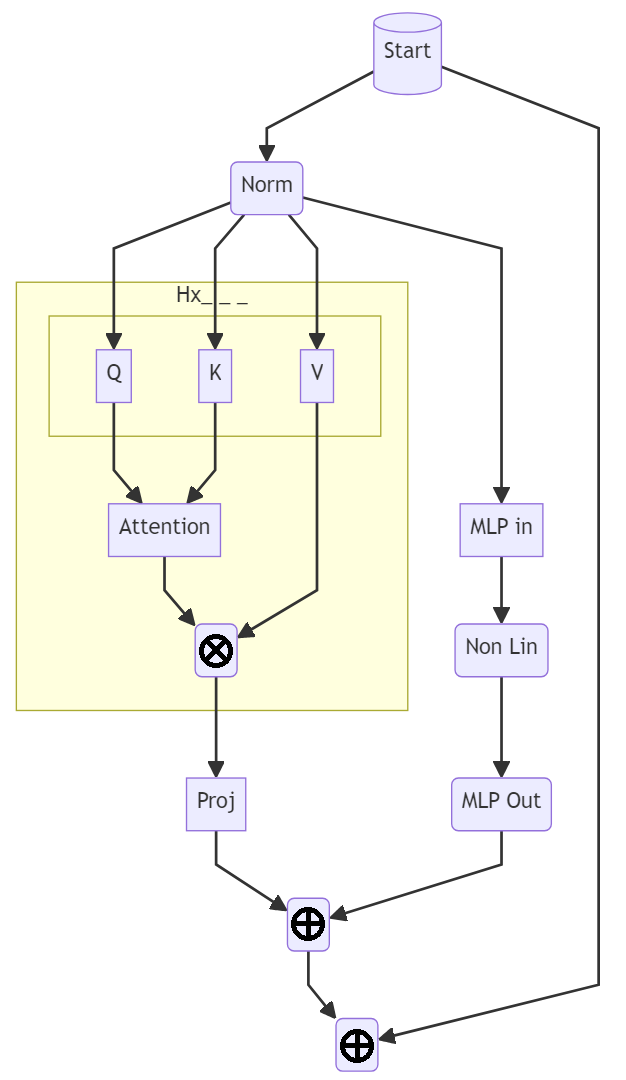
Motivation for Simplification
The drive to simplify Transformer blocks stems from the need for models that are both quick and efficient in terms of parameters. Speed is crucial not only in deployment but also during training, as faster training enables more experimentation and quicker research progress. Parameter efficiency aims for equal or better performance using fewer parameters, reducing computational heaviness. Models with fewer parameters typically use less memory and compute faster, enhancing accessibility and ease of deployment, particularly on devices with limited resources.
The shift towards simplified Transformer blocks is influenced by practical needs and theoretical research. Studies on signal propagation in neural networks indicate that some Transformer components might not be as essential as previously believed. Empirical evidence also shows that models can retain performance with certain elements removed or modified. Based on these theoretical and empirical findings, researchers are experimenting with streamlined Transformer blocks, exploring how much they can simplify while carefully observing performance impacts.
Streamlining Transformer architecture offers multiple advantages. Primarily, it reduces training time and computational resources, democratizing model development and enabling wider participation in AI advancements. Simplified models are also easier to understand and debug, enhancing transparency and trustworthiness, crucial in fields like healthcare and finance where interpretability matters. Additionally, streamlined models may generalize better, avoiding over-parameterization and its associated risk of overfitting on training data. Simplifying Transformer blocks could lead to a new wave of efficient and effective models that carry forward the strengths of their predecessors while addressing the limitation of their complexity.
The Simplified Transformer Block Explained
The proposed simplified Transformer block is a streamlined version of the traditional model, designed to keep crucial functions while eliminating unnecessary complexity. The key goal is to preserve the model's capacity for effective learning and generalization across tasks. This involves carefully assessing the contribution of each component to overall performance and methodically evaluating the effects of removing or altering these components.
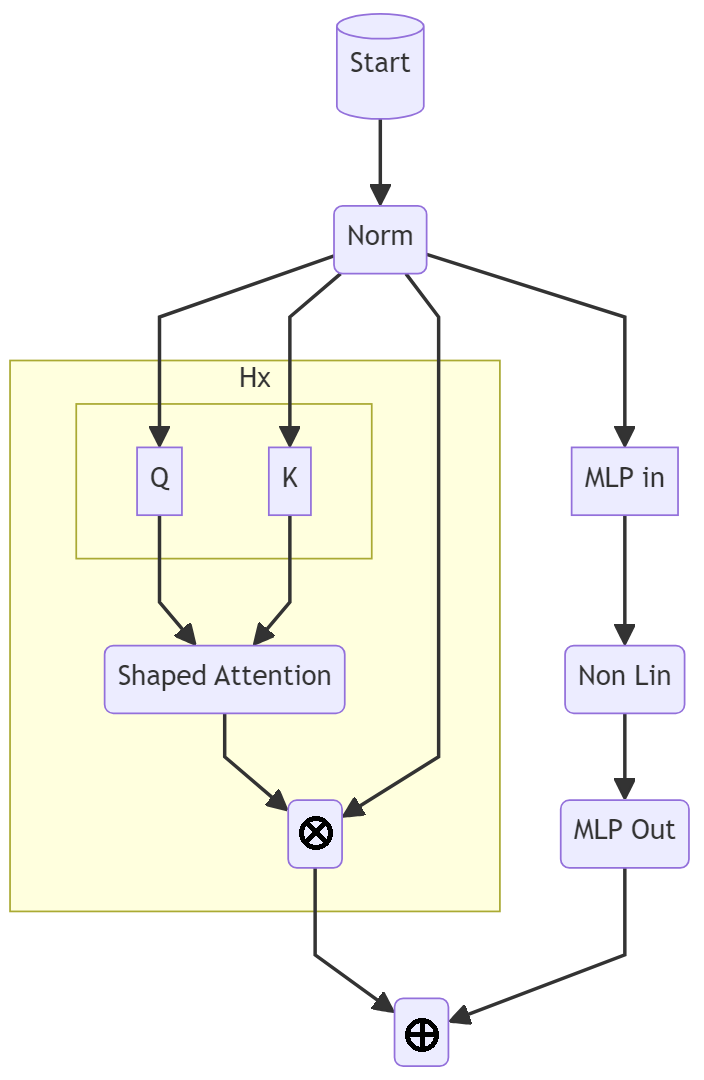
Several key components have been either removed or significantly modified in the simplified Transformer block:
- Skip Connections: Traditional blocks use skip connections to facilitate gradient flow, but these have been removed, suggesting that with the right adjustments, they may not be necessary for maintaining performance. Their removal is based on the insight that they may not be crucial for all types of models, especially if other components are adjusted to compensate for their absence.
- Projection Matrix: The projection layer following the attention mechanism has been eliminated, streamlining the data path from attention to MLP layers. By removing the projection matrix, the model reduces computational load and parameter count, which can speed up training without a noticeable loss in performance.
- Value Parameters: These parameters in the attention mechanism have been removed, simplifying the attention computation. The empirical observation that the model can rely solely on the query (Q) and key (K) matrices for attention suggests that the value parameters might be redundant.
- Normalization Layers: In some configurations of the simplified Transformer block, normalization layers, which are typically applied before each sub-block, is repositioned or even entirely removed. This adjustment or removal is supported by signal propagation theory, suggesting that with meticulous initialization and training, normalization may become less essential. This approach aligns with efforts to streamline the architecture while maintaining effective model performance.
Each modification is a careful step towards a Transformer block that is leaner, more efficient, and potentially more versatile. The implications of these changes are intense, suggesting that the Transformer architecture can be significantly more lightweight without sacrificing the qualities that make it so powerful.
Empirical Results and Observations
The empirical findings from simplifying the Transformer block are significant. The research conducted involved a series of experiments to test the performance of the simplified blocks against the standard Pre-LN Transformer blocks. The experiments were methodical, ensuring that each modified Transformer was tested across a range of tasks and conditions to validate the robustness of the simplifications.
Simplified Transformer blocks showed a notable increase in training speed, with models training up to 15% faster. This efficiency results from reducing parameters and computational complexity. Performance-wise, these streamlined blocks equaled or surpassed traditional architecture in tasks like next-token prediction and those used in BERT models. This maintained performance, despite reduced complexity, indicates that the omitted components might not have been as vital for learning as once believed. A 15% reduction in parameters means lower memory requirements, enabling training and deployment on less powerful hardware, including edge devices. This opens avenues for real-time applications in resource-limited environments.
The increase in training throughput means that models can be trained on larger datasets within the same time frame or the same dataset in a shorter period. This can significantly accelerate the development cycle for new models and applications. Additionally, the reduction in parameters and the associated computational load can decrease the carbon footprint of training large models, contributing to more sustainable AI practices. It also means that researchers and organizations without access to vast computational resources can participate in developing state-of-the-art models, democratizing the field of AI.
Practical Applications and Broader Implications
Simplified Transformer blocks have demonstrated effectiveness in both autoregressive models, like GPT and BERT encoder architectures, essential in various natural language processing applications. In autoregressive tasks such as text generation and language modeling, the streamlined architecture facilitates faster training and more efficient deployment without sacrificing text quality. For BERT encoder models, used in language understanding and processing tasks like sentiment analysis, question-answering, and document summarization, simplification allows for quicker task-specific adaptability with reduced resource demands.
The impact of a simplified Transformer architecture reaches beyond NLP to other deep learning domains, like computer vision for image classification and object detection, and even bioinformatics for protein structure prediction. In these fields, the advantages of a more efficient model are evident: shorter training times, decreased computational costs, and the capability to deploy advanced models on devices with limited processing capabilities, like smartphones and embedded systems.
The advancements in simplified Transformer architecture offer substantial benefits to the industry. Companies can deploy advanced AI models more affordably, allowing small and medium-sized enterprises to utilize cutting-edge AI technologies. Academically, the lower computational demands enable researchers with limited resources to conduct advanced AI research, promoting innovation and diversity in AI.
Additionally, this simplification aligns with the increasing emphasis on sustainable AI practices. As the environmental impact of large-scale AI computations grows more concerning, efficient models like these present a pathway towards more eco-friendly AI research and development. In summary, the simplified Transformer block marks a significant progress in neural network architecture design. It demonstrates the possibility of top-tier performance with an efficient, accessible approach, potentially ushering in a new era of AI. This advancement could make advanced AI capabilities more attainable for a wider audience, leading to sustainable, broadly distributed AI applications.
Challenges and Limitations
In addition to the advantages of the simplified Transformer block, it is also crucial to consider its limitations. A key concern is the potential reduction in depth and richness of learning compared to more complex models. Standard Transformer blocks, though resource-intensive, might capture data nuances that simpler models overlook. This is especially relevant in tasks demanding high-level understanding and subtlety, like nuanced language translation, complex sentiment analysis, or advanced generative tasks.
Moreover, while the empirical results for simplified blocks are promising, they are based on specific datasets and tasks. The extent to which these findings apply universally to all data and task types is uncertain. In some specialized applications, the complete complexity of the standard Transformer architecture may still provide superior results.
There are scenarios where the standard, more complex, Transformer architecture might still be necessary or preferable:
- Decision-Making: In fields like healthcare or finance, where the cost of errors is very high, the added complexity and potential for deeper understanding with standard Transformer blocks might be justified.
- Advanced Research Applications: In cutting-edge research, where exploring the limits of what AI can achieve is the goal, the full complexity of standard Transformers may offer insights that simplified models do not.
- Rich Multimodal Tasks: Tasks that involve multimodal data might benefit from the deeper feature extraction and processing capabilities of more complex Transformer architectures.
In scenarios where a deeper, more nuanced understanding is critical, the choice may favor standard, more complex Transformer models over simplified ones. The simplified Transformer block represents just one option in a range of architectural choices. Selecting between a simplified and a standard model should be based on the specific needs and limitations of the task at hand. The simplified Transformer block offers promising opportunities for efficient and accessible AI, but it's part of a wider array of tools available to practitioners. Choosing the right tool depends on specific project requirements and constraints.
Conclusion
The study of the simplified Transformer block offers several important insights, reshaping our understanding of AI architectures:
- Efficiency in Design: This model shows it's feasible to retain or even enhance neural network performance while greatly reducing complexity and computational needs.
- Complexity and Performance: The modifications to the standard Transformer architecture – such as removing skip connections, projection matrices, and value parameters, and altering normalization layers – highlight a critical balance between model complexity and operational efficiency.
- Empirical Validation: The results from various experiments indicate that these models can achieve similar or better training speeds and maintain robust performance across a range of tasks, signaling a promising direction for future model design.
Simplifying Transformer architectures marks a pivotal moment in the evolution of deep learning. It reflects a growing recognition of the need for models that are both powerful and practical. Models that can be trained quickly, require fewer resources, and are accessible to a broader range of users and applications. The development of simplified transformer blocks is likely to inspire further innovations in neural network design. This shift could lead to more sustainable AI practices and democratize access to cutting-edge technologies.
Moreover, the principles learned from simplifying Transformer blocks could be applied to other areas of deep learning, potentially leading to breakthroughs in how we construct and understand neural networks. As we continue to push the boundaries of AI, it would lead to making them more efficient, understandable, and widely applicable.
In conclusion, the simplified Transformer block is not just a technical achievement; it is a step towards a future where advanced AI is more sustainable, accessible, and adaptable – a future where the benefits of AI can be more evenly distributed across different sectors and communities.