What Are LLMs?
So, what are LLMs? LLMs (Large Language Models) are neural network models with a vast number of parameters trained on massive amounts of text data. These models have the ability to generate human-like text, translate languages, summarize articles, and answer questions with remarkable accuracy. Some of the popular LLMs include GPT-3, T5, and BERT. For instance, the GPT-3 has a whopping 175 billion parameters, and it has been shown to be capable of writing articles, essays, and even entire books!
LLMs have become increasingly popular in recent years due to their ability to generate human-like responses and perform complex language tasks. Building an LLM from scratch can be a challenging and time-consuming task, but with the right resources and techniques, it is possible to create a powerful language model that can be used for a wide range of applications. In this article, we will discuss how to get started in building, training, and launching a large language model.
The primary task of an LLM is language generation, which involves predicting the next word in a sentence or generating a new sentence based on a given prompt. However, LLMs can also perform a variety of other language-related tasks, such as language translation, summarization, sentiment analysis, and question answering.
The technical specifications of an LLM depend on the specific architecture and training data used. Some popular LLM architectures include the GPT (Generative Pre-trained Transformer) series, BERT (Bidirectional Encoder Representations from Transformers), and T5 (Text-to-Text Transfer Transformer). These models can contain billions of parameters and require large amounts of computational power to train and run.
How Does a Large Language Model Work?
A large language model, such as GPT-3, works by using a deep neural network to process and understand natural language text. It is trained on vast amounts of text data, such as books, articles, and websites, to learn patterns and relationships between words and phrases.
The model consists of multiple layers of artificial neurons, each of which processes a different aspect of the input text. The first layer of neurons takes in the raw text data, and subsequent layers build on the information learned by previous layers to extract higher-level features and meaning.
During training, the model is presented with input sequences of text and learns to predict the next word or sequence of words in the text based on the context. This process is known as language modeling, and the model's ability to accurately predict the next word in a sequence is used as a measure of its performance.
Once trained, the model can generate text by taking in an initial prompt or seed text and using its learned patterns and relationships to predict and generate the rest of the text. This process is known as language generation.
The large size of the model and the vast amount of training data it has been exposed to enable it to produce text that is often difficult to distinguish from that written by a human. This capability has many practical applications, such as natural language processing, chatbots, and language translation.
LLMs are changing the way we interact with machines and language. They can assist in customer service chatbots, auto-complete text in search engines, and even generate text for news articles and marketing content. The possibilities of LLMs are endless!
Moreover, LLMs have made significant contributions to various NLP tasks, including language modeling, machine translation, text summarization, and question-answering. In particular, LLMs have shown incredible results in language modeling, where they have outperformed traditional N-gram models, Hidden Markov Models, and even other Deep Learning models. For instance, the GPT-3 model has shown to achieve state-of-the-art results in several NLP benchmarks, including GLUE and SuperGLUE.
Furthermore, LLMs require massive computational resources to train and fine-tune, which is where cloud computing and GPUs come into play. The demand for cloud-based GPU computing has surged since the emergence of LLMs, and many cloud computing firms, including the one you're visiting, have capitalized on this opportunity by offering specialized hardware and software solutions for Deep Learning. Here are the detailed features of an LLM:
- Scale
One of the significant features of LLMs is their size. These models can have billions of parameters, which makes them much more accurate and efficient at processing natural language. For instance, the GPT-3 model released in 2020 has 175 billion parameters, making it one of the most significant LLMs to date. Training such a large model requires massive computational resources, including high-performance GPUs.
- Complexity
LLMs are complex models that are trained on vast amounts of text data. This training data is used to optimize the model's parameters to make it better at language-related tasks. The complexity of LLMs makes them better at handling natural language tasks such as machine translation, sentiment analysis, and question-answering systems.
- Fine-Tuning
Another critical feature of LLMs is fine-tuning, which involves training the model on a smaller dataset to make it more accurate for a specific task. Fine-tuning requires less computational power than training a large model from scratch, but still requires a powerful GPU to speed up the training process.
- Pre-Training
Pre-training is another feature of LLMs that involves training the model on a large corpus of text data before fine-tuning it on a specific task. Pre-training allows the model to learn the language's general patterns and structures, making it more efficient at handling various language-related tasks.
Getting Started: Building, Training, and Launching a Large Language Model
Building and deploying large language models have become an essential task for data scientists and AI practitioners. These models have revolutionized various fields, including Natural Language Processing, chatbots, and content generation. In this article, we will provide a detailed overview of the process of building, training, and launching a Large Language Model. We will cover the fundamental steps involved, along with key considerations and best practices. So, let's dive in!
Define Your Objectives and Scope
Before embarking on the journey of building an LLM, it's crucial to clearly define your objectives and scope. Determine the specific tasks you want the model to perform, such as text generation, sentiment analysis, or question-answering. Consider the domain, size, and complexity of the language model you need, as these factors will influence subsequent steps.
Data Collection and Preprocessing
Language models require substantial amounts of text data for training. Start by collecting a diverse and representative dataset relevant to your target domain. Ensure that the data is clean, properly annotated, and aligned with your objectives. Preprocess the data by removing noise, handling missing values, and performing tokenization, stemming, or lemmatization, depending on your requirements.
Model Architecture Selection
Choosing the right model architecture is crucial for achieving optimal performance. Popular choices include transformer-based architectures like GPT (Generative Pre-trained Transformer) and BERT (Bidirectional Encoder Representations from Transformers) . Consider factors such as model size, computational requirements, and available resources when making your selection.
Training the Language Model
Training a large language model is a computationally intensive task, often requiring specialized hardware like GPUs. Cloud providers like E2E Cloud offer flexible per-hour pricing for running GPU-based workloads. E2E Cloud supports NVIDIA GPUs, known for their performance and cost-effectiveness. With us, you can leverage the power of GPUs to accelerate the training process and reduce time-to-market.
Fine-tuning and Optimization
Once the initial training is complete, fine-tuning the model on specific downstream tasks can enhance its performance. Fine-tuning involves training the model on task-specific datasets while keeping the pre-trained weights intact. Experiment with different hyperparameters, learning rates, and optimization techniques to improve the model's accuracy and efficiency.
Deployment and Serving
After training and fine-tuning, it's time to deploy the language model and make it available for inference. Consider the deployment options that best suit your requirements, such as serving the model via APIs, integrating it into existing applications, or deploying it as a standalone service. Ensure that the deployment environment is scalable, secure, and able to handle the expected workload.
Monitoring and Iteration
Once your language model is live, continuous monitoring is essential to identify performance issues, detect biases, and gather feedback from users. Monitor metrics such as response time, accuracy, and user satisfaction to ensure that the model is delivering the desired results. Iterate and refine the model based on insights gained from monitoring to improving its performance over time.
Installing Open Source Frameworks like PyTorch to Build a Language Model and Leveraging the Power of NVIDIA GPUs on E2E Cloud
Using E2E Cloud Myaccount portal
- First login to the myaccount portal of E2E Networks with your respective credentials.
- Now, navigate to the GPU Wizard from your dashboard.
- Under the “Compute” menu on the extreme left, click on “GPU”
- Then click on “GPU Cloud Wizard”

- For NGC Container Pytorch click on “Next” under the “Actions” column.
- Choose the card according to requirement – A100 is recommended.

Now, choose your plan amongst the given options.
- Optionally you can add SSH key (recommended) or subscribe to CDP backup.
- Click on “Create My Node”.
- Wait for a few minutes and and confirm that node is in running state.

- Now, open terminal on your local PC and type the following command
ssh -NL localhost:1234:localhost:8888 root@<your_node_ip>
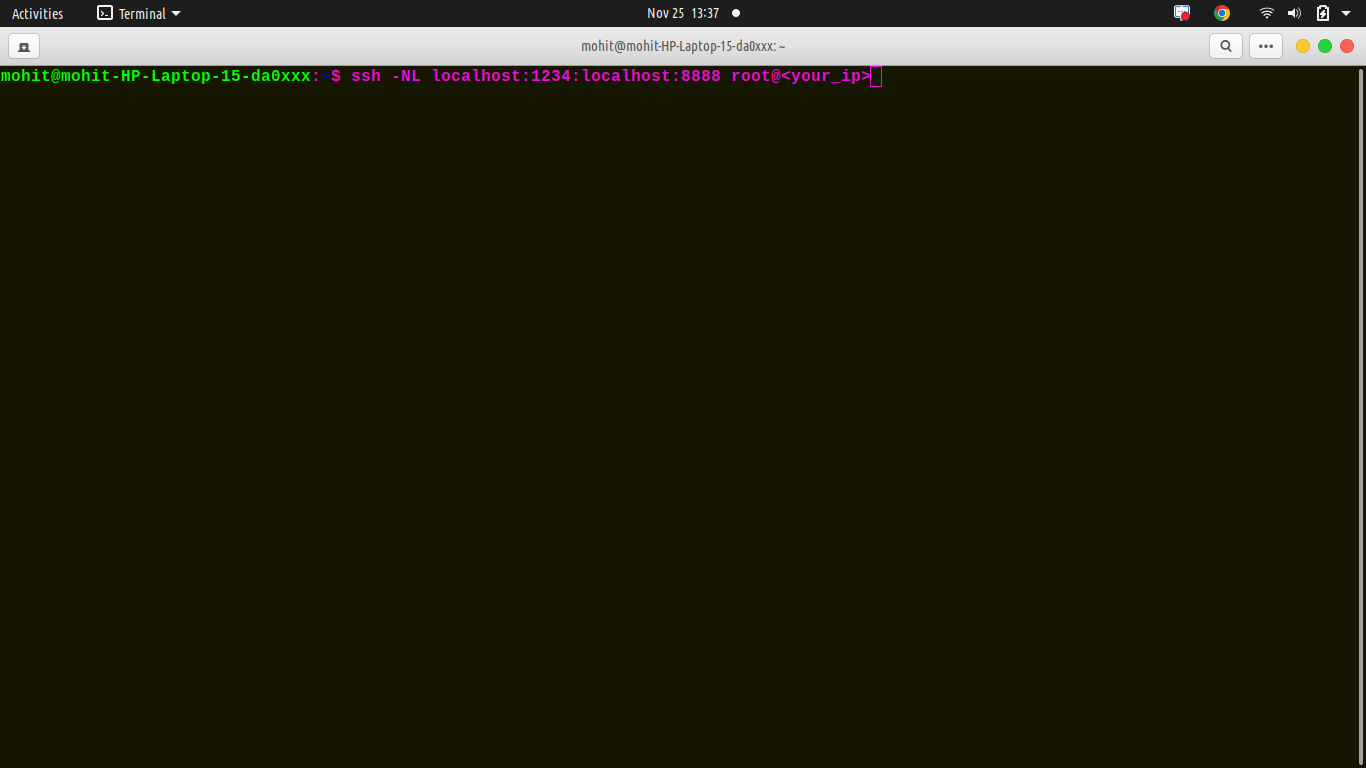
- The command usually will not show any output, which means the command has run without any error.
- Go to a web browser on your local PC and hit the url:http://localhost:1234/

- Congratulations! Now you can run your python code inside this jupyter notebook, which has Pytorch and all the libraries frequently used in Machine Learning preconfigured.
- To get the most out of GPU acceleration, use RAPIDS and DALI which are already installed inside this container.
- RAPIDS and DALI accelerate the tasks in ML apart from the learning also, like data loading and preprocessing.
LLMs are one of the most exciting and revolutionary developments in the field of AI, with the potential to transform the way we interact with machines and language. These models have shown to achieve state-of-the-art results in various NLP tasks, including language modeling, machine translation, and text summarization.
Additionally, they require massive computational resources to train, which has created a significant demand for cloud-based GPU computing. With the rapid advancements in LLMs and cloud computing, the possibilities for innovation in NLP and AI are limitless!
Sign Up for an E2E Cloud Account
Start your journey of building, training, and launching a Large Language Model by signing up for an E2E Cloud account and taking advantage of our competitive pricing and industry-vetted performance.
Here’s how can you sign up on E2E: https://myaccount.e2enetworks.com/