AI frameworks are software libraries or platforms that provide developers with tools, APIs, and pre-built components to build and deploy artificial intelligence (AI) models and applications. These frameworks offer a set of functionalities and abstractions that simplify the process of developing AI systems by handling the complex underlying operations and algorithms.
How Are AI Frameworks Helpful for Developers?
AI frameworks are of great importance for developers to harness the power of Artificial Intelligence and Machine Learning. They provide a set of tools, libraries, and APIs that simplify the process of building, training, and deploying AI models.
By providing high-level abstractions, these frameworks allow developers to focus on the core logic of their AI applications without getting bogged down by intricate mathematical computations or low-level operations. This abstraction streamlines the development process, enabling faster prototyping, experimentation, and implementation of AI models.
Furthermore, AI frameworks offer optimized implementations and leverage hardware accelerators, such as GPUs, to maximize efficiency and performance. They provide pre-built components, algorithms, and optimization techniques that are fine-tuned for AI tasks. Developers can benefit from the extensive ecosystems and communities surrounding these frameworks, gaining access to valuable resources, documentation, and support. With AI frameworks, developers can leverage the power of AI and machine learning, creating cutting-edge applications that address a wide range of real-world challenges.
Top Open Source AI Frameworks You Can Learn in 2023
Open-source AI frameworks are software frameworks that are available freely and are distributed under an open-source license. These frameworks provide a set of tools, libraries, and resources that enable developers to build, train, and deploy AI/ML models. Open-source AI frameworks allow developers to access and modify the source code, contributing to the improvement and customization of the framework to meet their specific needs.
Some of the most widely used AI Frameworks are:
Tensor Flow
TensorFlow is an open-source AI framework developed by Google. It is widely used by developers and researchers due to its versatility and powerful features. TensorFlow allows users to build and deploy machine learning models for various applications, including image and speech recognition, natural language processing, and more. TensorFlow stands apart from other frameworks because of its scalability and flexibility. It offers a comprehensive ecosystem of tools, libraries, and resources that enable developers to design complex neural networks and implement cutting-edge algorithms. TensorFlow supports both high-level APIs, such as Keras, which provide a user-friendly interface for building models quickly, and low-level APIs, which offer finer control over the model architecture and optimization. This flexibility allows developers to experiment with different approaches and tailor their models to specific requirements.
PyTorch
PyTorch is a popular open-source machine learning framework that has gained significant traction among developers and researchers. It offers a dynamic computational graph, making it particularly suitable for projects that involve complex and dynamic neural networks. PyTorch's flexibility and ease of use have made it a preferred choice for many deep learning practitioners.
A unique feature offered by PyTorch is the dynamic computational graph, which allows for dynamic changes to the model’s architecture during runtime. This feature provides an intuitive and interactive development experience, making it easier to experiment with different model designs and debug code. Additionally, PyTorch's Pythonic syntax and straightforward API make it accessible to users of varying skill levels.
Theano
Theano is a Python library for deep learning that also works well with NumPy. It allows developers to define, optimize, and evaluate mathematical expressions involving multi-dimensional arrays efficiently. Theano was developed to be a low-level framework that provides a foundation for building and training neural networks.
One of the key advantages of Theano is the ability to perform symbolic mathematical computations. This means that users can define mathematical operations symbolically and let Theano automatically optimize and compile them for efficient execution. This approach enables Theano to leverage the computational power of GPUs and CPUs, delivering high-performance computations. Theano also offers automatic differentiation, allowing users to compute gradients of expressions easily, which is crucial for training neural networks through techniques like backpropagation.
Keras
Keras is a high-level open-source neural network library that runs on top of other deep learning frameworks, including TensorFlow and Theano. It provides a user-friendly and intuitive interface for building and training deep learning models. Keras aims to simplify the process of developing deep learning models by offering a consistent and easy-to-use API.
Keras offers a variety of utilities and tools that facilitate the model development process. It provides various optimization algorithms, loss functions, and evaluation metrics that can be easily integrated into the training pipeline. Keras simplifies the process of training models with methods like automatic differentiation, batch normalization, and dropout regularization. Furthermore, Keras includes functionalities for model saving, loading, and visualization, allowing users to analyze and deploy their models efficiently.
Caffe
Convolutional Architecture for Fast Feature Embedding (CAFFE) is an open-source deep learning framework developed by Berkeley AI Research (BAIR). It is specifically designed for efficiency and speed, with a primary focus on convolutional neural networks (CNNs) and computer vision tasks. Caffe provides a simple and expressive architecture for defining and training deep learning models.
Caffe supports a wide range of network architectures and layer types, including convolutional layers, pooling layers, fully connected layers, and more. It provides a declarative model definition through its configuration files, which allows users to define network architectures and specify layer properties and connections. This approach simplifies the model development process and makes it easier to experiment with different network configurations.
Scikit-Learn
Scikit-learn is a popular open-source machine learning library for Python. It provides a wide range of tools and algorithms for various machine learning tasks, including classification, regression, clustering, dimensionality reduction, and model selection. SciKit-learn is designed to be user-friendly and efficient, making it a go-to choice for both beginners and experienced practitioners in the field of machine learning.
SciKit-learn provides a suite of utilities and functions for data preprocessing, feature extraction, and model evaluation. It offers methods for data normalization, handling missing values, feature scaling, and feature selection, allowing users to preprocess their data effectively before feeding it into the models. SciKit-learn also includes various evaluation metrics, cross-validation techniques, and model selection tools to assess the performance of models and select the best one for a given task.
Deploying Top AI Frameworks with E2E Cloud
To use these frameworks and deploy them on E2E Cloud, you can follow these steps:
Log in to the MyAccount portal of E2E Networks.

Under the “Compute” menu on the extreme left, click on “GPU”. Then click on “GPU Cloud Wizard”.

You can select the framework best suited for your requirements, and click on Next. Further, you can choose whether you want a committed node or an hourly billed node.
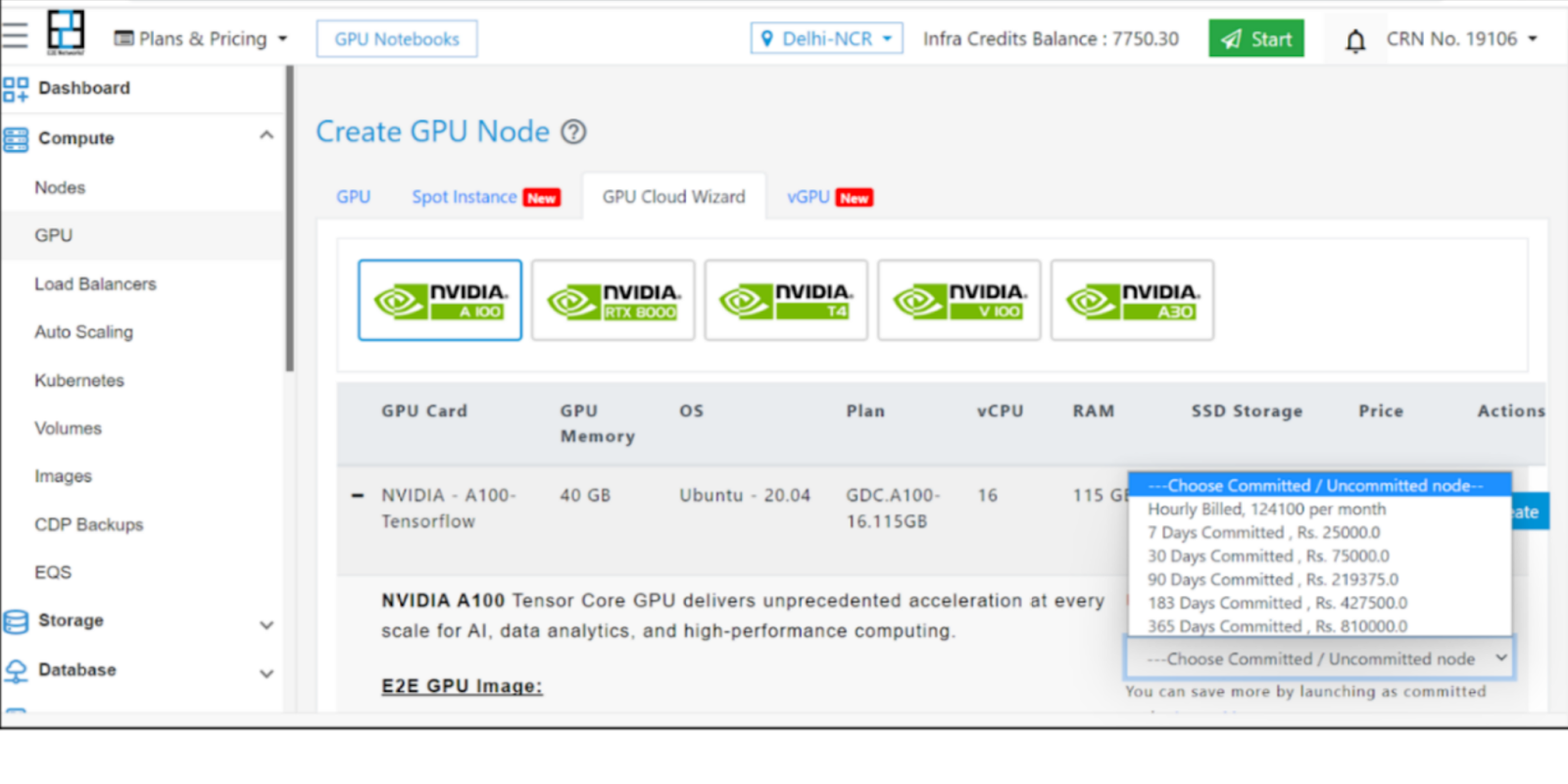
Click on Create to launch the machine.
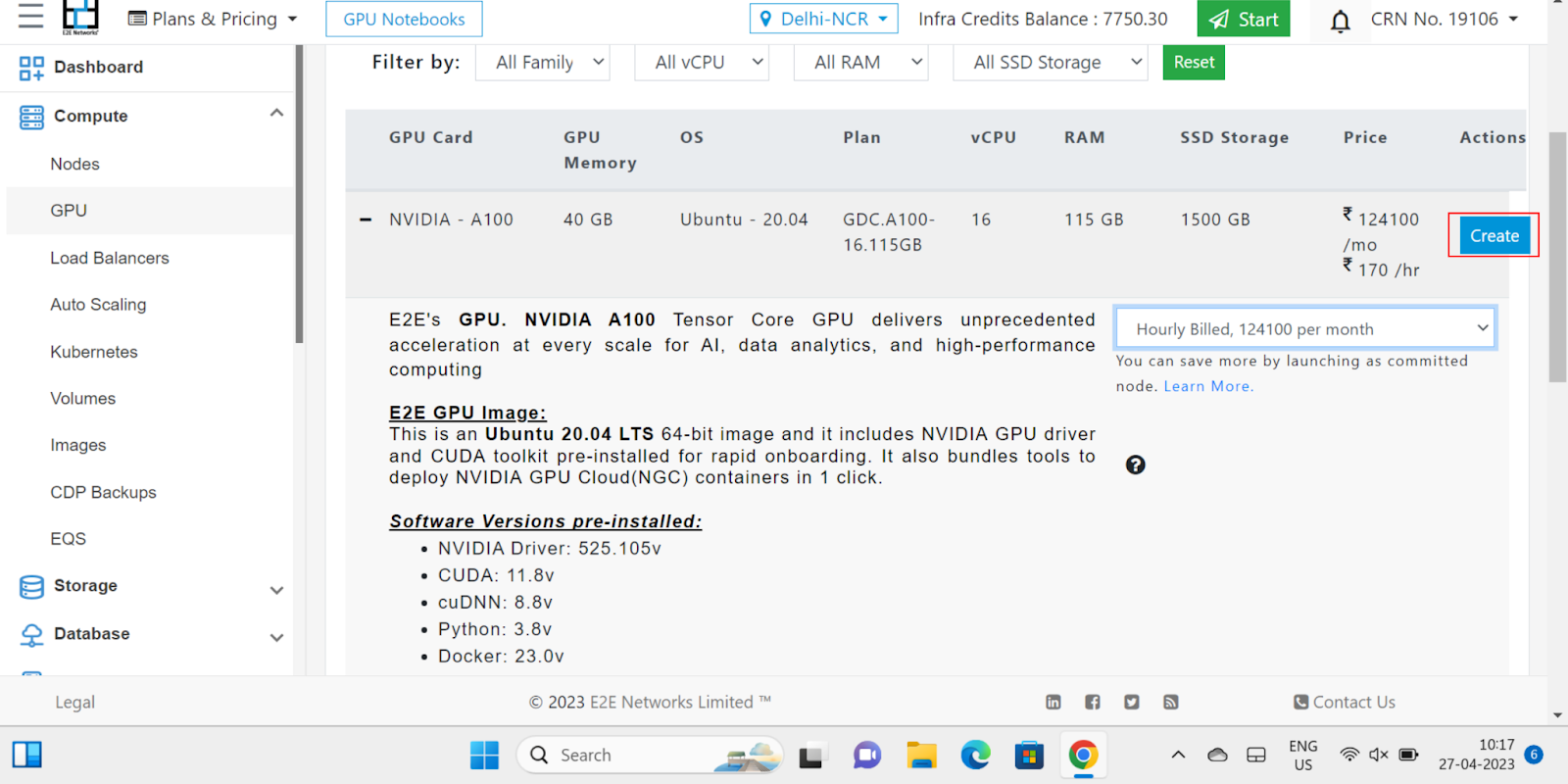
You can use your launched machine to deploy your model based on the open source AI Framework like PyTorch. To get further help, you can get in touch with our team at sales@e2enetworks.com.